A Graph Neural Network Model with a Transparent Decision-Making Process Defines the Applicability Domain for Environmental Estrogen Screening.
Environmental science & technology(2023)
摘要
The application of deep learning (DL) models for screening environmental estrogens (EEs) for the sound management of chemicals has garnered significant attention. However, the currently available DL model for screening EEs lacks both a transparent decision-making process and effective applicability domain (AD) characterization, making the reliability of its prediction results uncertain and limiting its practical applications. To address this issue, a graph neural network (GNN) model was developed to screen EEs, achieving accuracy rates of 88.9% and 92.5% on the internal and external test sets, respectively. The decision-making process of the GNN model was explored through the network-like similarity graphs (NSGs) based on the model features (FT). We discovered that the accuracy of the predictions is dependent on the feature distribution of compounds in NSGs. An AD characterization method called AD was proposed, which excludes predictions falling outside of the model's prediction range, leading to a 15% improvement in the F1 score of the GNN model. The GNN model with the AD method may serve as an efficient tool for screening EEs, identifying 800 potential EEs in the Inventory of Existing Chemical Substances of China. Additionally, this study offers new insights into comprehending the decision-making process of DL models.
更多查看译文
关键词
environmental estrogens, deep learning, graphneural network, decision-making process, applicabilitydomain
AI 理解论文
溯源树
样例
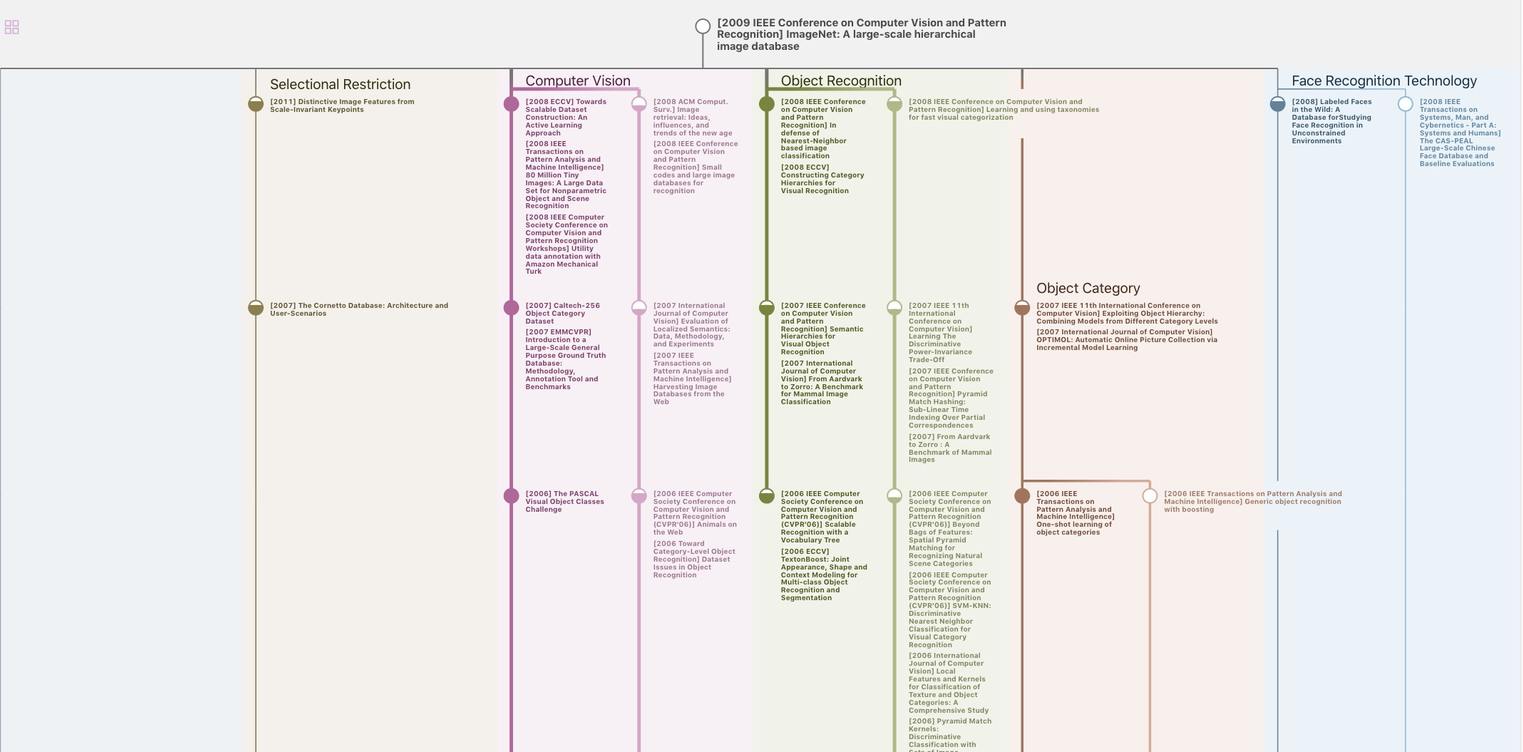
生成溯源树,研究论文发展脉络
Chat Paper
正在生成论文摘要