Exploiting Remote Sensing Imagery for Vehicle Detection and Classification Using an Artificial Intelligence Technique.
Remote sensing(2023)
摘要
Remote sensing imagery involves capturing and examining details about the Earth’s surface from a distance, often using satellites, drones, or other aerial platforms. It offers useful data with which to monitor and understand different phenomena on Earth. Vehicle detection and classification play a crucial role in various applications, including traffic monitoring, urban planning, and environmental analysis. Deep learning, specifically convolutional neural networks (CNNs), has revolutionized vehicle detection in remote sensing. This study designs an improved Chimp optimization algorithm with a DL-based vehicle detection and classification (ICOA-DLVDC) technique on RSI. The presented ICOA-DLVDC technique involves two phases: object detection and classification. For vehicle detection, the ICOA-DLVDC technique applies the EfficientDet model. Next, the detected objects can be classified by using the sparse autoencoder (SAE) model. To optimize the SAE’s hyperparameters effectively, we introduce an ICOA which streamlines the parameter tuning process, accelerating convergence and enhancing the overall performance of the SAE classifier. An extensive set of experiments has been conducted to highlight the improved vehicle classification outcomes of the ICOA-DLVDC technique. The simulation values demonstrated the remarkable performance of the ICOA-DLVDC approach compared to other recent techniques, with a maximum accuracy of 99.70% and 99.50% on the VEDAI dataset and ISPRS Postdam dataset, respectively.
更多查看译文
关键词
artificial intelligence,object detector,computer vision,remote sensing,target detection,deep learning
AI 理解论文
溯源树
样例
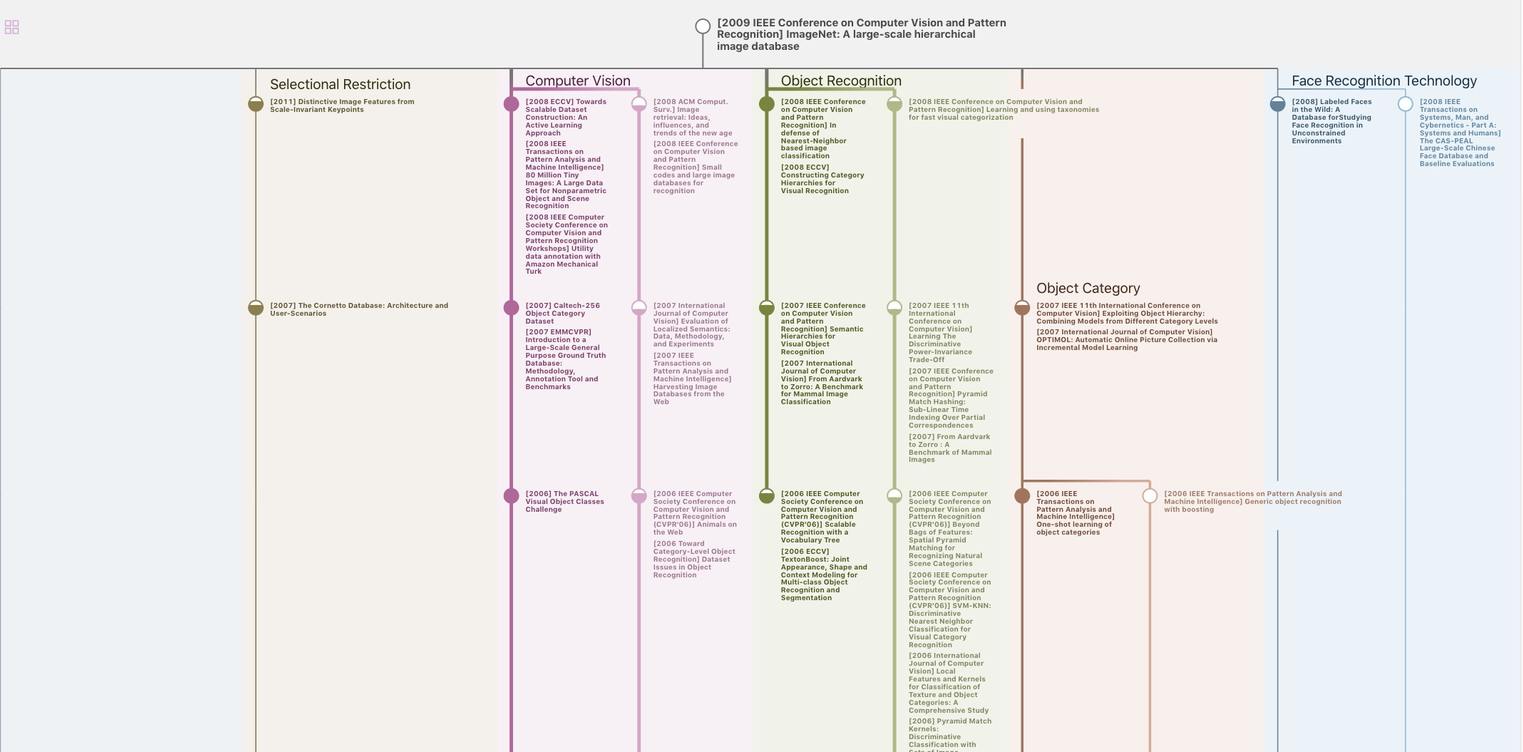
生成溯源树,研究论文发展脉络
Chat Paper
正在生成论文摘要