Multivariate long-time series traffic passenger flow prediction using causal convolutional sparse self-attention MTS-Informer
Neural Computing and Applications(2023)
摘要
As an important part of the operation preparation process of the intelligent transportation system, the passenger flow distribution law and forecast can guide the urban rail transit to formulate a reasonable operation scheduling plan. Due to the complexity, multi-variables, and instability of traffic passenger flow data, accurate passenger flow prediction takes a lot of work. Based on a convolutional neural network, a causal convolution self-attention traffic passenger flow prediction model MTS-Informer framework is proposed. This method follows the changing law of auxiliary variables, adopts the stabilization method to reduce the instability of the original sequence, and uses the causal convolution feature to improve the ability of the model’s self-attention mechanism to extract local information from the input sequence. The weakening effect of the self-attention mechanism ensures that it can learn similarly to the differential features in the original sequence data. In addition, the stationarity detection of the original sequence data is added. The experimental results show that the fitting degree of the sample data is significantly improved, and the standard error decreases between 10 and 40%, which verifies the effectiveness of the proposed modeling technique. It has higher prediction accuracy and operating efficiency and can provide a basis for urban traffic passenger flow prediction.
更多查看译文
关键词
Informer,Stationarity detection,Causal convolutional networks,Traffic passenger flow prediction
AI 理解论文
溯源树
样例
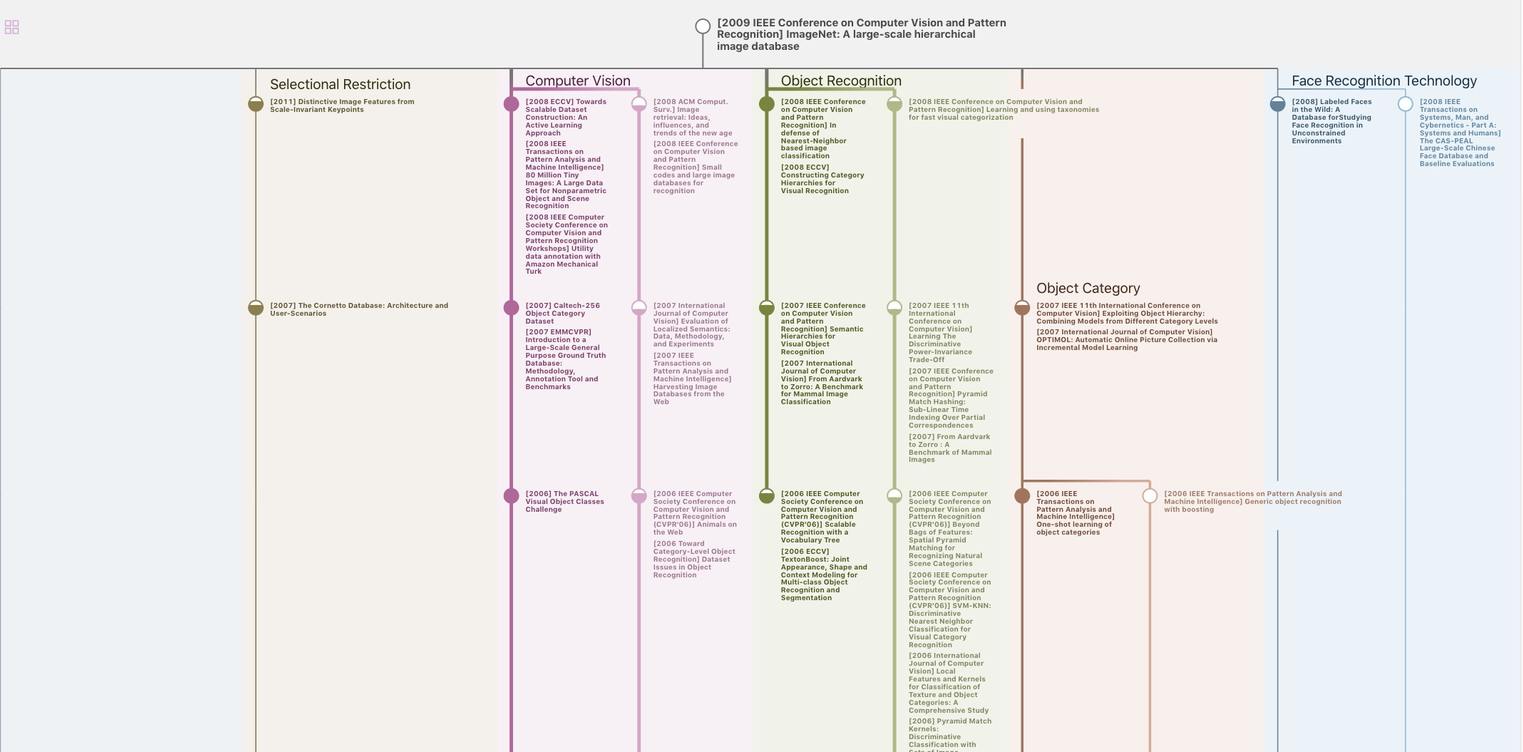
生成溯源树,研究论文发展脉络
Chat Paper
正在生成论文摘要