Direct Short-Term Net Load Forecasting Based on Machine Learning Principles for Solar-Integrated Microgrids
IEEE Access(2023)
摘要
Accurate net load forecasting is a cost-effective technique, crucial for the planning, stability, reliability, and integration of variable solar photovoltaic (PV) systems in modern power systems. This work presents a direct short-term net load forecasting (STNLF) methodology for solar-integrated microgrids by leveraging machine learning (ML) principles. The proposed data-driven method comprises of an initial input feature engineering and filtering step, construction of forecasting model using Bayesian neural networks, and an optimization stage. The performance of the proposed model was validated on historical net load data obtained from a university campus solar-powered microgrid. The results demonstrated the effectiveness of the model for providing accurate and robust STNLF. Specifically, the optimally constructed model yielded a normalized root mean square error of 3.98% when benchmarked using a 1-year historical microgrid data. The $k$ -fold cross-validation method was then used and proved the stability of the forecasting model. Finally, the obtained ML-based forecasts demonstrated improvements of 17.77% when compared against forecasts of a baseline naïve persistence model. To this end, this work provides insights on how to construct high-performance STNLF models for solar-integrated microgrids. Such insights on the development of accurate STNLF architectures can have positive implications in actual microgrid decision-making by utilities/operators.
更多查看译文
关键词
machine learning principles,machine learning,short-term,solar-integrated
AI 理解论文
溯源树
样例
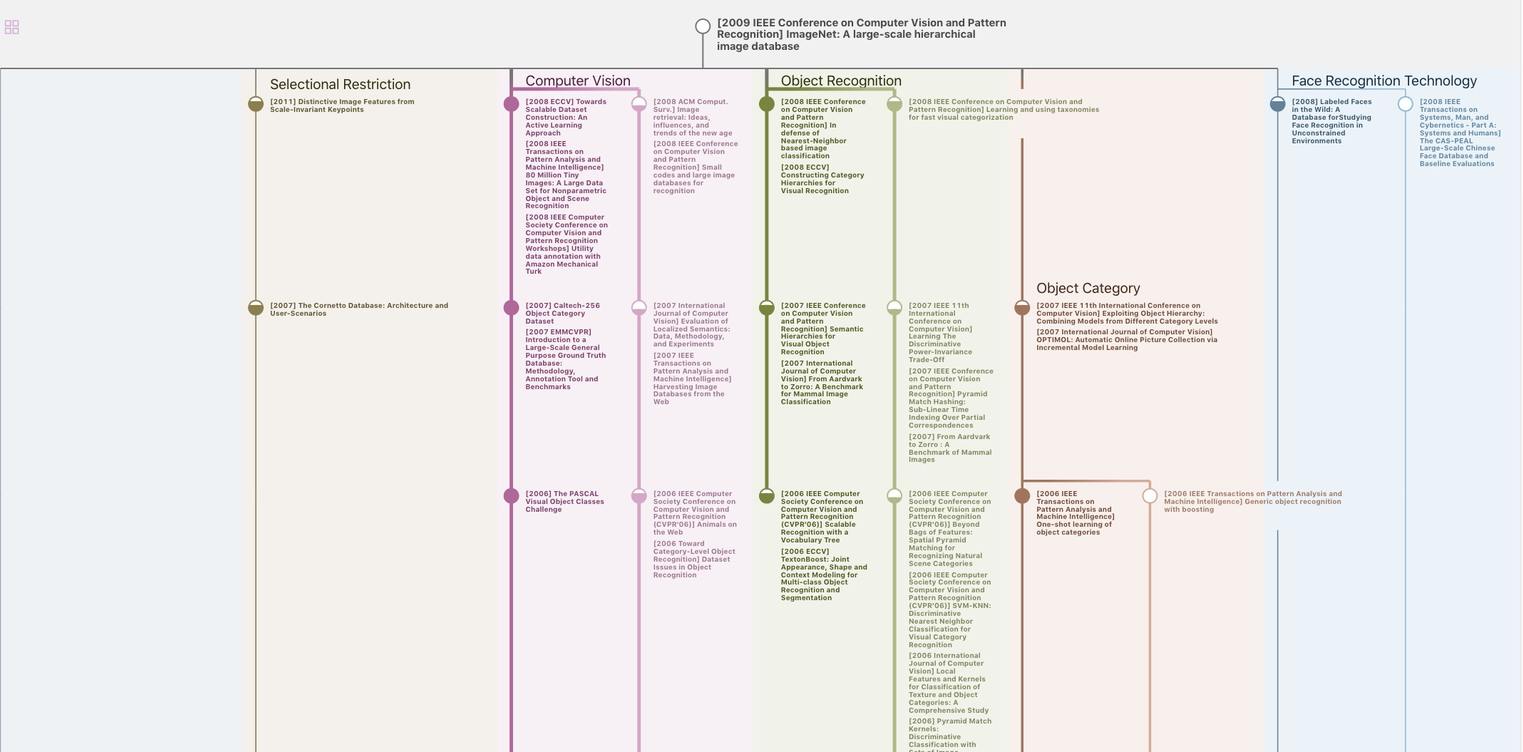
生成溯源树,研究论文发展脉络
Chat Paper
正在生成论文摘要