Convolutional autoencoder-based multimodal one-class classification
CoRR(2023)
摘要
One-class classification refers to approaches of learning using data from a single class only. In this paper, we propose a deep learning one-class classification method suitable for multimodal data, which relies on two convolutional autoencoders jointly trained to reconstruct the positive input data while obtaining the data representations in the latent space as compact as possible. During inference, the distance of the latent representation of an input to the origin can be used as an anomaly score. Experimental results using a multimodal macroinvertebrate image classification dataset show that the proposed multimodal method yields better results as compared to the unimodal approach. Furthermore, study the effect of different input image sizes, and we investigate how recently proposed feature diversity regularizers affect the performance of our approach. We show that such regularizers improve performance.
更多查看译文
关键词
classification,autoencoder-based,one-class
AI 理解论文
溯源树
样例
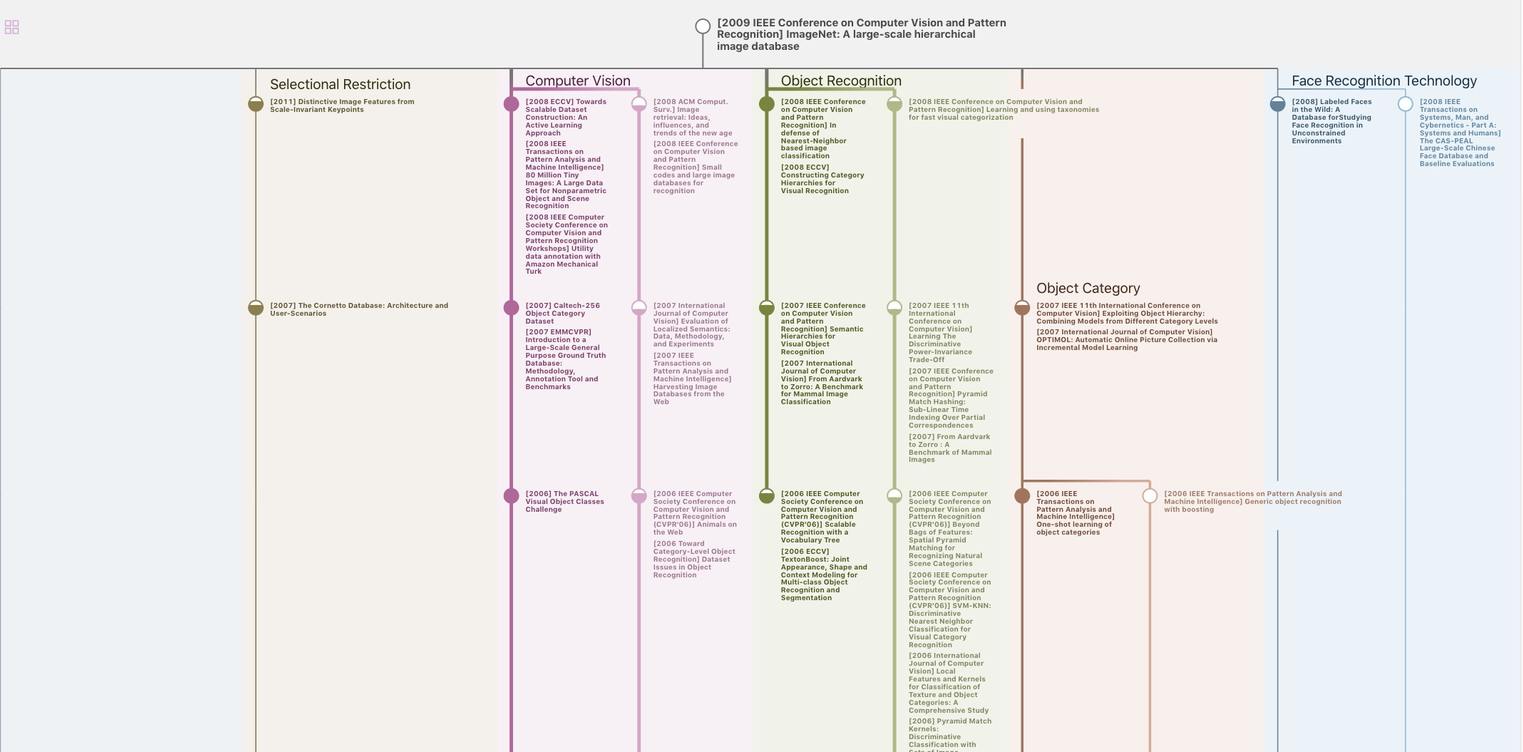
生成溯源树,研究论文发展脉络
Chat Paper
正在生成论文摘要