A transferability-aware covariance alignment network for image steganalysis
MULTIMEDIA TOOLS AND APPLICATIONS(2023)
摘要
Image steganalysis seeks to detect whether the secret information is hidden in images. Recently, to alleviate the distribution discrepancy between the training and test data, domain adaptation-based image steganalysis approaches have attracted much attention. However, existing methods ignore the evaluation of the transferability between datasets and inevitably lead to negative transfer. In this paper, we propose a Transferability-Aware Covariance Alignment Network (TA-CAN) for image steganalysis. This new solution consists of two key strategies: the transferable-aware module (TAM) and the covariance alignment loss (CAL). In TAM, we introduce a texture estimator and design a match query strategy based on texture pools, determining whether data sets can be transferred from one to another. Furthermore, to reduce the discrepancies between datasets with transferability, we leverage CAL to align second-order statistics in different domains. Extensive experiments demonstrate that our proposed algorithm can effectively handle distributional differences between training and test sets.
更多查看译文
关键词
Image steganalysis,Domain adaptation,Transferability,Covariance alignment,Texture complexity
AI 理解论文
溯源树
样例
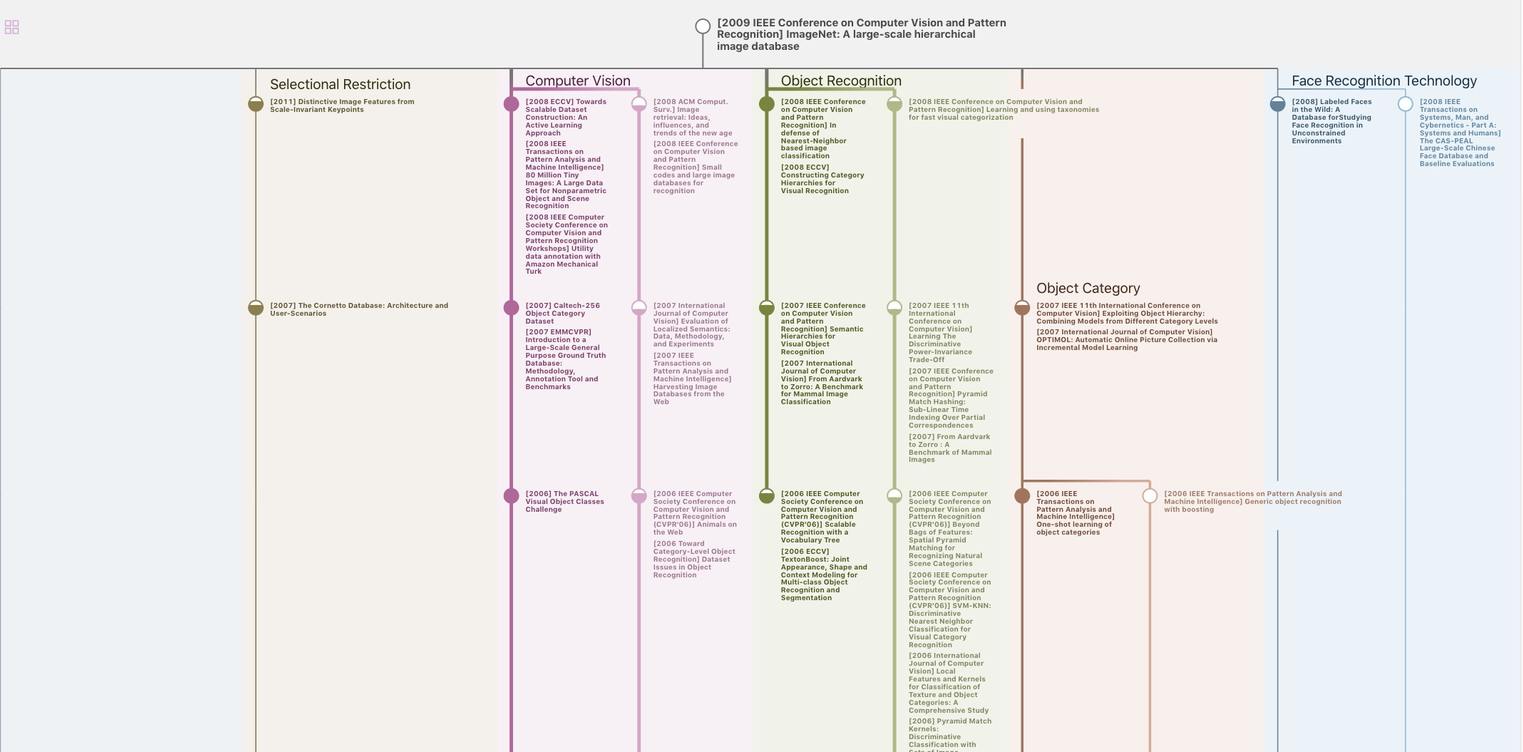
生成溯源树,研究论文发展脉络
Chat Paper
正在生成论文摘要