Principal relation component reasoning-enhanced social relation recognition
Appl. Intell.(2023)
摘要
Social relationships (SRs) are the basis of human life. Hence, the ability to accurately recognize interpersonal relations in public spaces based on visual observations helps policymakers improve mental health programs and address social challenges. The key to image-based computer-vision research on SR recognition (SRR) is a deep-learning mechanism that can predict SRs based on the contents of visual scenery images. Current methods explore logical constraints using relatively simple scenes with small groups of people. However, this is insufficient when desiring to form relation graphs of multiple groups simultaneously from complex scenes. Generally, complex scenes contain a principal relationship that applies to the largest proportion of people, and secondary relationships apply to smaller proportions. To effectively explore relational situations in complex scenes, we propose a new distributed reasoning strategy that accounts for principal and secondary SRs. First, our novel model enhances principal relation component reasoning, and a new contrastive learning algorithm supplements the principal relationship with secondary types. A shifted-window transformer is applied to extract interactive human relation features and local-global features to support more accurate and comprehensive relation prediction. Extensive experiments demonstrate that each part of the proposed model improves the accuracy of SRR and that the whole model outperforms state-of-the-art methods on public datasets. Graphical abstract
更多查看译文
关键词
Social relation recognition,Principal relation component reasoning,Swin transformer,Contrastive learning
AI 理解论文
溯源树
样例
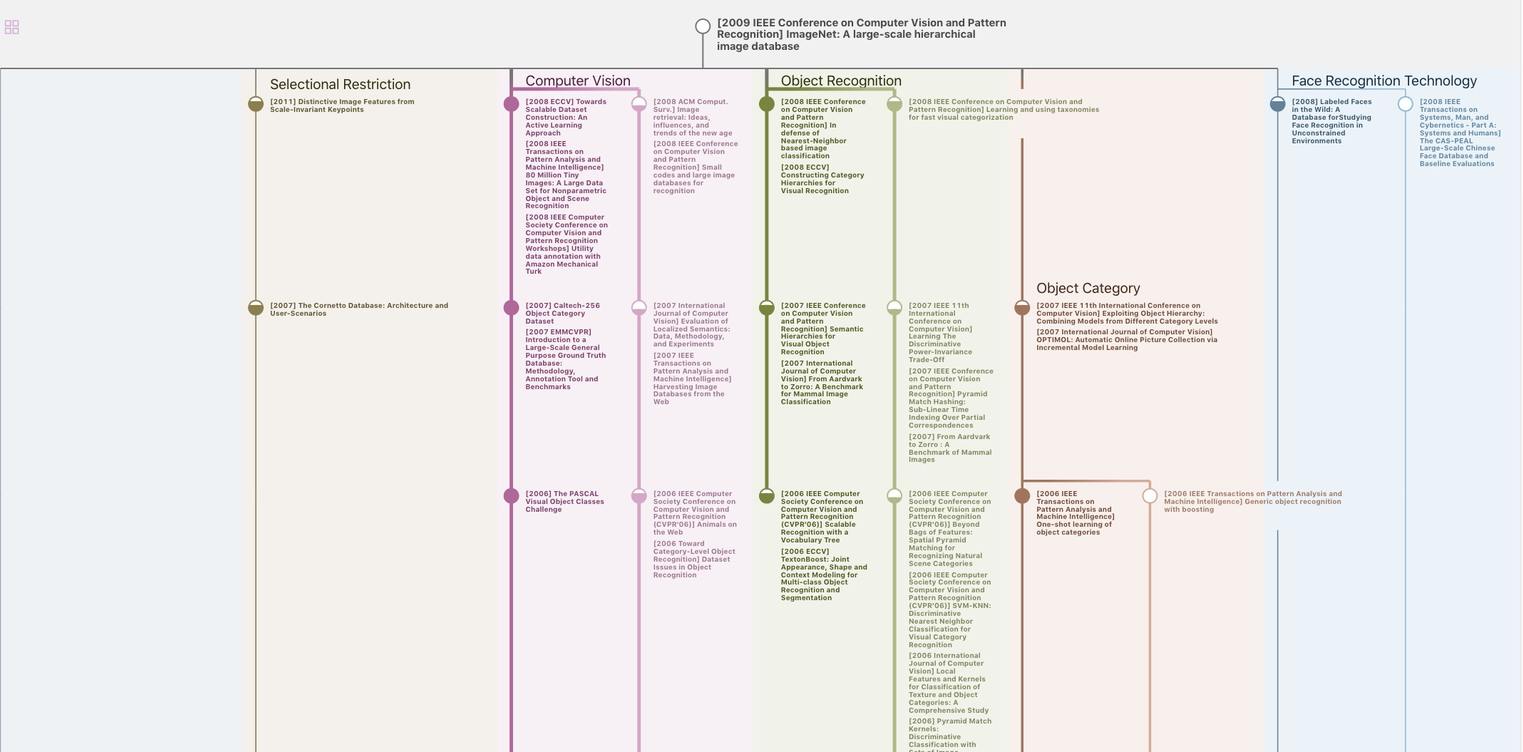
生成溯源树,研究论文发展脉络
Chat Paper
正在生成论文摘要