Iqc: Machine-Learning-driven Prediction of Surgical Procedure Uncovers Systematic Confounds of Cancer Whole Slide Images in Specific Medical Centers
medrxiv(2023)
摘要
Problem The past decades have yielded an explosion of research using artificial intelligence for cancer detection and diagnosis in the field of computational pathology. Yet, an often unspoken assumption of this research is that a glass microscopy slide faithfully represents the underlying disease. Here we show systematic failure modes may dominate the slides digitized from a given medical center, such that neither the whole slide images nor the glass slides are suitable for rendering a diagnosis. Methods We quantitatively define high quality data as a set of whole slide images where the type of surgery the patient received may be accurately predicted by an automated system such as ours, called “iQC”. We find iQC accurately distinguished biopsies from nonbiopsies, e.g. prostatectomies or transurethral resections (TURPs, a.k.a. prostate chips), only when the data qualitatively appeared to be high quality, e.g. vibrant histopathology stains and minimal artifacts. Crucially, prostate needle biopsies appear as thin strands of tissue, whereas prostatectomies and TURPs appear as larger rectangular blocks of tissue. Therefore, when the data are of high quality, iQC (i) accurately classifies pixels as tissue, (ii) accurately generates statistics that describe the distribution of tissue in a slide, and (iii)accurately predicts surgical procedure from said statistics. We additionally compare our “iQC” to “HistoQC”, both in terms of how many slides are excluded and how much tissue is identified in the slides. Results While we do not control any medical center’s protocols for making or storing slides, we developed the iQC tool to hold all medical centers and datasets to the same objective standard of quality. We validate this standard across five Veterans Affairs Medical Centers (VAMCs) and the Automated Gleason Grading Challenge (AGGC) 2022 public dataset. For our surgical procedure prediction task, we report an Area Under Receiver Operating Characteristic (AUROC) of 0.9966-1.000 at the VAMCs that consistently produce high quality data and AUROC of 0.9824 for the AGGC dataset. In contrast, we report an AUROC of 0.7115 at the VAMC that consistently produced poor quality data. An attending pathologist determined poor data quality was likely driven by faded histopathology stains and protocol differences among VAMCs. Corroborating this, iQC’s novel stain strength statistic finds this institution has significantly weaker stains ( p < 2.2 × 10 − 16, two-tailed Wilcoxon rank-sum test) than the VAMC that contributed the most slides, and this stain strength difference is a large effect (Cohen’s d = 1.208). In addition to accurately detecting the distribution of tissue in slides, we find iQC recommends only 2 of 3736 VAMC slides (0.005%) be reviewed for inadequate tissue. With its default configuration file, HistoQC excluded 89.9% of VAMC slides because tissue was not detected in these slides. With our customized configuration file for HistoQC, we reduced this to 16.7% of VAMC slides. Strikingly, the default configuration of HistoQC included 94.0% of the 1172 prostate cancer slides from The Cancer Genome Atlas (TCGA), which may suggest HistoQC defaults were calibrated against TCGA data but this calibration did not generalize well to non-TCGA datasets. For VAMC and TCGA, we find a negligible to small degree of agreement in the include/exclude status of slides, which may suggest iQC and HistoQC are not equivalent. Conclusion Our surgical procedure prediction AUROC may be a quantitative indicator positively associated with high data quality at a medical center or for a specific dataset. We find iQC accurately identifies tissue in slides and excludes few slides, unless the data are poor quality. To produce high quality data, we recommend producing slides using robotics or other forms of automation whenever possible. We recommend scanning slides digitally before the glass slide has time to develop signs of age, e.g faded stains and acrylamide bubbles. We recommend using high-quality reagents to stain and mount slides, which may slow aging. We recommend protecting stored slides from ultraviolet light, from humidity, and from changes in temperature. To our knowledge, iQC is the first automated system in computational pathology that validates data quality against objective evidence, e.g. surgical procedure data available in the EHR or LIMS, which requires zero efforts or annotations from anatomic pathologists. Please see and for instructions and updates. ### Competing Interest Statement The authors have declared no competing interest. ### Funding Statement This work was funded through a Prostate Cancer Foundation grant PCFCHAL22 to MBR, BSK, IPG, and SP. Support for the GenISIS platform was additionally provided by the United States Veterans Administration (VA) Office of Research and Development (ORD) through SP. ### Author Declarations I confirm all relevant ethical guidelines have been followed, and any necessary IRB and/or ethics committee approvals have been obtained. Yes The details of the IRB/oversight body that provided approval or exemption for the research described are given below: Ethics committee/IRB of Veterans Affairs Boston Healthcare System, Boston, VA waived ethical approval of this work. This is a human subjects exempt study of archived pathology specimens. I confirm that all necessary patient/participant consent has been obtained and the appropriate institutional forms have been archived, and that any patient/participant/sample identifiers included were not known to anyone (e.g., hospital staff, patients or participants themselves) outside the research group so cannot be used to identify individuals. Yes I understand that all clinical trials and any other prospective interventional studies must be registered with an ICMJE-approved registry, such as ClinicalTrials.gov. I confirm that any such study reported in the manuscript has been registered and the trial registration ID is provided (note: if posting a prospective study registered retrospectively, please provide a statement in the trial ID field explaining why the study was not registered in advance). Yes I have followed all appropriate research reporting guidelines, such as any relevant EQUATOR Network research reporting checklist(s) and other pertinent material, if applicable. Yes All data produced in the present study are available in a de-identified format on the Veterans Affairs GenISIS platform for researchers upon request and approval.
