MHLDet: A Multi-Scale and High-Precision Lightweight Object Detector Based on Large Receptive Field and Attention Mechanism for Remote Sensing Images
Remote Sensing(2023)
摘要
Object detection in remote sensing images (RSIs) has become crucial in recent years. However, researchers often prioritize detecting small objects, neglecting medium- to large-sized ones. Moreover, detecting objects hidden in shadows is challenging. Additionally, most detectors have extensive parameters, leading to higher hardware costs. To address these issues, this paper proposes a multi-scale and high-precision lightweight object detector named MHLDet. Firstly, we integrated the SimAM attention mechanism into the backbone and constructed a new feature-extraction module called validity-neat feature extract (VNFE). This module captures more feature information while simultaneously reducing the number of parameters. Secondly, we propose an improved spatial pyramid pooling model, named SPPE, to integrate multi-scale feature information better, enhancing the model to detect multi-scale objects. Finally, this paper introduces the convolution aggregation crosslayer (CACL) into the network. This module can reduce the size of the feature map and enhance the ability to fuse context information, thereby obtaining a feature map with more semantic information. We performed evaluation experiments on both the SIMD dataset and the UCAS-AOD dataset. Compared to other methods, our approach achieved the highest detection accuracy. Furthermore, it reduced the number of parameters by 12.7% compared to YOLOv7-Tiny. The experimental results illustrated that our proposed method is more lightweight and exhibits superior detection accuracy compared to other lightweight models.
更多查看译文
关键词
large receptive field,remote sensing images,detector,remote sensing,multi-scale,high-precision
AI 理解论文
溯源树
样例
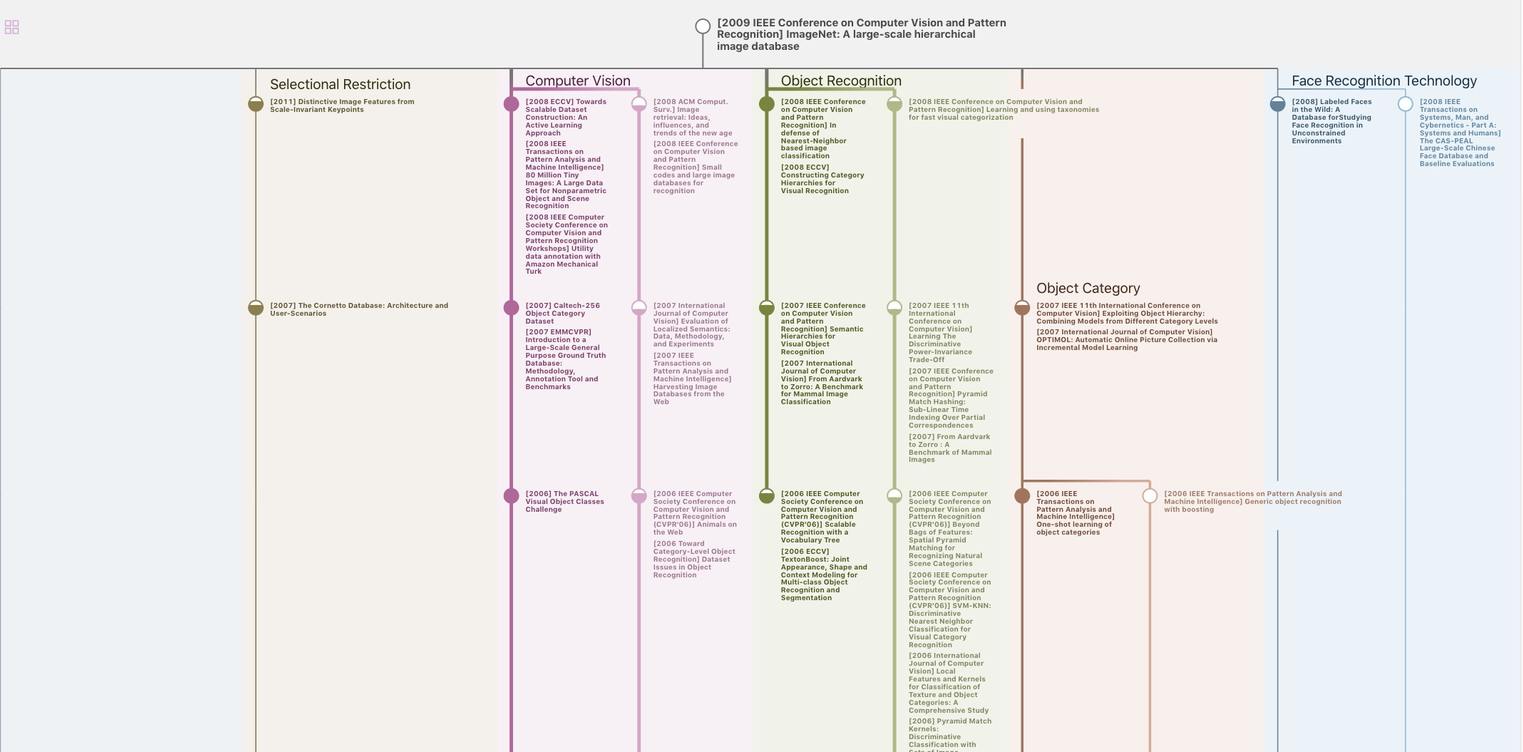
生成溯源树,研究论文发展脉络
Chat Paper
正在生成论文摘要