Audio classification with Dilated Convolution with Learnable Spacings
CoRR(2023)
摘要
Dilated convolution with learnable spacings (DCLS) is a recent convolution method in which the positions of the kernel elements are learned throughout training by backpropagation. Its interest has recently been demonstrated in computer vision (ImageNet classification and downstream tasks). Here we show that DCLS is also useful for audio tagging using the AudioSet classification benchmark. We took two state-of-the-art convolutional architectures using depthwise separable convolutions (DSC), ConvNeXt and ConvFormer, and a hybrid one using attention in addition, FastViT, and drop-in replaced all the DSC layers by DCLS ones. This significantly improved the mean average precision (mAP) with the three architectures without increasing the number of parameters and with only a low cost on the throughput. The method code is based on PyTorch and is available at https://github.com/K-H-Ismail/DCLS-Audio
更多查看译文
关键词
audio classification,dilated convolution,learnable spacings
AI 理解论文
溯源树
样例
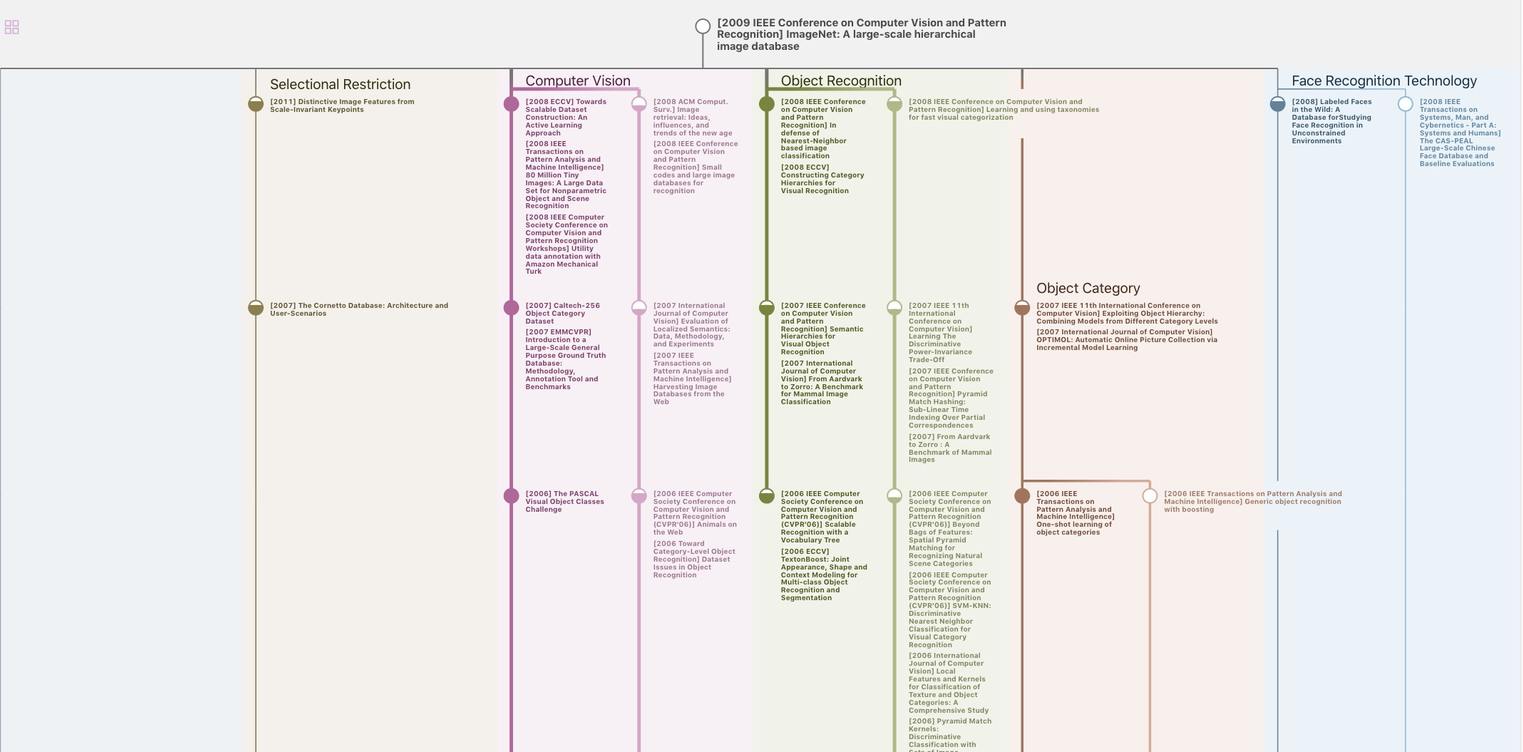
生成溯源树,研究论文发展脉络
Chat Paper
正在生成论文摘要