更多查看译文
AI 理解论文
溯源树
样例
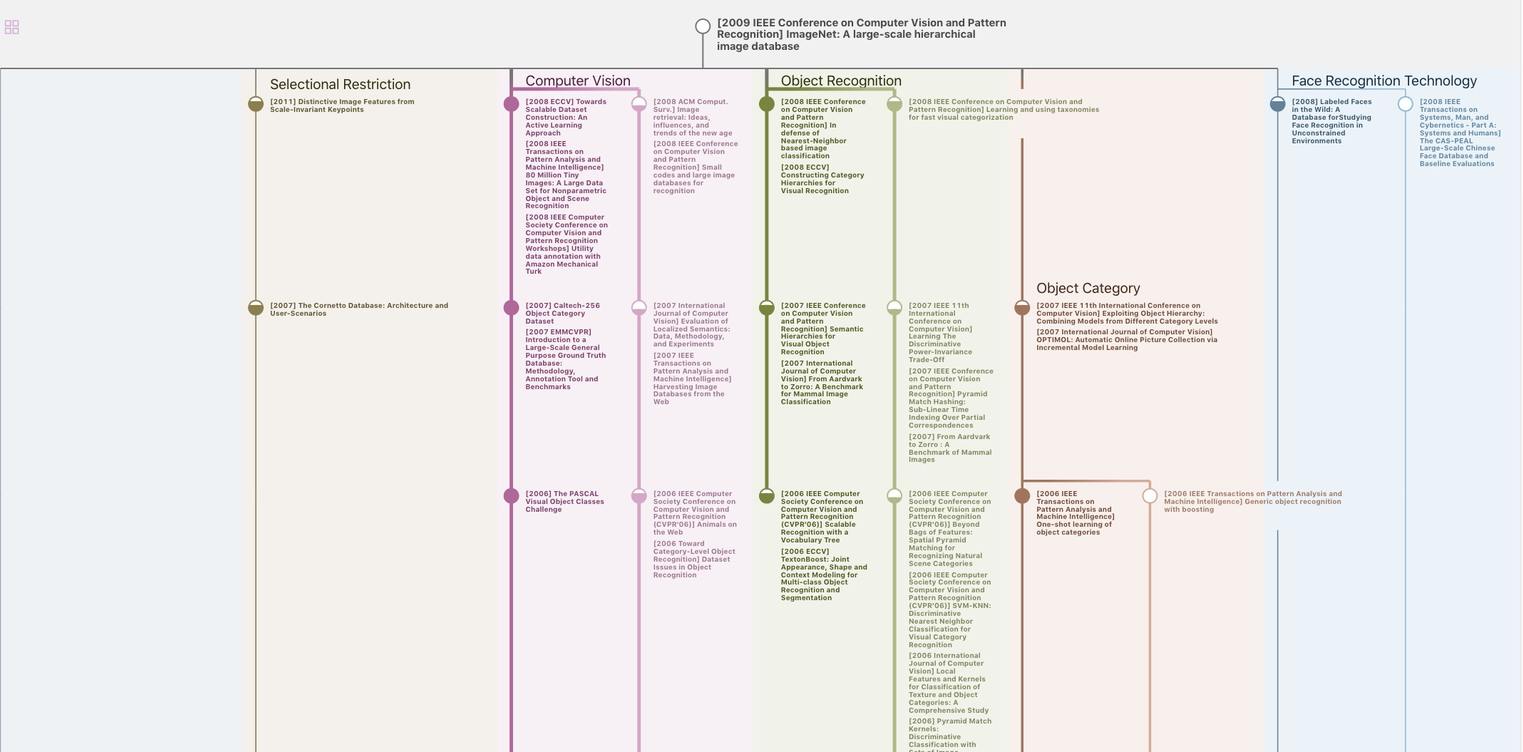
生成溯源树,研究论文发展脉络
Chat Paper
正在生成论文摘要