Online Boosted Gaussian Learners for In-Situ Detection and Characterization of Protein Folding States in Molecular Dynamics Simulations
2023 IEEE 19th International Conference on e-Science (e-Science)(2023)
摘要
Molecular Dynamics (MD) simulations are a crucial tool for understanding how proteins fold. In its easiest form, MD simulations can be scaled through data parallelism, this means that multiple folding trajectories can be spawned and executed in parallel, facilitating a more efficient exploration of the protein folding space. However, due to data dependencies, the analysis of MD simulations remains largely as a centralized process. In this work, we propose a data parallel, lightweight technique to learn the characteristics of protein folding states in MD simulations. Contrary to other methods, ours can differentiate relevant states in a single protein folding trajectory without requiring centralized global knowledge of the protein dynamics. As its processing and memory overheads are negligible (in the order of milliseconds per window of frames, and kilo bytes respectively) this technique can be coupled with the simulation for in-situ analysis.
更多查看译文
关键词
protein folding states,molecular dynamics simulations,in-situ
AI 理解论文
溯源树
样例
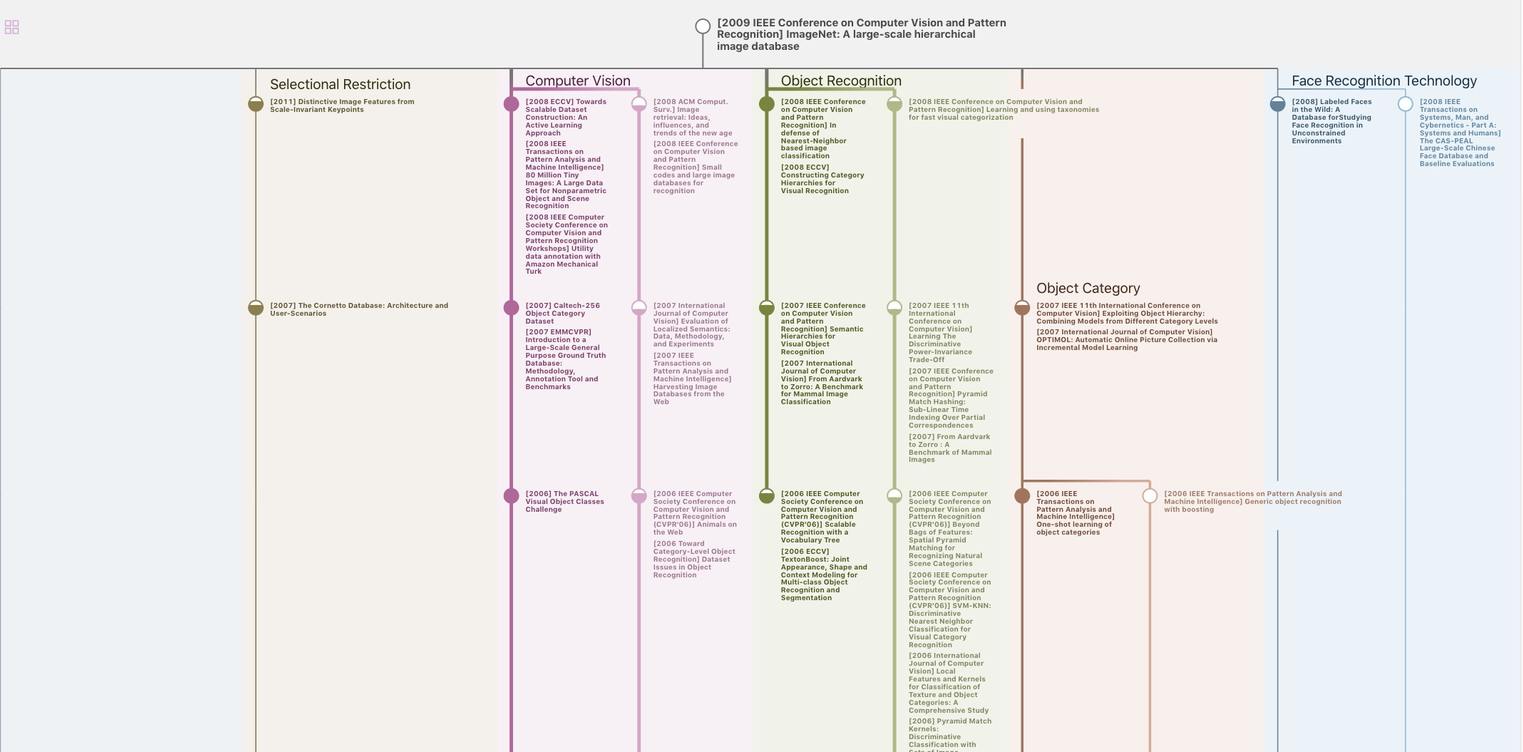
生成溯源树,研究论文发展脉络
Chat Paper
正在生成论文摘要