UNICON: A unified framework for behavior-based consumer segmentation in e-commerce
CoRR(2023)
摘要
Data-driven personalization is a key practice in fashion e-commerce, improving the way businesses serve their consumers needs with more relevant content. While hyper-personalization offers highly targeted experiences to each consumer, it requires a significant amount of private data to create an individualized journey. To alleviate this, group-based personalization provides a moderate level of personalization built on broader common preferences of a consumer segment, while still being able to personalize the results. We introduce UNICON, a unified deep learning consumer segmentation framework that leverages rich consumer behavior data to learn long-term latent representations and utilizes them to extract two pivotal types of segmentation catering various personalization use-cases: lookalike, expanding a predefined target seed segment with consumers of similar behavior, and data-driven, revealing non-obvious consumer segments with similar affinities. We demonstrate through extensive experimentation our framework effectiveness in fashion to identify lookalike Designer audience and data-driven style segments. Furthermore, we present experiments that showcase how segment information can be incorporated in a hybrid recommender system combining hyper and group-based personalization to exploit the advantages of both alternatives and provide improvements on consumer experience.
更多查看译文
关键词
consumer segmentation,behavior-based,e-commerce
AI 理解论文
溯源树
样例
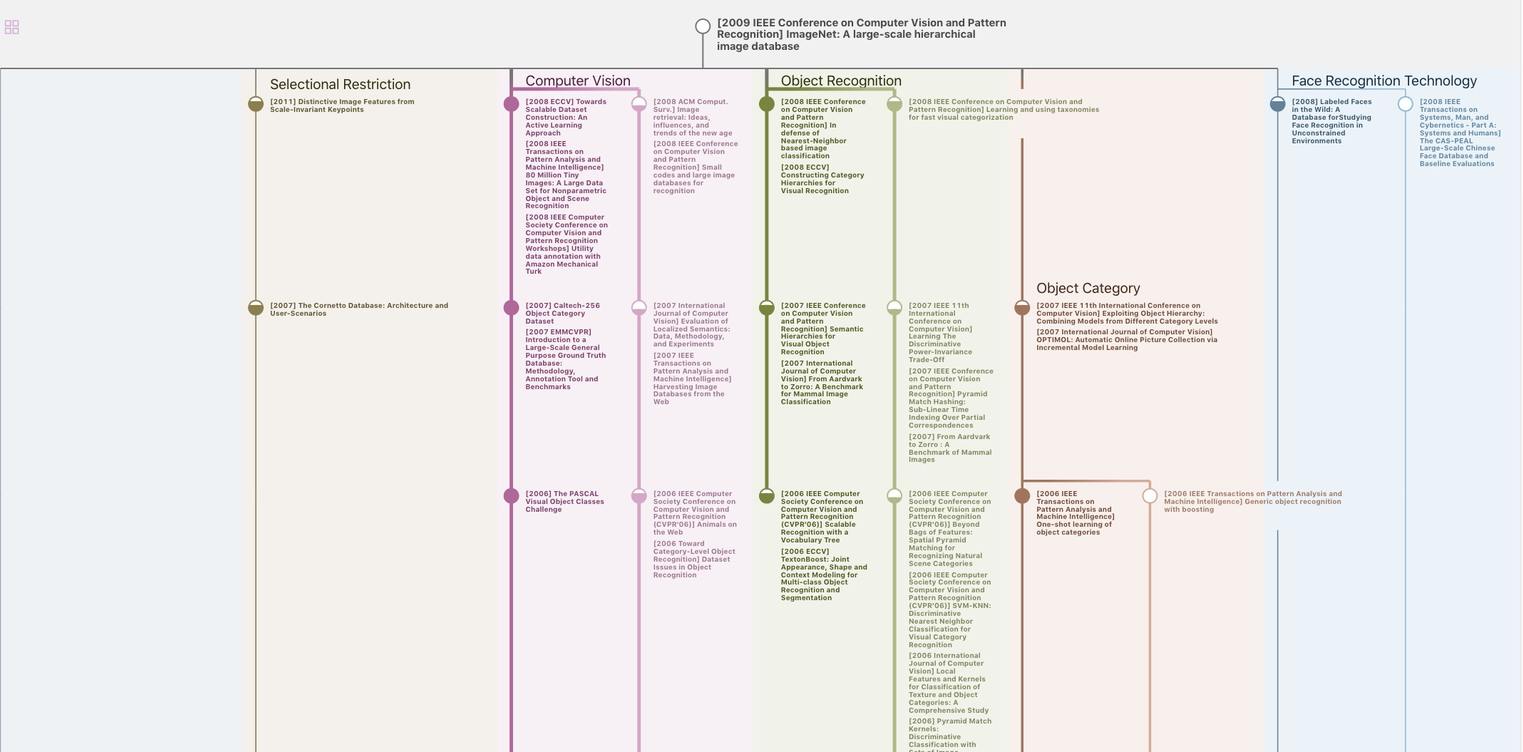
生成溯源树,研究论文发展脉络
Chat Paper
正在生成论文摘要