Fast clinical trial identification using fuzzy-search elastic searches: retrospective validation with high-quality Cochrane benchmark
medrxiv(2023)
摘要
Evidence-based medicine relies on systematic reviews of randomized controlled trials and other clinical intervention studies to synthesize the latest and most valid estimates of treatment effects. However, conducting these systematic reviews is time-consuming and labor-intensive, requiring careful design of sensitive search queries and manual identification of relevant studies.
New database architectures and natural language processing (NLP) techniques have recently emerged that may streamline the systematic review process. These new approaches allow fast and fuzzy searches using non-Boolean queries and automatically extracting meta-information from unstructured database texts.
Our study compares the effectiveness of NLP-based literature searches within a new database structure to the yield of the Cochrane Database of Systematic Reviews study sets – currently the gold standard. We built a stand-alone, freely available, fuzzy-enabled elastic search database containing all 36 million PubMed-indexed entries. The database is daily synchronized with PubMed. We developed and validated reliable filters to identify randomized clinical trials and other clinical intervention studies and extract Population and Intervention-relevant subtext.
Relevant subtexts were detected with a precision of 0.74, recall of 0.81, and F1-score of 0.77 for the Population subtext, and a precision of 0.70, recall of 0.71, and an F1-score of 0.70 for the Intervention subtext. We found that short, user-friendly, and approximate queries were valuable in rapidly identifying a list of included studies within a random set of Cochrane intervention reviews. In 90% of systematic reviews (27/30), the new search strategy missed no more than two of all included trials by Cochrane, yet keeping the total hits lower compared to a comparable PubMed keyword search (87%; 26/30). This identification suggests that NLP-based literature searches within a new database structure on top of PubMed can be a promising approach for conducting and updating aggregated clinical evidence more efficiently and effectively.
### Competing Interest Statement
The authors have declared no competing interest.
### Funding Statement
This study did not receive any funding
### Author Declarations
I confirm all relevant ethical guidelines have been followed, and any necessary IRB and/or ethics committee approvals have been obtained.
Yes
I confirm that all necessary patient/participant consent has been obtained and the appropriate institutional forms have been archived, and that any patient/participant/sample identifiers included were not known to anyone (e.g., hospital staff, patients or participants themselves) outside the research group so cannot be used to identify individuals.
Yes
I understand that all clinical trials and any other prospective interventional studies must be registered with an ICMJE-approved registry, such as [ClinicalTrials.gov][1]. I confirm that any such study reported in the manuscript has been registered and the trial registration ID is provided (note: if posting a prospective study registered retrospectively, please provide a statement in the trial ID field explaining why the study was not registered in advance).
Yes
I have followed all appropriate research reporting guidelines, such as any relevant EQUATOR Network research reporting checklist(s) and other pertinent material, if applicable.
Yes
All data produced in the present study are available upon reasonable request to the authors
[1]: http://ClinicalTrials.gov
更多查看译文
AI 理解论文
溯源树
样例
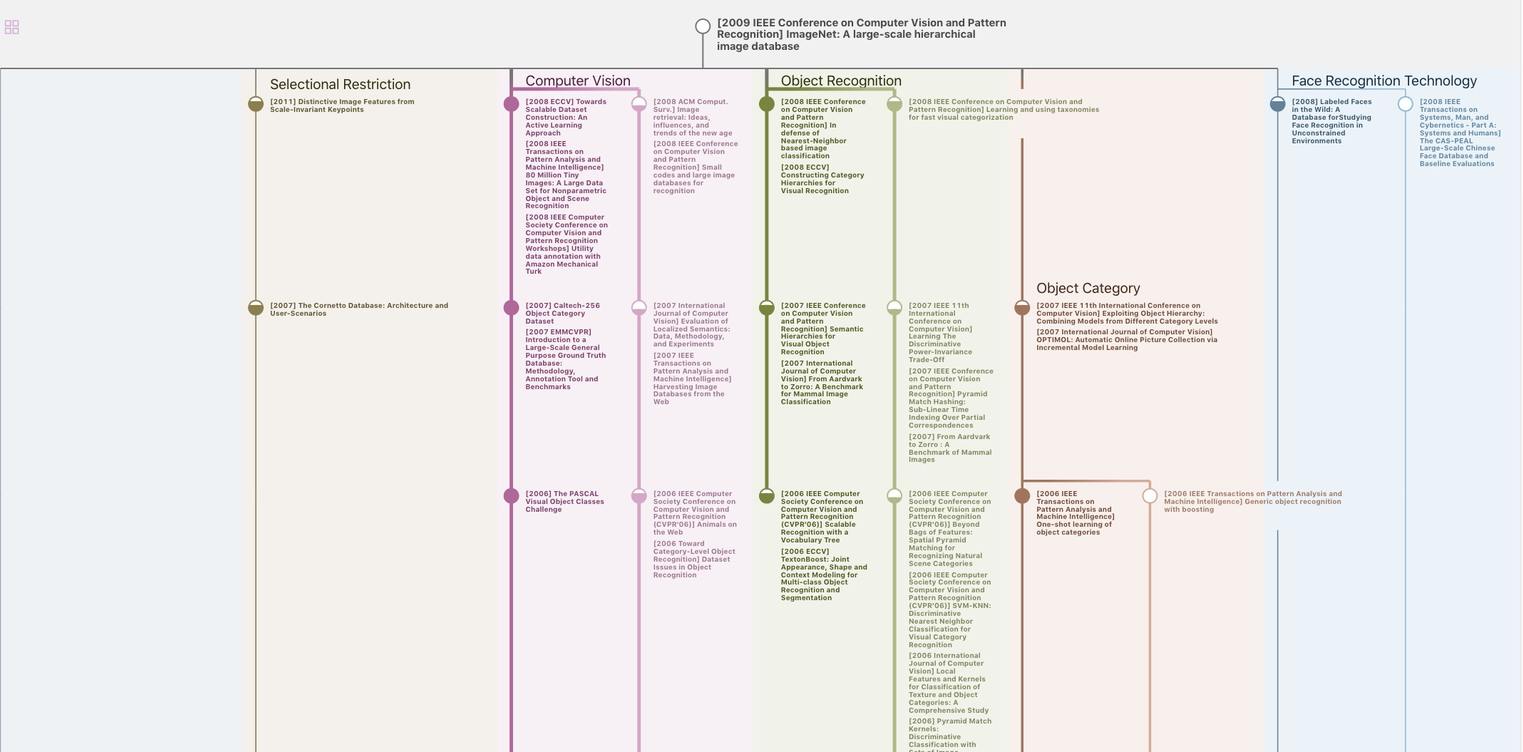
生成溯源树,研究论文发展脉络
Chat Paper
正在生成论文摘要