ARGUS: Rapid Wildfire Tracking Using Satellite Data Collections
2023 IEEE 16th International Conference on Cloud Computing (CLOUD)(2023)
摘要
Interactive visual analytics over distributed systems housing voluminous datasets is hindered by three main factors - disk and network I/O, and data processing overhead. Requests over geospatial data are prone to erratic query load and hotspots due to users' simultaneous interest over a small sub-domain of the overall data space at a time. Interactive analytics in a distributed setting is further hindered in cases of voluminous datasets with large/high-dimensional data objects, such as multi-spectral satellite imagery. The size of the data objects prohibits efficient caching mechanisms that could significantly reduce response latencies. Additionally, extracting information from these large data objects incurs significant data processing overheads and they often entail resource-intensive computational methods. Here, we present our framework, Argus,that extracts low-dimensional representation (embeddings) of high-dimensional satellite images during ingestion and houses them in the cache for use in model-driven analysis relating to wildfire detection. These embeddings are versatile and are used to perform model-based extraction of analytical information for a set of different scenarios, to reduce the high computational costs that are involved with typical transformations over high-dimensional datasets. The models for each such analytical process are trained in a distributed manner in a connected, multi-task learning fashion, along with the encoder network that generates the original embeddings.
更多查看译文
关键词
embedding,multi-task learning,science-guided machine learning,visual analytics
AI 理解论文
溯源树
样例
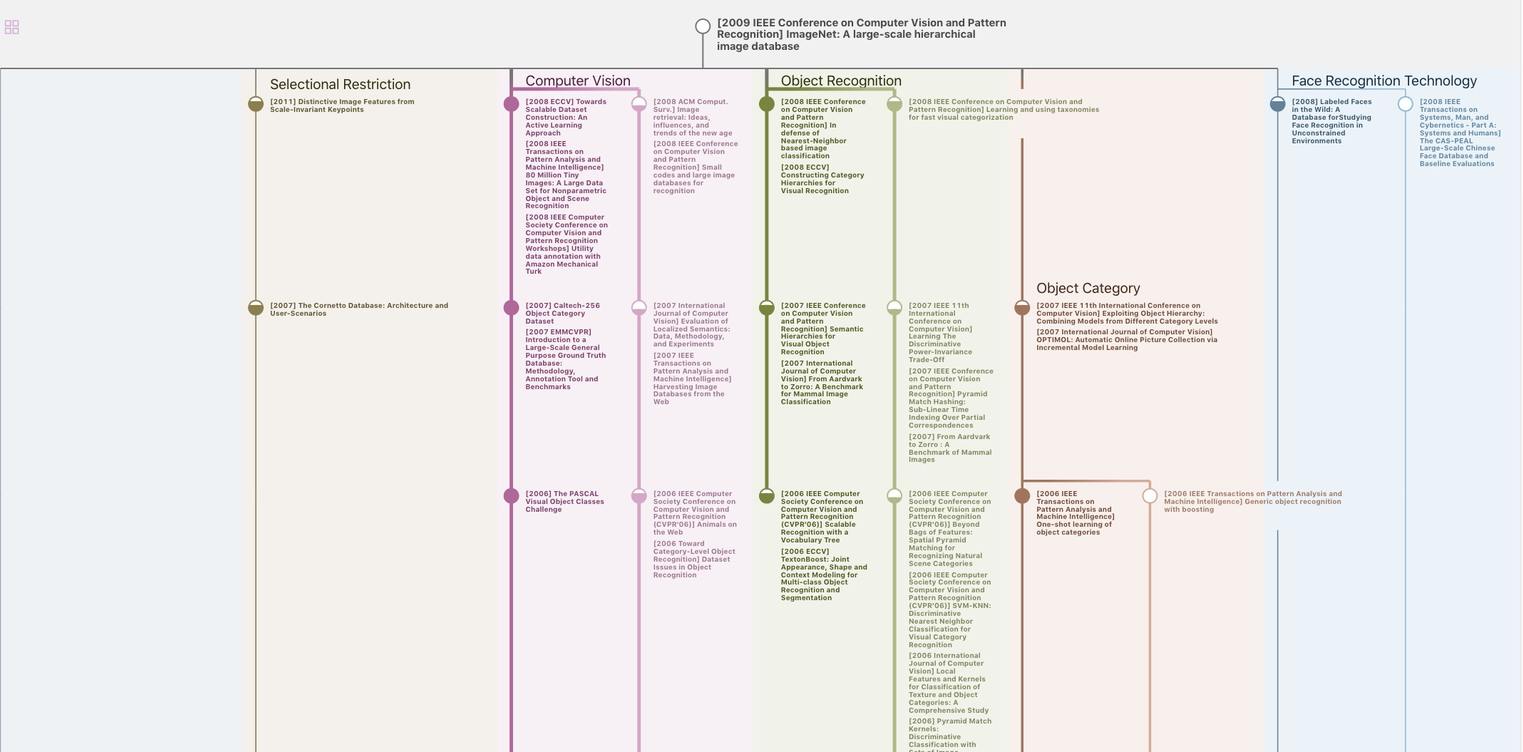
生成溯源树,研究论文发展脉络
Chat Paper
正在生成论文摘要