VIC-KD: Variance-Invariance-Covariance Knowledge Distillation to Make Keyword Spotting More Robust Against Adversarial Attacks
arXiv (Cornell University)(2023)
摘要
Keyword spotting (KWS) refers to the task of identifying a set of predefined words in audio streams. With the advances seen recently with deep neural networks, it has become a popular technology to activate and control small devices, such as voice assistants. Relying on such models for edge devices, however, can be challenging due to hardware constraints. Moreover, as adversarial attacks have increased against voice-based technologies, developing solutions robust to such attacks has become crucial. In this work, we propose VIC-KD, a robust distillation recipe for model compression and adversarial robustness. Using self-supervised speech representations, we show that imposing geometric priors to the latent representations of both Teacher and Student models leads to more robust target models. Experiments on the Google Speech Commands datasets show that the proposed methodology improves upon current state-of-the-art robust distillation methods, such as ARD and RSLAD, by 12% and 8% in robust accuracy, respectively.
更多查看译文
关键词
more robust,variance-invariance-covariance
AI 理解论文
溯源树
样例
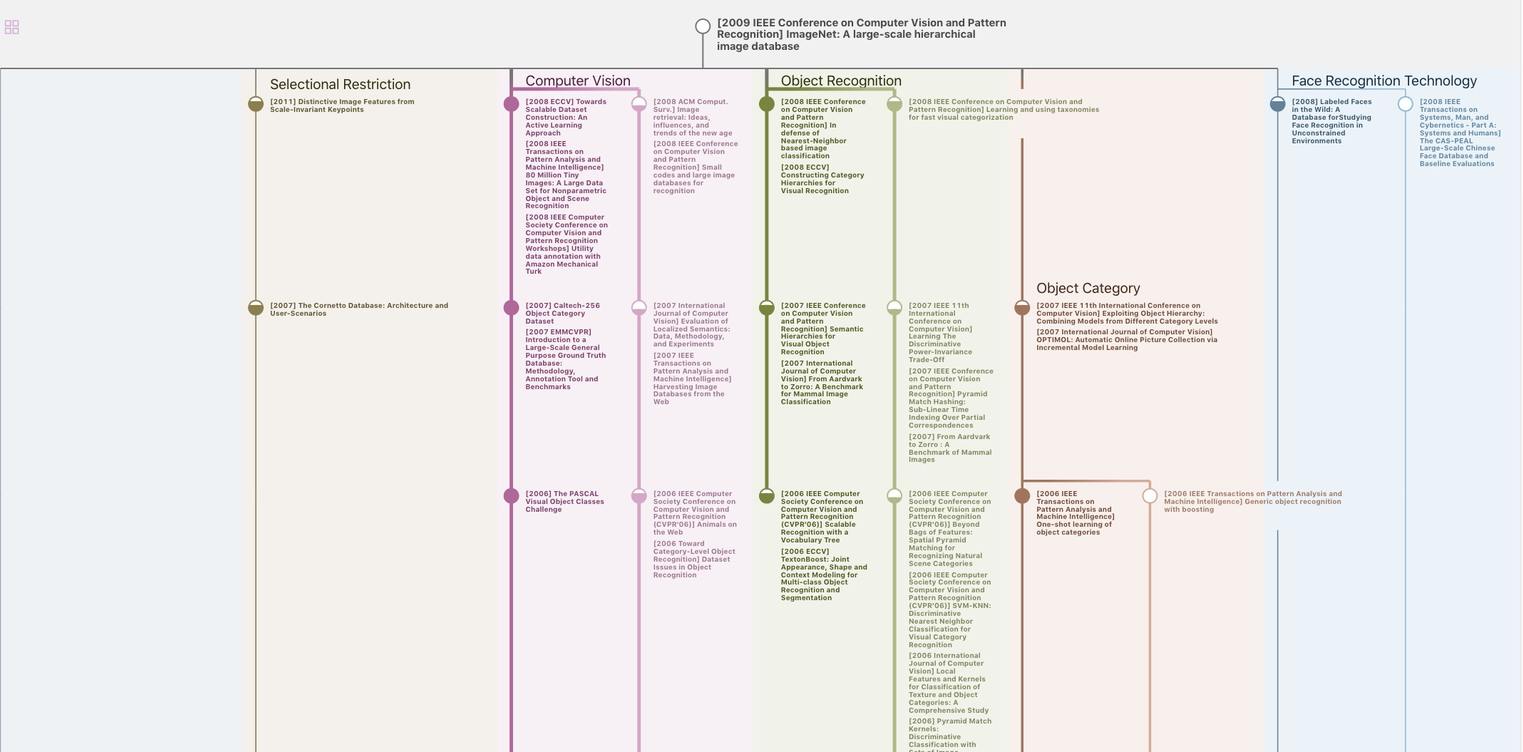
生成溯源树,研究论文发展脉络
Chat Paper
正在生成论文摘要