DROP: Dynamics Responses from Human Motion Prior and Projective Dynamics.
CoRR(2023)
摘要
Synthesizing realistic human movements, dynamically responsive to the environment, is a long-standing objective in character animation, with applications in computer vision, sports, and healthcare, for motion prediction and data augmentation. Recent kinematics-based generative motion models offer impressive scalability in modeling extensive motion data, albeit without an interface to reason about and interact with physics. While simulator-in-the-loop learning approaches enable highly physically realistic behaviors, the challenges in training often affect scalability and adoption. We introduce DROP, a novel framework for modeling Dynamics Responses of humans using generative mOtion prior and Projective dynamics. DROP can be viewed as a highly stable, minimalist physics-based human simulator that interfaces with a kinematics-based generative motion prior. Utilizing projective dynamics, DROP allows flexible and simple integration of the learned motion prior as one of the projective energies, seamlessly incorporating control provided by the motion prior with Newtonian dynamics. Serving as a model-agnostic plug-in, DROP enables us to fully leverage recent advances in generative motion models for physics-based motion synthesis. We conduct extensive evaluations of our model across different motion tasks and various physical perturbations, demonstrating the scalability and diversity of responses.
更多查看译文
关键词
human motion prior,dynamics responses,human motion
AI 理解论文
溯源树
样例
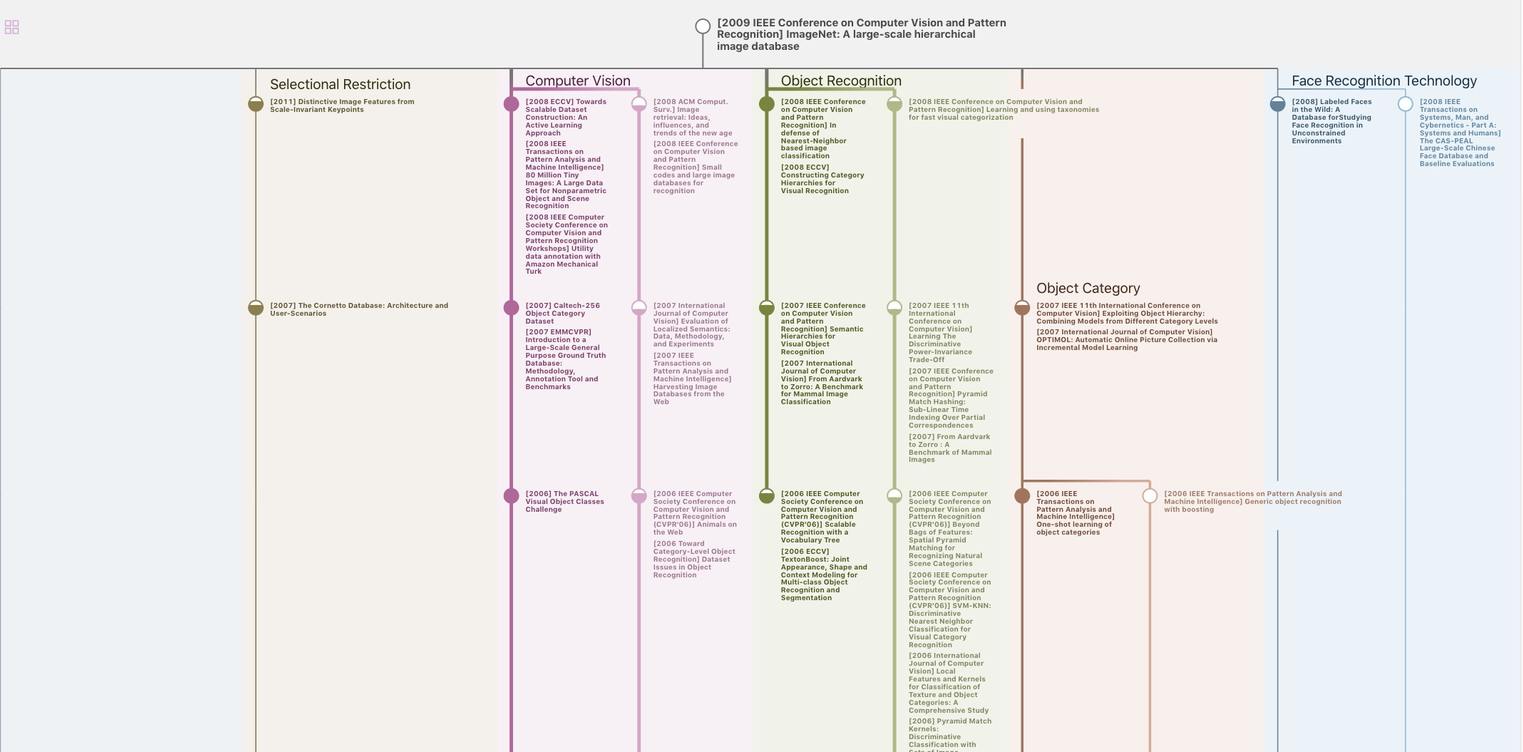
生成溯源树,研究论文发展脉络
Chat Paper
正在生成论文摘要