C2BNet: A Deep Learning Architecture with Coupled Composite Backbone for Parasitic EGG Detection in Microscopic Images.
IEEE journal of biomedical and health informatics(2023)
摘要
Internet of Medical Things (IoMT) enabled by artificial intelligence (AI) technologies can facilitate automatic diagnosis and management of chronic diseases (e.g., intestinal parasitic infection) based on two-dimensional (2D) microscopic images. To improve the model performance of object detection challenged by microscopic image characteristics (e.g., focus failure, motion blur, and whether zoomed or not), we propose Coupled Composite Backbone Network (C2BNet) to execute the parasitic egg detection task using 2D microscopic images. In particular, the C2BNet backbone adopts a two-path structure-based backbone and leverages model heterogeneity to learn object features from different perspectives. A novel feature composition style is proposed to flow the feature within the coupled composite backbone, and ensure mutual enhancement of feature representation ability among the different paths of the backbone. To further improve the accuracy of the detection results, we propose Multiscale Weighted Box Fusion (WBF) to fuse the location and confidence scores of all bounding boxes predicted from the multiscale feature maps, and iteratively refine the box coordinates to form the final prediction. Experimental results on Chula-ParasiteEgg-11 dataset demonstrate that the C2BNet not only performs satisfactorily compared with state-of-the-art methods, but also can focus more on learning detailed morphology features and abundant semantic features, resulting in more precise detection for parasitic eggs located in the 2D microscopic image.
更多查看译文
关键词
IoMT,deep learning,chronic disease diagnosis,parasitic egg detection,C2BNet,multi-scale WBF
AI 理解论文
溯源树
样例
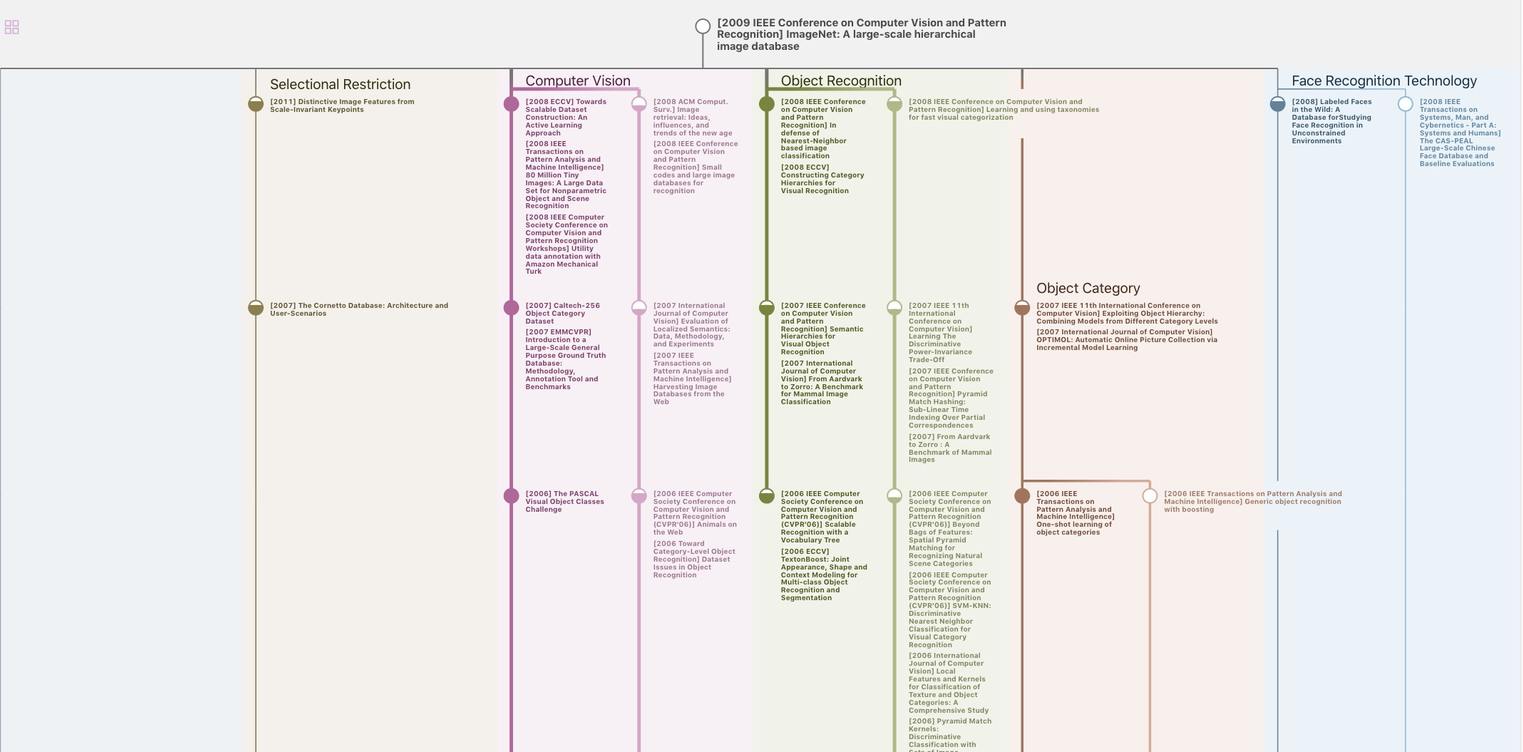
生成溯源树,研究论文发展脉络
Chat Paper
正在生成论文摘要