EFCKD: Edge-Assisted Federated Contrastive Knowledge Distillation Approach for Energy Management: Energy Theft Perspective
2023 24st Asia-Pacific Network Operations and Management Symposium (APNOMS)(2023)
摘要
The widespread deployment of the smart meters makes it possible to record massive fine-grained energy consumption data. However, the end energy users (e.g., prosumers) may tamper with their smart meters to mitigate the energy usage record fraudulently so as to reduce their payment, called energy theft. This behavior can result in poor energy management decision-making and economic losses for the power utilities. Therefore, it is pivotally important to take energy theft detection into consideration for ameliorating energy management. To this end, in this paper, an edge-assisted federated contrastive knowledge distillation (EFCKD) approach is proposed for energy management in terms of energy theft detection aspect towards a prosumer-based urban area, where gathering data in the power utility side is not required and the purpose is to achieve the average energy theft detection loss. Concretely, each client located on an edge server contains a local teacher model (LTM) and a local student model (LSM), where the LSM is a copy of the shared global student model (SGSM). The knowledge of each teacher network is distilled to teach the corresponding student network, while LSMs are collaboratively learned to update SGSM. In addition, model-contrastive learning is introduced to ameliorate performance. Experiments show that the proposed EFCKD outperforms the benchmarks since it can achieve the lowest average loss (0.0104).
更多查看译文
关键词
Prosumer-based urban area,energy theft detection,model-contrastive learning,knowledge distillation,federated learning
AI 理解论文
溯源树
样例
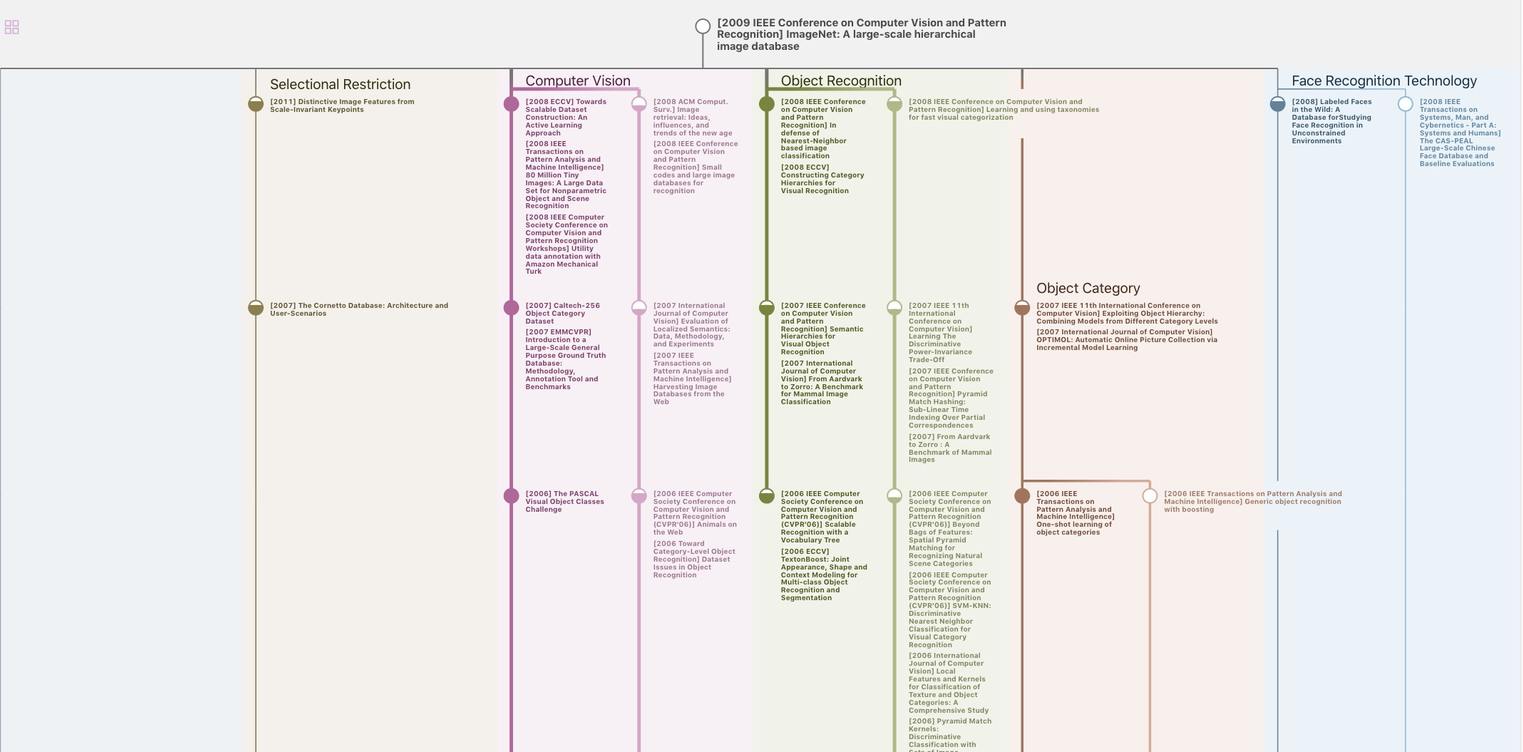
生成溯源树,研究论文发展脉络
Chat Paper
正在生成论文摘要