Exploring RWKV for Memory Efficient and Low Latency Streaming ASR
CoRR(2023)
摘要
Recently, self-attention-based transformers and conformers have been introduced as alternatives to RNNs for ASR acoustic modeling. Nevertheless, the full-sequence attention mechanism is non-streamable and computationally expensive, thus requiring modifications, such as chunking and caching, for efficient streaming ASR. In this paper, we propose to apply RWKV, a variant of linear attention transformer, to streaming ASR. RWKV combines the superior performance of transformers and the inference efficiency of RNNs, which is well-suited for streaming ASR scenarios where the budget for latency and memory is restricted. Experiments on varying scales (100h - 10000h) demonstrate that RWKV-Transducer and RWKV-Boundary-Aware-Transducer achieve comparable to or even better accuracy compared with chunk conformer transducer, with minimal latency and inference memory cost.
更多查看译文
关键词
rwkv,memory efficient
AI 理解论文
溯源树
样例
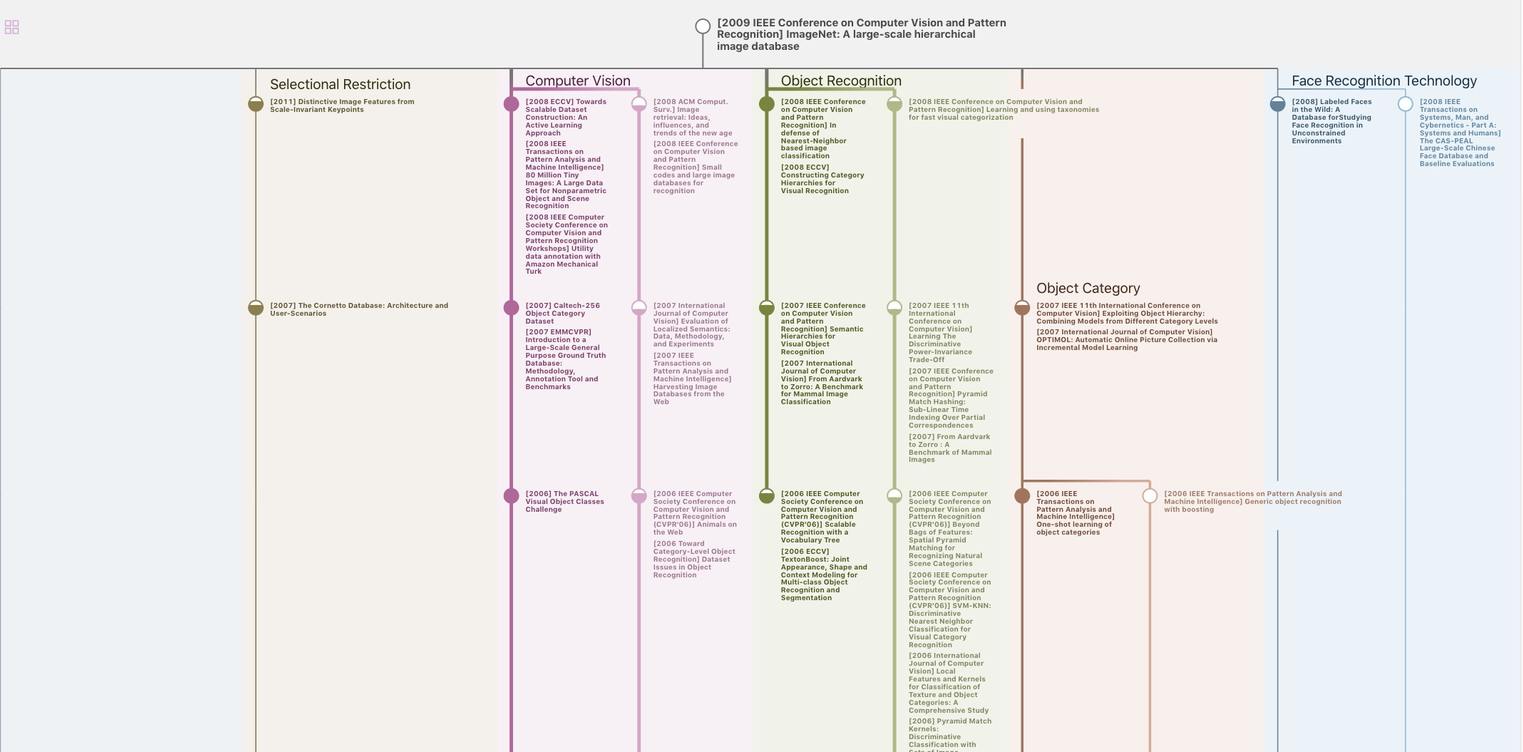
生成溯源树,研究论文发展脉络
Chat Paper
正在生成论文摘要