Symbolic Regression Applied to Cosmology: an Approximate Expression for the Density Perturbation Variance
e-Science(2023)
摘要
Computations of cosmological properties, such as the density perturbation variance, $\sigma$ , are computationally expensive. In this work we propose the application of Symbolic Regression to generate analytical expressions to approximate these quantities. We created simulated data for $\sigma$ using a Boltzmann solver, CAMB. These simulations cover seven parameters; the five cosmological parameters and the redshift and mass of dark matter halos. We then apply a Symbolic Regression engine, TuringBot, to this simulated data and obtain an analytical equation to approximate $\sigma$ . The resulting mathematical expression has a mean accuracy of ≈ 98.96% over the entire domain, and is five orders of magnitude faster than using simulated data from CAMB, demonstrating the applicability of Symbolic Regression to accelerate cosmological inference.
更多查看译文
关键词
Astrophysics,Cosmology,Machine Learning,Symbolic Regression
AI 理解论文
溯源树
样例
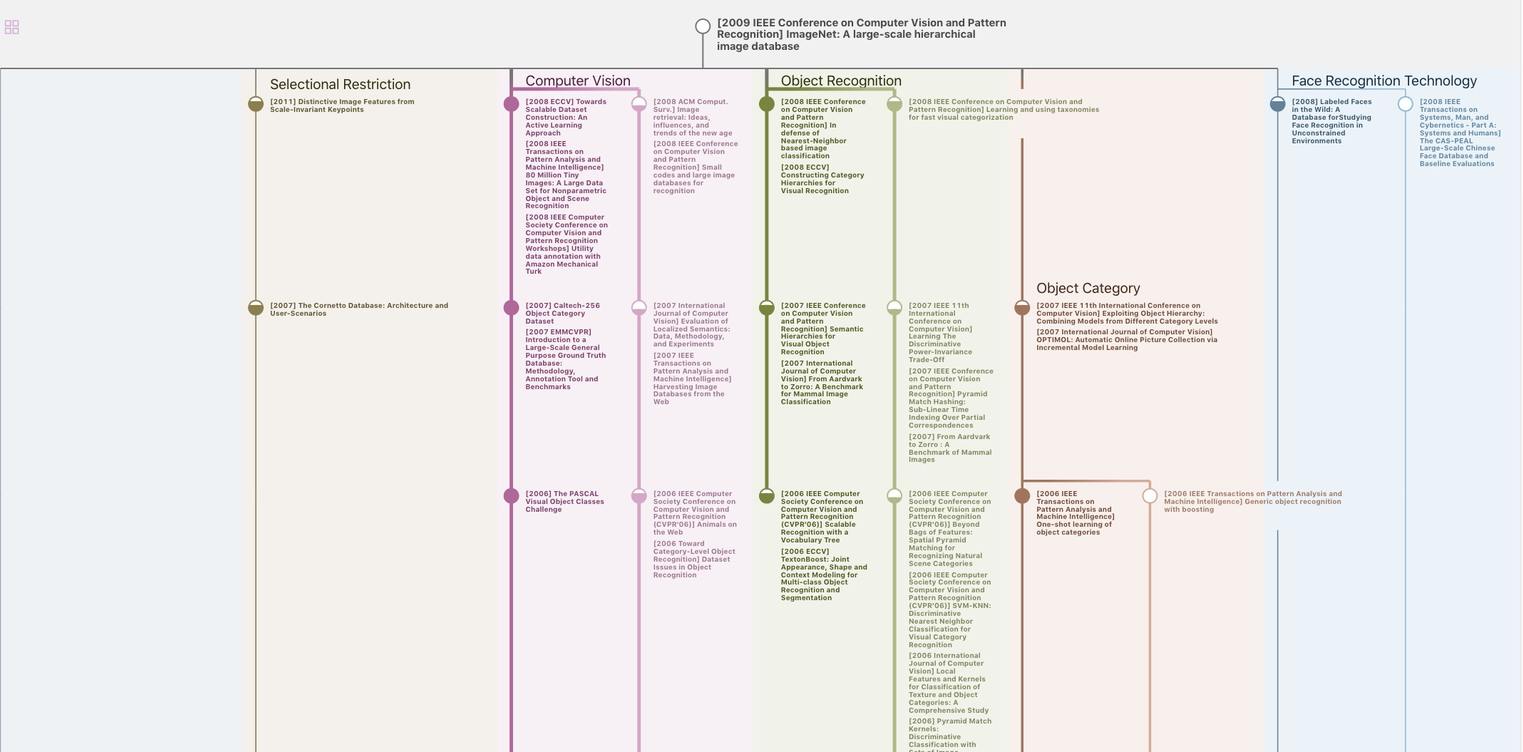
生成溯源树,研究论文发展脉络
Chat Paper
正在生成论文摘要