Context-Aware Generative Models for Prediction of Aircraft Ground Tracks
CoRR(2023)
摘要
Trajectory prediction (TP) plays an important role in supporting the decision-making of Air Traffic Controllers (ATCOs). Traditional TP methods are deterministic and physics-based, with parameters that are calibrated using aircraft surveillance data harvested across the world. These models are, therefore, agnostic to the intentions of the pilots and ATCOs, which can have a significant effect on the observed trajectory, particularly in the lateral plane. This work proposes a generative method for lateral TP, using probabilistic machine learning to model the effect of the epistemic uncertainty arising from the unknown effect of pilot behaviour and ATCO intentions. The models are trained to be specific to a particular sector, allowing local procedures such as coordinated entry and exit points to be modelled. A dataset comprising a week's worth of aircraft surveillance data, passing through a busy sector of the United Kingdom's upper airspace, was used to train and test the models. Specifically, a piecewise linear model was used as a functional, low-dimensional representation of the ground tracks, with its control points determined by a generative model conditioned on partial context. It was found that, of the investigated models, a Bayesian Neural Network using the Laplace approximation was able to generate the most plausible trajectories in order to emulate the flow of traffic through the sector.
更多查看译文
关键词
aircraft,prediction,models,ground,context-aware
AI 理解论文
溯源树
样例
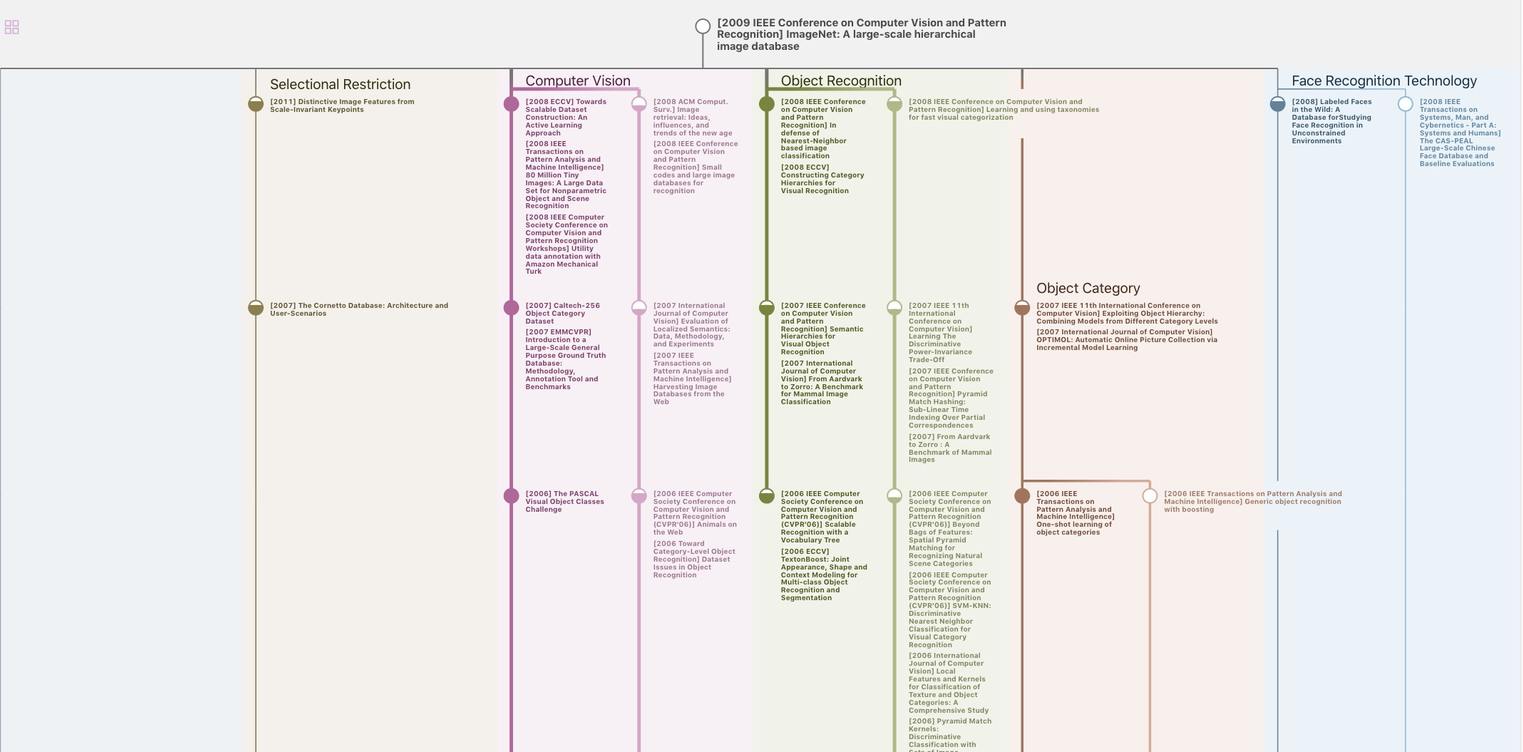
生成溯源树,研究论文发展脉络
Chat Paper
正在生成论文摘要