PI-RADS v2 Compliant Automated Segmentation of Prostate Zones Using co-training Motivated Multi-task Dual-Path CNN
CoRR(2023)
摘要
The detailed images produced by Magnetic Resonance Imaging (MRI) provide life-critical information for the diagnosis and treatment of prostate cancer. To provide standardized acquisition, interpretation and usage of the complex MRI images, the PI-RADS v2 guideline was proposed. An automated segmentation following the guideline facilitates consistent and precise lesion detection, staging and treatment. The guideline recommends a division of the prostate into four zones, PZ (peripheral zone), TZ (transition zone), DPU (distal prostatic urethra) and AFS (anterior fibromuscular stroma). Not every zone shares a boundary with the others and is present in every slice. Further, the representations captured by a single model might not suffice for all zones. This motivated us to design a dual-branch convolutional neural network (CNN), where each branch captures the representations of the connected zones separately. Further, the representations from different branches act complementary to each other at the second stage of training, where they are fine-tuned through an unsupervised loss. The loss penalises the difference in predictions from the two branches for the same class. We also incorporate multi-task learning in our framework to further improve the segmentation accuracy. The proposed approach improves the segmentation accuracy of the baseline (mean absolute symmetric distance) by 7.56%, 11.00%, 58.43% and 19.67% for PZ, TZ, DPU and AFS zones respectively.
更多查看译文
关键词
prostate zones,pi-rads,co-training,multi-task,dual-path
AI 理解论文
溯源树
样例
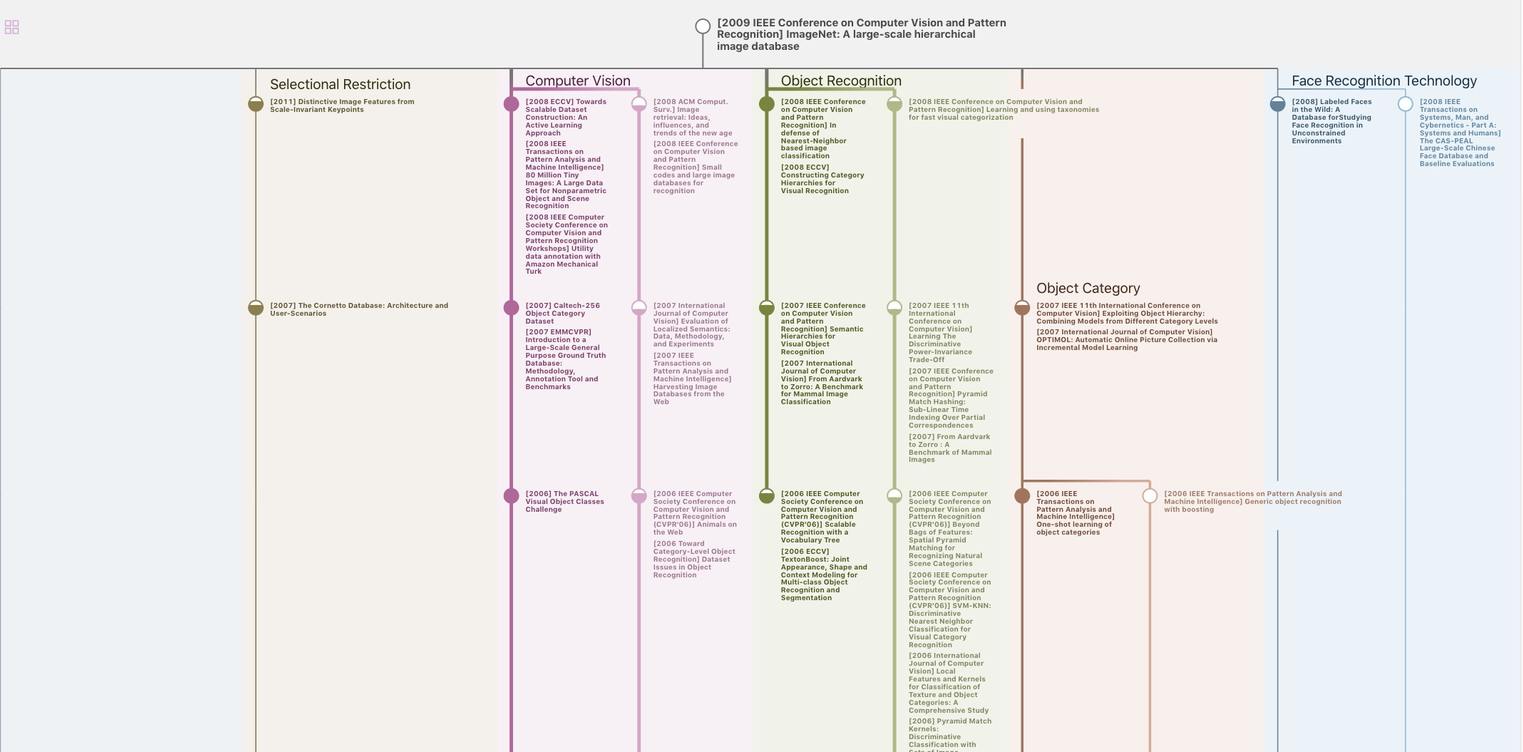
生成溯源树,研究论文发展脉络
Chat Paper
正在生成论文摘要