End-to-End High-Level Control of Lower-Limb Exoskeleton for Human Performance Augmentation Based on Deep Reinforcement Learning
IEEE Access(2023)
摘要
This paper proposes a novel end-to-end controller for the lower-limb exoskeleton for human performance augmentation (LEHPA) systems based on deep reinforcement learning (E2EDRL). The model-free controller contains two control levels: the high-level control responsible for end-to-end human motion intention recognition based on the exoskeleton state signals and the human-exoskeleton interaction (HEI) force signals by deep neural network predictor, and the low-level control for motion tracking by joint PD controllers. The deep neural network predictor does not require complex kinematic calculations that are inevitable in conventional human motion intention recognition methods. We execute the learning process in simulation to learn the E2EDRL strategy efficiently and safely by constructing a novel multibody simulation environment and proposing its specific hybrid inverse-forward dynamics simulation method. The passive mode (all joints remain unpowered) is introduced as a benchmark for comparison purposes. A novel performance assessment method based on HEI forces is put forward to evaluate the E2EDRL strategy quantitatively. The global ratio of the HEI forces in the E2EDRL strategy relative to those in the passive mode is as low as 0.65. The global reduction of the HEI forces demonstrates the superior control performance of the E2EDRL strategy.
更多查看译文
关键词
Lower-limb exoskeleton for human performance augmentation,end-to-end human motion intention recognition,deep reinforcement learning
AI 理解论文
溯源树
样例
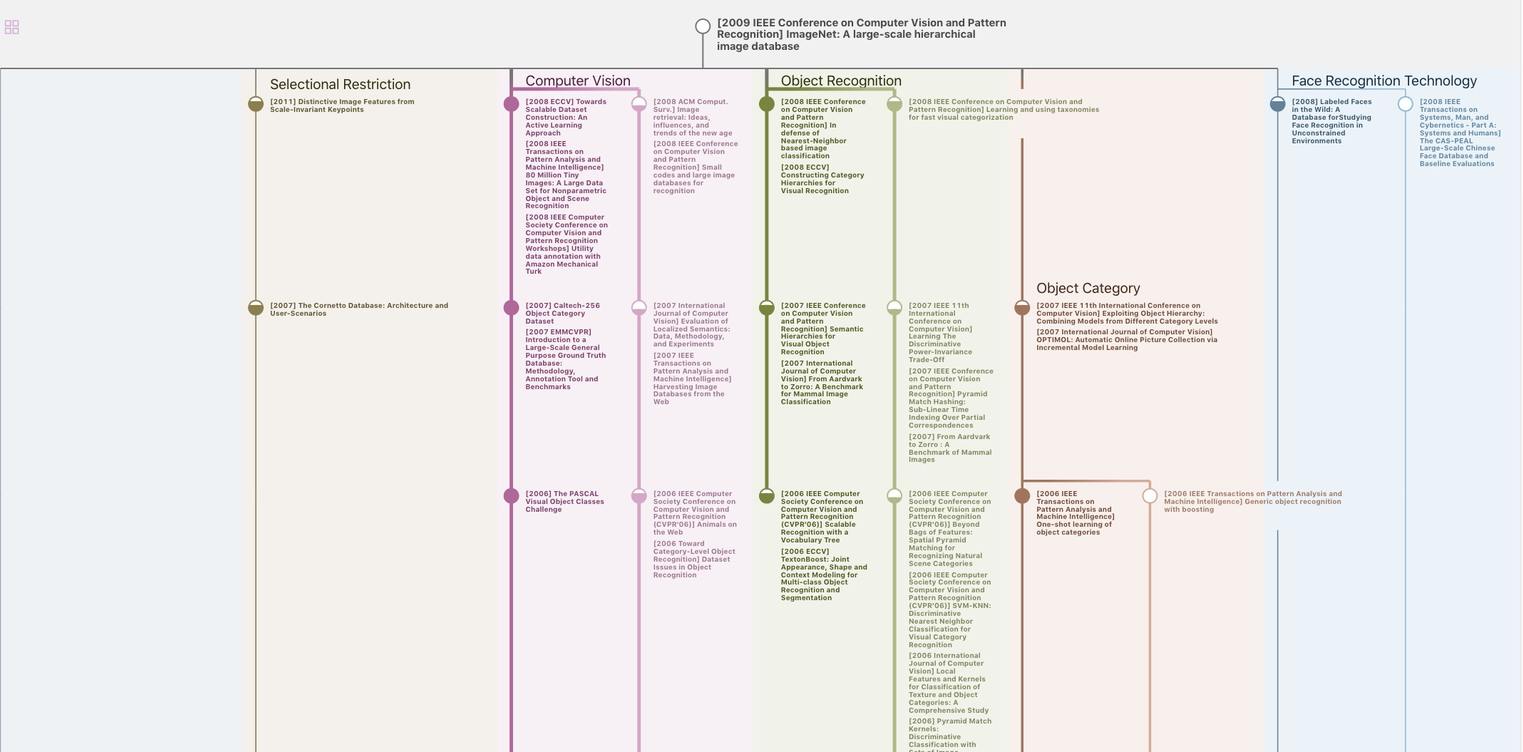
生成溯源树,研究论文发展脉络
Chat Paper
正在生成论文摘要