Importance of Smoothness Induced by Optimizers in FL4ASR: Towards Understanding Federated Learning for End-to-End ASR
CoRR(2023)
摘要
In this paper, we start by training End-to-End Automatic Speech Recognition (ASR) models using Federated Learning (FL) and examining the fundamental considerations that can be pivotal in minimizing the performance gap in terms of word error rate between models trained using FL versus their centralized counterpart. Specifically, we study the effect of (i) adaptive optimizers, (ii) loss characteristics via altering Connectionist Temporal Classification (CTC) weight, (iii) model initialization through seed start, (iv) carrying over modeling setup from experiences in centralized training to FL, e.g., pre-layer or post-layer normalization, and (v) FL-specific hyperparameters, such as number of local epochs, client sampling size, and learning rate scheduler, specifically for ASR under heterogeneous data distribution. We shed light on how some optimizers work better than others via inducing smoothness. We also summarize the applicability of algorithms, trends, and propose best practices from prior works in FL (in general) toward End-to-End ASR models.
更多查看译文
关键词
practical guide,federated learning,end-to-end,automatic speech recognition,CTC-AED
AI 理解论文
溯源树
样例
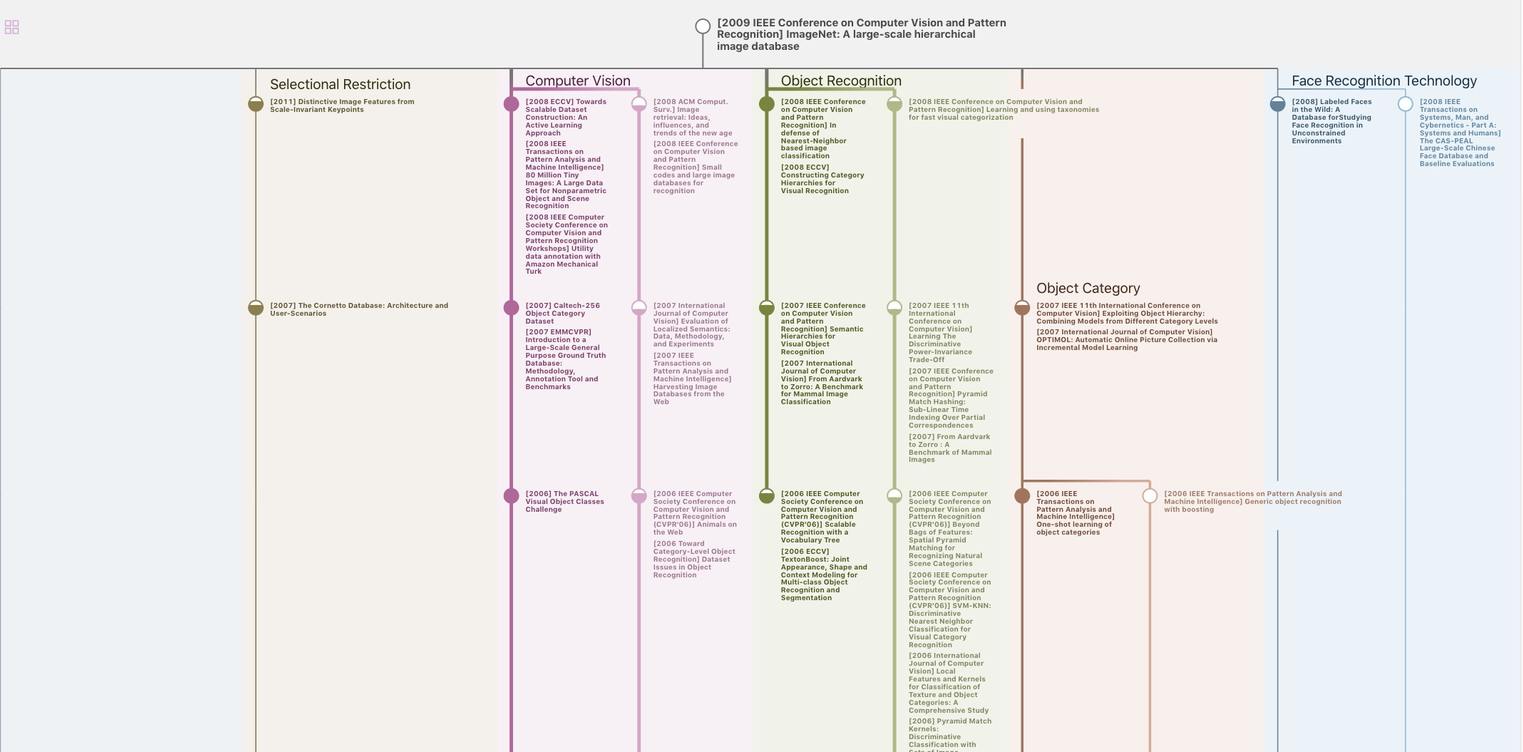
生成溯源树,研究论文发展脉络
Chat Paper
正在生成论文摘要