Can Class-Priors Help Single-Positive Multi-Label Learning?
CoRR(2023)
摘要
Single-positive multi-label learning (SPMLL) is a typical weakly supervised multi-label learning problem, where each training example is annotated with only one positive label. Existing SPMLL methods typically assign pseudo-labels to unannotated labels with the assumption that prior probabilities of all classes are identical. However, the class-prior of each category may differ significantly in real-world scenarios, which makes the predictive model not perform as well as expected due to the unrealistic assumption on real-world application. To alleviate this issue, a novel framework named {\proposed}, i.e., Class-pRiors Induced Single-Positive multi-label learning, is proposed. Specifically, a class-priors estimator is introduced, which could estimate the class-priors that are theoretically guaranteed to converge to the ground-truth class-priors. In addition, based on the estimated class-priors, an unbiased risk estimator for classification is derived, and the corresponding risk minimizer could be guaranteed to approximately converge to the optimal risk minimizer on fully supervised data. Experimental results on ten MLL benchmark datasets demonstrate the effectiveness and superiority of our method over existing SPMLL approaches.
更多查看译文
关键词
learning,class-priors,single-positive,multi-label
AI 理解论文
溯源树
样例
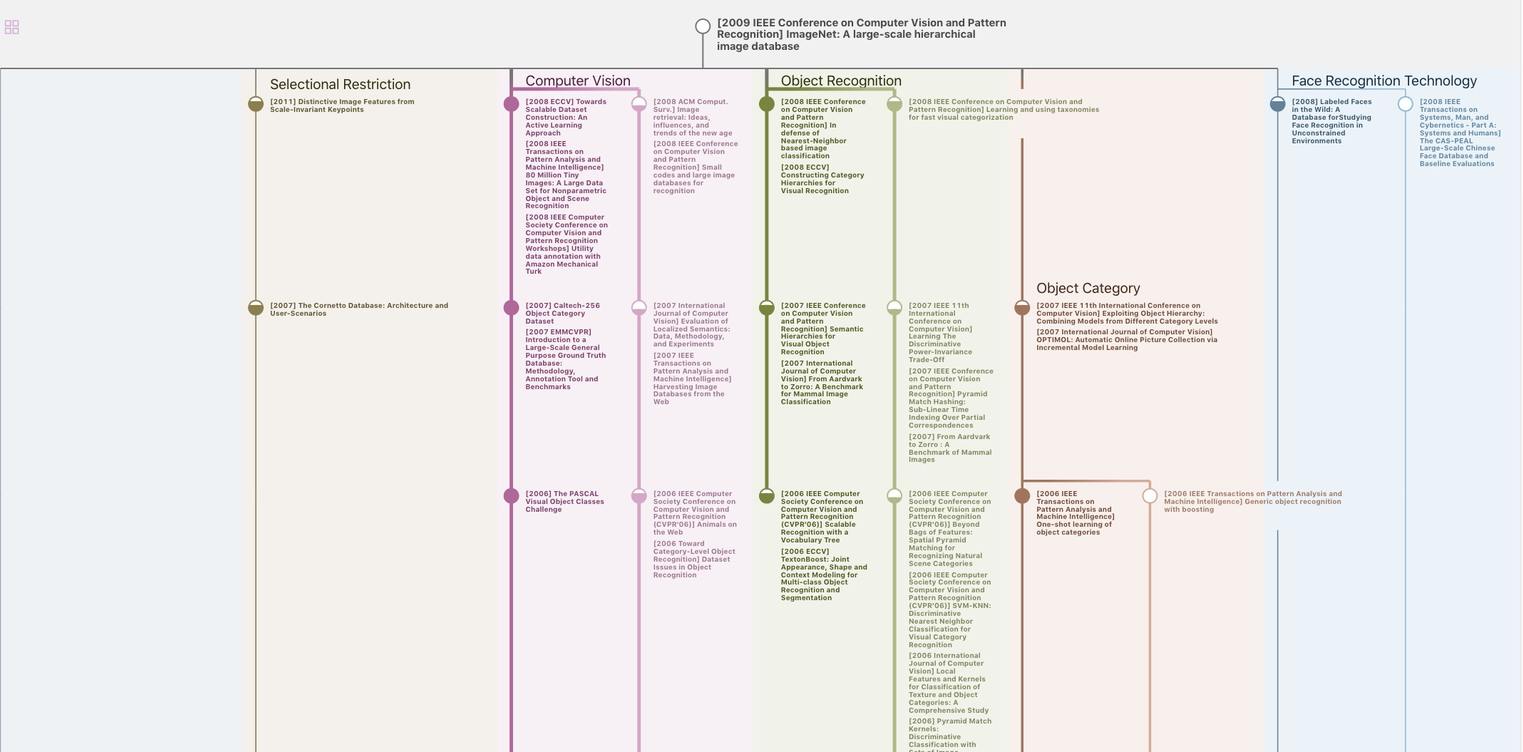
生成溯源树,研究论文发展脉络
Chat Paper
正在生成论文摘要