Communication-Efficient Federated Learning for Resource-Constrained Edge Devices
IEEE Transactions on Machine Learning in Communications and Networking(2023)
摘要
Federated learning (FL) is an emerging paradigm to train a global deep neural network (DNN) model by collaborative clients that store their private data locally through the coordination of a central server. A major challenge is a high communication overhead during the training stage, especially when the clients are edge devices that are linked wirelessly to the central server. In this paper, we propose efficient techniques to reduce the communication overhead of FL from three perspectives. First, to reduce the amount of data being exchanged between clients and the central server, we propose employing low-rank tensor models to represent neural networks to substantially reduce the model parameter size, leading to significant reductions in both computational complexity and communication overhead. Then, we consider two edge scenarios and propose the corresponding FL schemes over wireless channels. The first scenario is that the edge devices barely have sufficient computing and communication capabilities, and we propose a lattice-coded over-the-air computation scheme for the clients to transmit their local model parameters to the server. Compared with the traditional repetition transmission, this scheme significantly reduces the distortion. The second scenario is that the edge devices have very limited computing and communication power, and we propose natural gradient-based FL, that involves forward pass only, and each client transmits only one scalar to the server at each training iteration. Numerical results on the MNIST data set and the CIFAR-10 data set are provided to demonstrate the effectiveness of the proposed communication-efficient FL techniques, in that they significantly reduce the communication overhead while maintaining high learning performance.
更多查看译文
关键词
edge,learning,devices,communication-efficient,resource-constrained
AI 理解论文
溯源树
样例
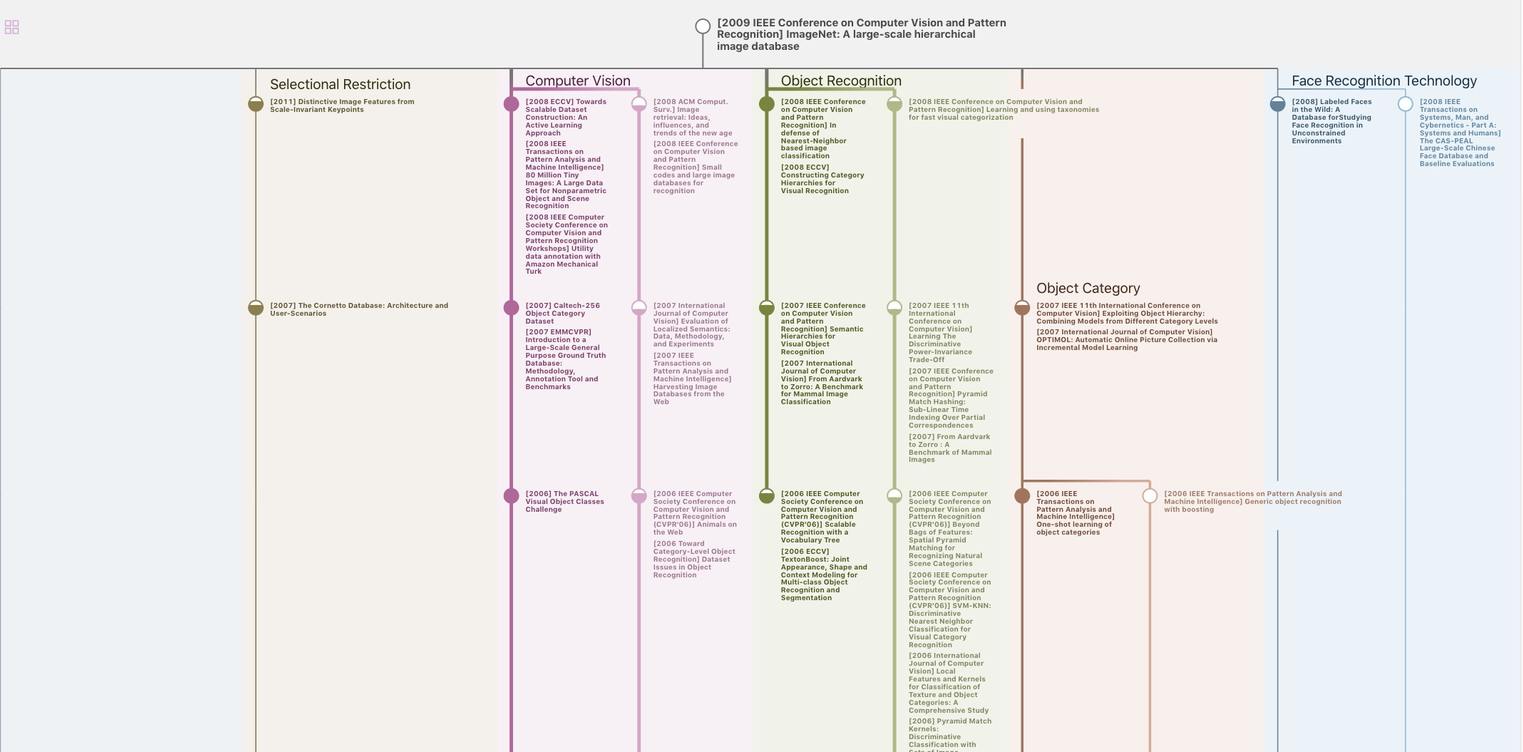
生成溯源树,研究论文发展脉络
Chat Paper
正在生成论文摘要