Sens-BERT: Enabling Transferability and Re-calibration of Calibration Models for Low-cost Sensors under Reference Measurements Scarcity
arXiv (Cornell University)(2023)
摘要
Low-cost sensors measurements are noisy, which limits large-scale
adaptability in airquality monitoirng. Calibration is generally used to get
good estimates of air quality measurements out from LCS. In order to do this,
LCS sensors are typically co-located with reference stations for some duration.
A calibration model is then developed to transfer the LCS sensor measurements
to the reference station measurements. Existing works implement the calibration
of LCS as an optimization problem in which a model is trained with the data
obtained from real-time deployments; later, the trained model is employed to
estimate the air quality measurements of that location. However, this approach
is sensor-specific and location-specific and needs frequent re-calibration. The
re-calibration also needs massive data like initial calibration, which is a
cumbersome process in practical scenarios.
To overcome these limitations, in this work, we propose Sens-BERT, a
BERT-inspired learning approach to calibrate LCS, and it achieves the
calibration in two phases: self-supervised pre-training and supervised
fine-tuning. In the pre-training phase, we train Sens-BERT with only LCS data
(without reference station observations) to learn the data distributional
features and produce corresponding embeddings. We then use the Sens-BERT
embeddings to learn a calibration model in the fine-tuning phase. Our proposed
approach has many advantages over the previous works. Since the Sens-BERT
learns the behaviour of the LCS, it can be transferable to any sensor of the
same sensing principle without explicitly training on that sensor. It requires
only LCS measurements in pre-training to learn the characters of LCS, thus
enabling calibration even with a tiny amount of paired data in fine-tuning. We
have exhaustively tested our approach with the Community Air Sensor Network
(CAIRSENSE) data set, an open repository for LCS.
更多查看译文
关键词
reference measurements scarcity,enabling transferability,models,sens-bert,re-calibration,low-cost
AI 理解论文
溯源树
样例
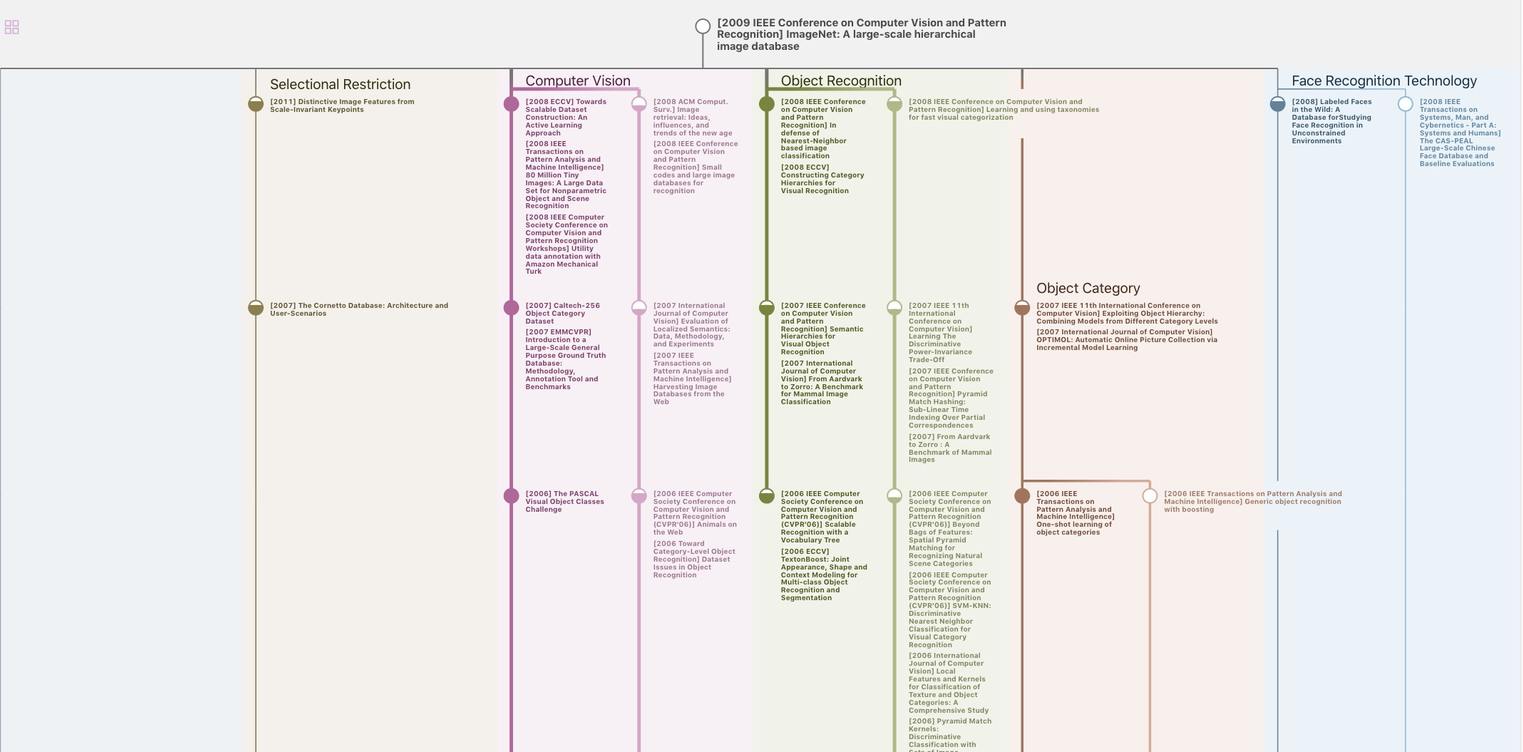
生成溯源树,研究论文发展脉络
Chat Paper
正在生成论文摘要