Co-clustering contaminated data: a robust model-based approach
Advances in Data Analysis and Classification(2023)
Abstract
The exploration and analysis of large high-dimensional data sets calls for well-thought techniques to extract the salient information from the data, such as co-clustering. Latent block models cast co-clustering in a probabilistic framework that extends finite mixture models to the two-way setting. Real-world data sets often contain anomalies which could be of interest per se and may make the results provided by standard, non-robust procedures unreliable. Also estimation of latent block models can be heavily affected by contaminated data. We propose an algorithm to compute robust estimates for latent block models. Experiments on both simulated and real data show that our method is able to resist high levels of contamination and can provide additional insight into the data by highlighting possible anomalies.
MoreTranslated text
Key words
Co-clustering,Robustness,Trimming,LBM,CEM algorithm
AI Read Science
Must-Reading Tree
Example
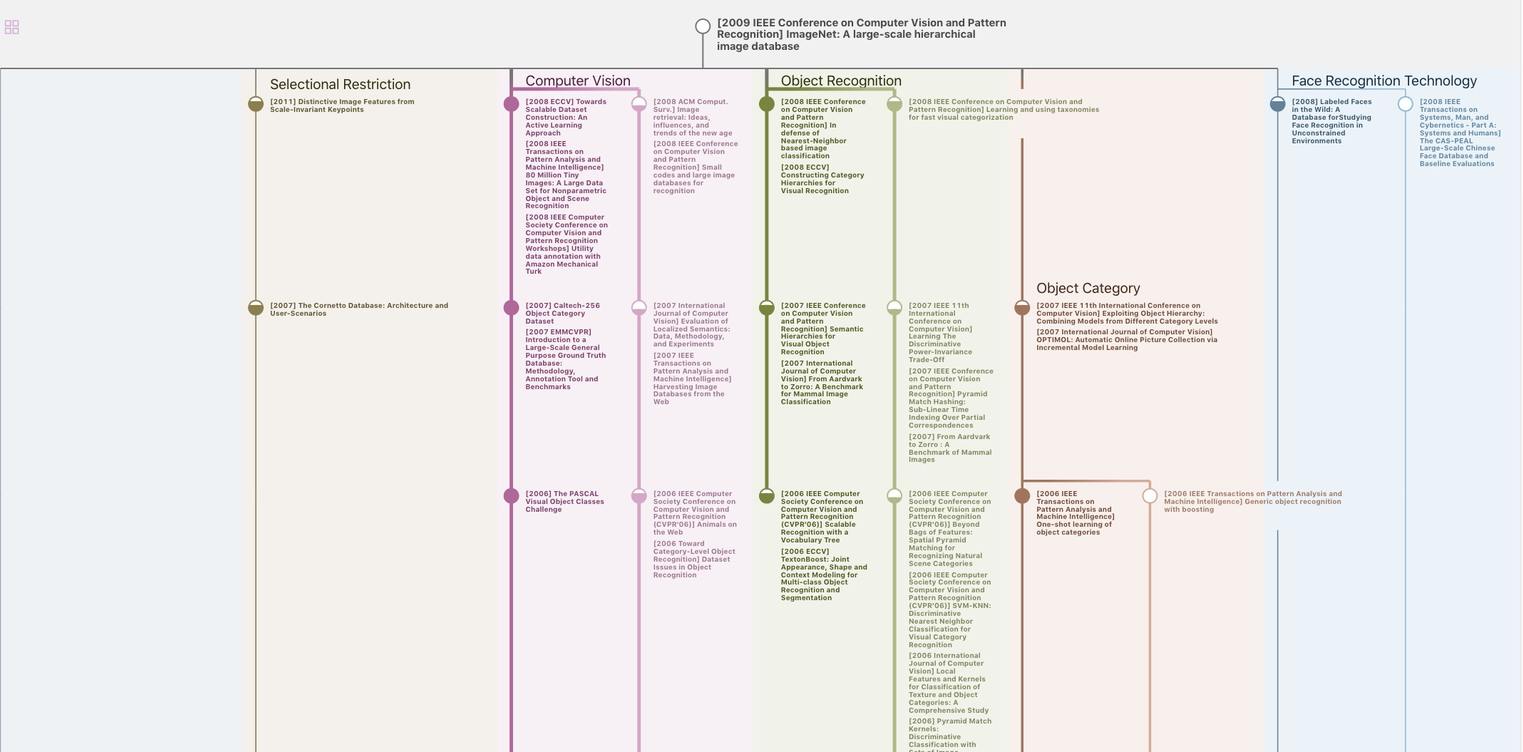
Generate MRT to find the research sequence of this paper
Chat Paper
Summary is being generated by the instructions you defined