Framingham risk score prediction at 12 months in the STANDFIRM randomised control trial
medRxiv (Cold Spring Harbor Laboratory)(2023)
摘要
Background The Shared Team Approach between Nurses and Doctors For Improved Risk factor Management (STANDFIRM, ACTRN12608000166370) trial was designed to test the effectiveness of chronic disease care management for modifying the Framingham risk score (FRS) among patients with stroke or transient ischemic attack. The primary outcome of change in FRS between baseline and 12 months was not met. We aimed to determine characteristics of participants at baseline that predict reduction in FRS at 12 months and whether future FRS is predetermined at the time of randomization
Method Data included 35 variables encompassing demographics, risk factors, psychological, social and education status, and laboratory tests. Five supervised machine learning (ML) methods were used: random forest (RF), extreme gradient boosting (XGBoost), support vector regression (SVR), multilayer perceptron artificial neural network (MLP) and K-nearest neighbor (KNN). We split data for training (80%, n=406) and testing (20%, n=102).
Results Training and test data were evenly matched for age, sex, baseline and 12-month FRS. Following tuning of the five ML methods, the optimal model for predicting FRS at 12 months was SVR (R2=0.763, root mean squared error or RMSE=8.52). The five most important variables for SVR were: baseline FRS, age, male sex, sodium/potassium excretion and proteinuria. All ML methods were poor at determining change in FRS at 12 months (R2<0.161).
Conclusion Our findings suggest that change in FRS as an endpoint in trials may have limited value as it is largely determined at baseline. In this cohort, Support Vector Regression was the optimal method to predict future but not change in FRS.
### Competing Interest Statement
The authors have declared no competing interest.
### Clinical Trial
ACTRN12608000166370
### Clinical Protocols
### Funding Statement
Funding was by the National Health and Medical Research Council (586605 (trial), 1042600 (fellowship for AGT), 1063761, 1061457 (fellowship for DAC)
### Author Declarations
I confirm all relevant ethical guidelines have been followed, and any necessary IRB and/or ethics committee approvals have been obtained.
Yes
The details of the IRB/oversight body that provided approval or exemption for the research described are given below:
Alfred Hospital Research & Ethics Committee Ethics committee address [1] Commercial Road Prahran 3181 Ethics committee country [1] Australia Date submitted for ethics approval [1] 25/03/2008 Approval date [1] 02/05/2008 Ethics approval number [1] 91/08
I confirm that all necessary patient/participant consent has been obtained and the appropriate institutional forms have been archived, and that any patient/participant/sample identifiers included were not known to anyone (e.g., hospital staff, patients or participants themselves) outside the research group so cannot be used to identify individuals.
Yes
I understand that all clinical trials and any other prospective interventional studies must be registered with an ICMJE-approved registry, such as ClinicalTrials.gov. I confirm that any such study reported in the manuscript has been registered and the trial registration ID is provided (note: if posting a prospective study registered retrospectively, please provide a statement in the trial ID field explaining why the study was not registered in advance).
Yes
I have followed all appropriate research reporting guidelines, such as any relevant EQUATOR Network research reporting checklist(s) and other pertinent material, if applicable.
Yes
The data is available on written request to Dr Amanda Thrift
更多查看译文
关键词
randomised control trial,risk,prediction
AI 理解论文
溯源树
样例
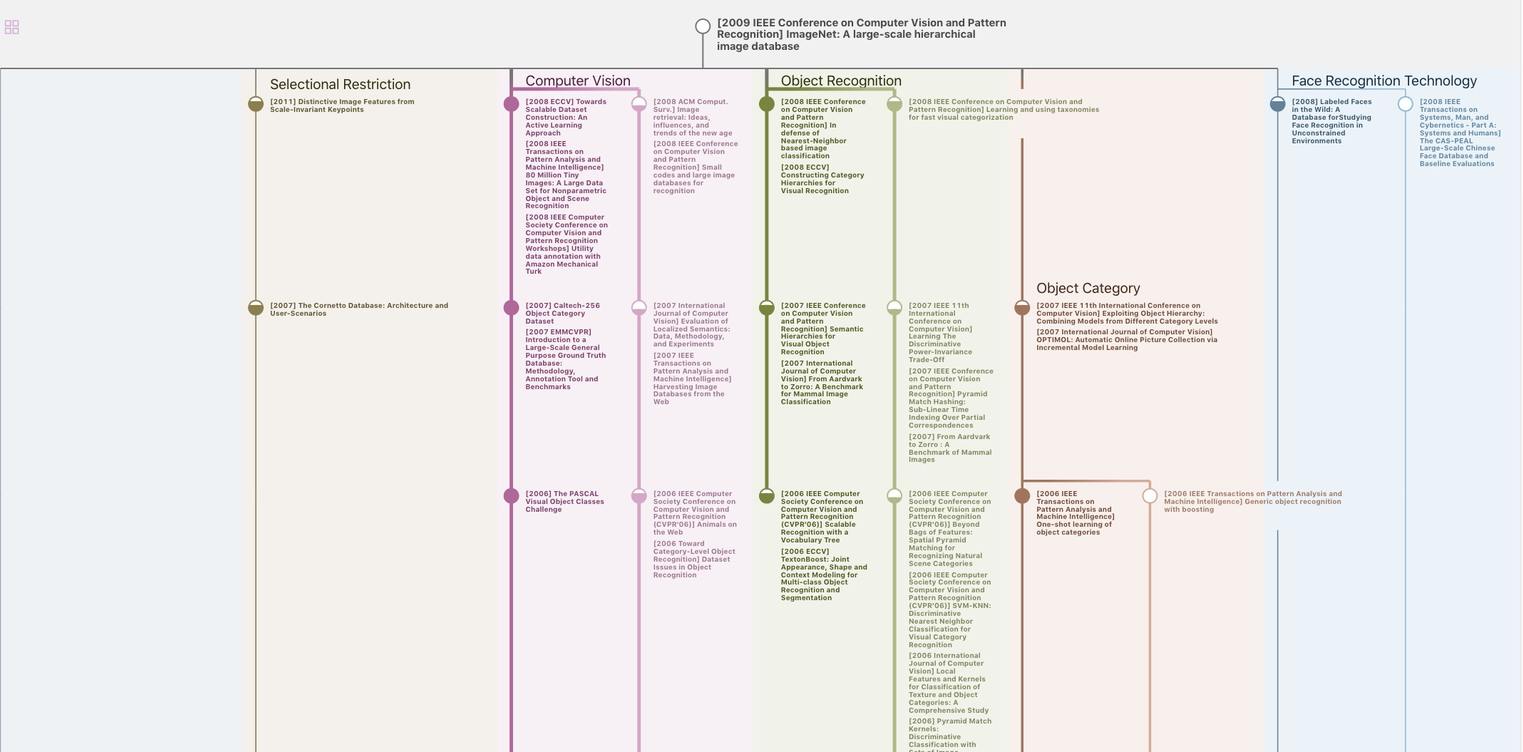
生成溯源树,研究论文发展脉络
Chat Paper
正在生成论文摘要