Prototype-Enhanced Hypergraph Learning for Heterogeneous Information Networks
CoRR(2023)
摘要
The variety and complexity of relations in multimedia data lead to Heterogeneous Information Networks (HINs). Capturing the semantics from such networks requires approaches capable of utilizing the full richness of the HINs. Existing methods for modeling HINs employ techniques originally designed for graph neural networks, and HINs decomposition analysis, like using manually predefined metapaths. In this paper, we introduce a novel prototype-enhanced hypergraph learning approach for node classification in HINs. Using hypergraphs instead of graphs, our method captures higher-order relationships among nodes and extracts semantic information without relying on metapaths. Our method leverages the power of prototypes to improve the robustness of the hypergraph learning process and creates the potential to provide human-interpretable insights into the underlying network structure. Extensive experiments on three real-world HINs demonstrate the effectiveness of our method.
更多查看译文
关键词
heterogeneous information
AI 理解论文
溯源树
样例
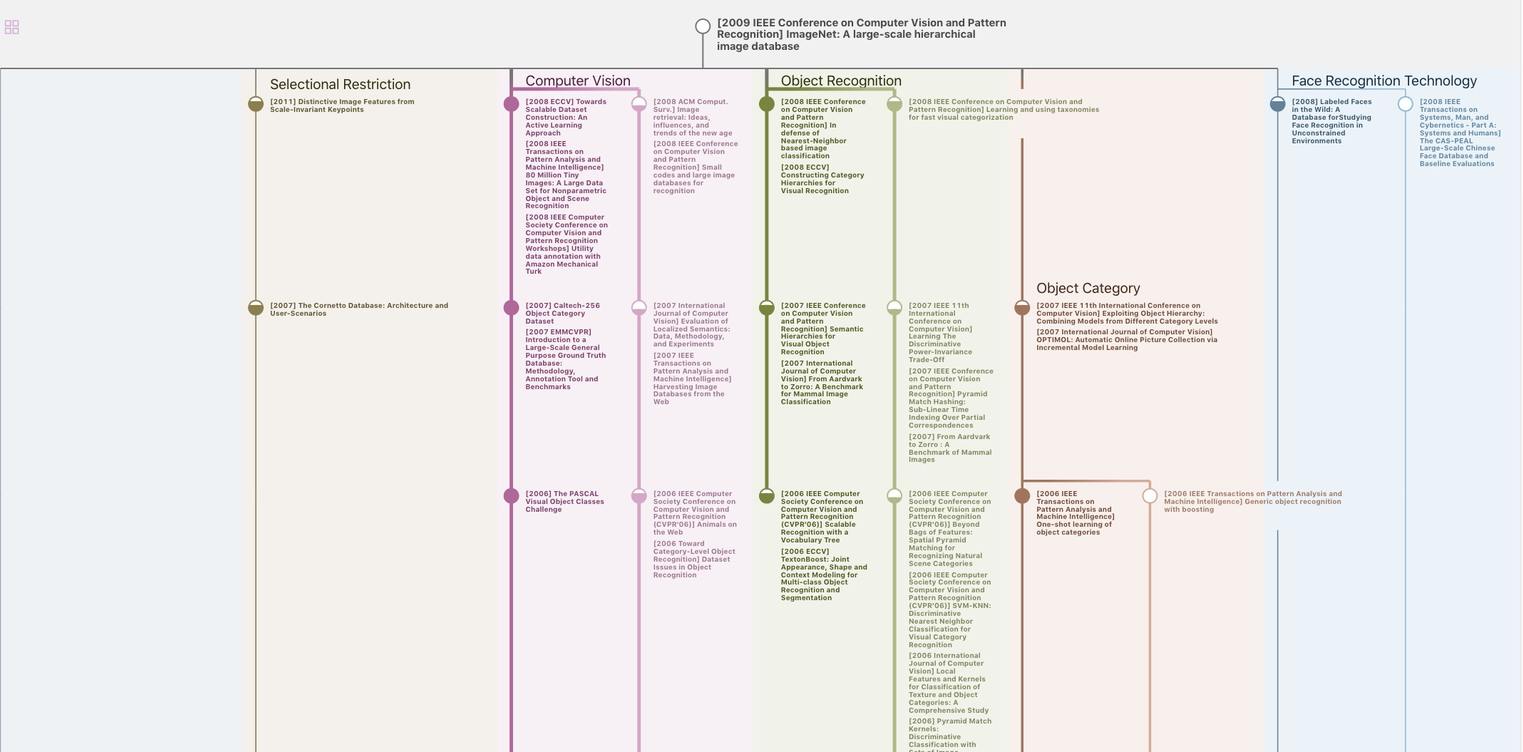
生成溯源树,研究论文发展脉络
Chat Paper
正在生成论文摘要