Sea Level Rise Learning Scenarios for Adaptive Decision-Making Based on IPCC AR6
Earth's Future(2023)
摘要
Adaptation decision-scientists increasingly use real-option analysis to consider the value of learning about future climate variable development in adaptation decisions. Toward this end learning scenarios are needed, which are scenarios that provide information on future variable values seen not only from today (as static scenarios), but also seen from future moments in time. Decision-scientists generally develop learning scenarios themselves, mostly through time-independent (stationary) or highly simplified methods. The climate learning scenarios thus attained generally only poorly represent the uncertainties of state-of-the-art climate science and thus may lead to biased decisions. This paper first motivates the need for learning scenarios by providing a simple example to illustrate characteristics and benefits of learning scenarios. Next, we analyze how well learning scenarios represent climate uncertainties in the context of sea level rise and present a novel method called direct fit to generate climate learning scenarios that outperforms existing methods. This is illustrated by quantifying the difference of the sea level rise learning scenarios created with both methods to the original underlying scenario. The direct fit method is based on pointwise probability distributions, for example, boxplots, and hence can be applied to static scenarios as well as ensemble trajectories. Furthermore, the direct fit method offers a much simpler process for generating learning scenarios from static or "ordinary" climate scenarios. Many climate change adaptation decisions require large investments in infrastructure (e.g., dikes), while at the same time future projections about critical variables (e.g., sea level rise) are highly uncertain. Decision-scientists address these challenges with methods based on flexibility and staged decision-making. For example, a coastal decision-maker could implement a dike with a wider foundation, and, if necessary, upgrade the dike height in the future. The decision-maker will learn by observing future sea level rise if higher dike protection levels are actually necessary in the future. In order to assess whether it is economically beneficial to wait for future learning through observations, and thus to justify additional expenses for flexible infrastructure investments, learning scenarios are required. Learning scenarios provide projections of critical variables seen from today and from future moments in time. For example, learning scenarios of sea level rise contain sea level rise projections seen from 2050 onward, depending on a certain amount of sea level rise observed until 2050. In this paper, we provide a simple example to illustrate coastal decision-making with a learning scenario, propose a new method to generate learning scenarios, and apply this method to generate sea level rise learning scenarios. We show how climate learning scenarios can be applied for improving and justifying investments in flexible long-lasting infrastructureWe develop sea level rise learning scenarios based on Intergovernmental Panel on Climate Change sixth Assessment Report using a novel method termed direct fitOur new method reduces the average deviation of learning scenarios from the original data by 83% compared to standard methods
更多查看译文
关键词
learning scenarios,adaptation,real-option analysis,climate uncertainty,sea level rise
AI 理解论文
溯源树
样例
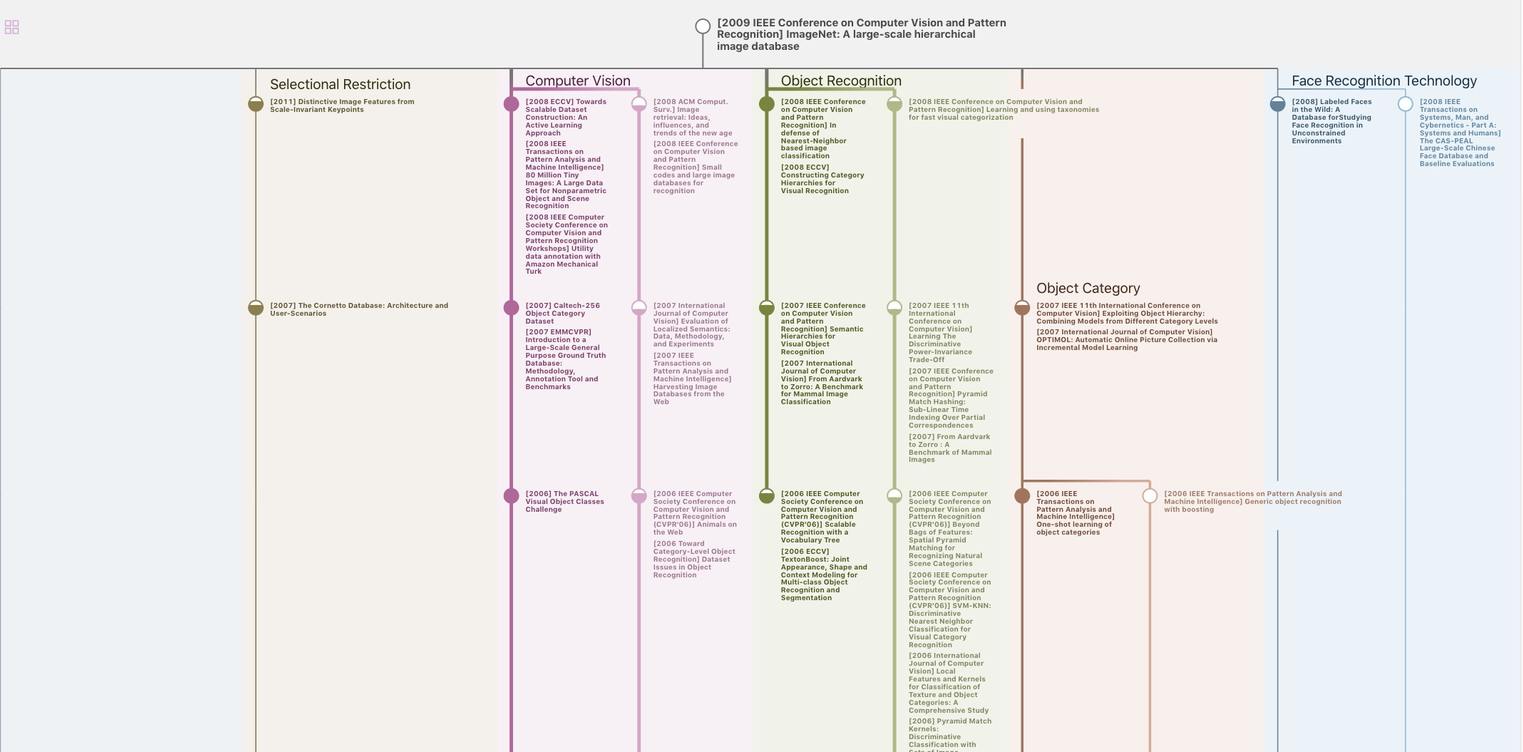
生成溯源树,研究论文发展脉络
Chat Paper
正在生成论文摘要