The Classification of Hyperspectral Images: A Double-Branch Multi-Scale Residual Network
Remote Sensing(2023)
摘要
With the continuous advancement of deep learning technology, researchers have made further progress in the hyperspectral image (HSI) classification domain. We propose a double-branch multi-scale residual network (DBMSRN) framework for HSI classification to improve classification accuracy and reduce the number of required training samples. The DBMSRN consists of two branches designed to extract spectral and spatial features from the HSI. Thus, to obtain more comprehensive feature information, we extracted additional local and global features at different scales by expanding the network width. Moreover, we also increased the network depth to capture deeper feature information. Based on this concept, we devise spectral multi-scale residuals and spatial multi-scale residuals within a double-branch architecture. Additionally, skip connections are employed to augment the context information of the network. We demonstrate that the proposed framework effectively enhances classification accuracy in scenarios with limited training samples through experimental analysis. The proposed framework achieves an overall accuracy of 98.67%, 98.09%, and 96.76% on the Pavia University (PU), Kennedy Space Center (KSC), and Indian Pines (IP) datasets, respectively, surpassing the classification accuracy of existing advanced frameworks under identical conditions.
更多查看译文
关键词
hyperspectral image classification, spectral multi-scale residuals, spatial multi-scale residuals
AI 理解论文
溯源树
样例
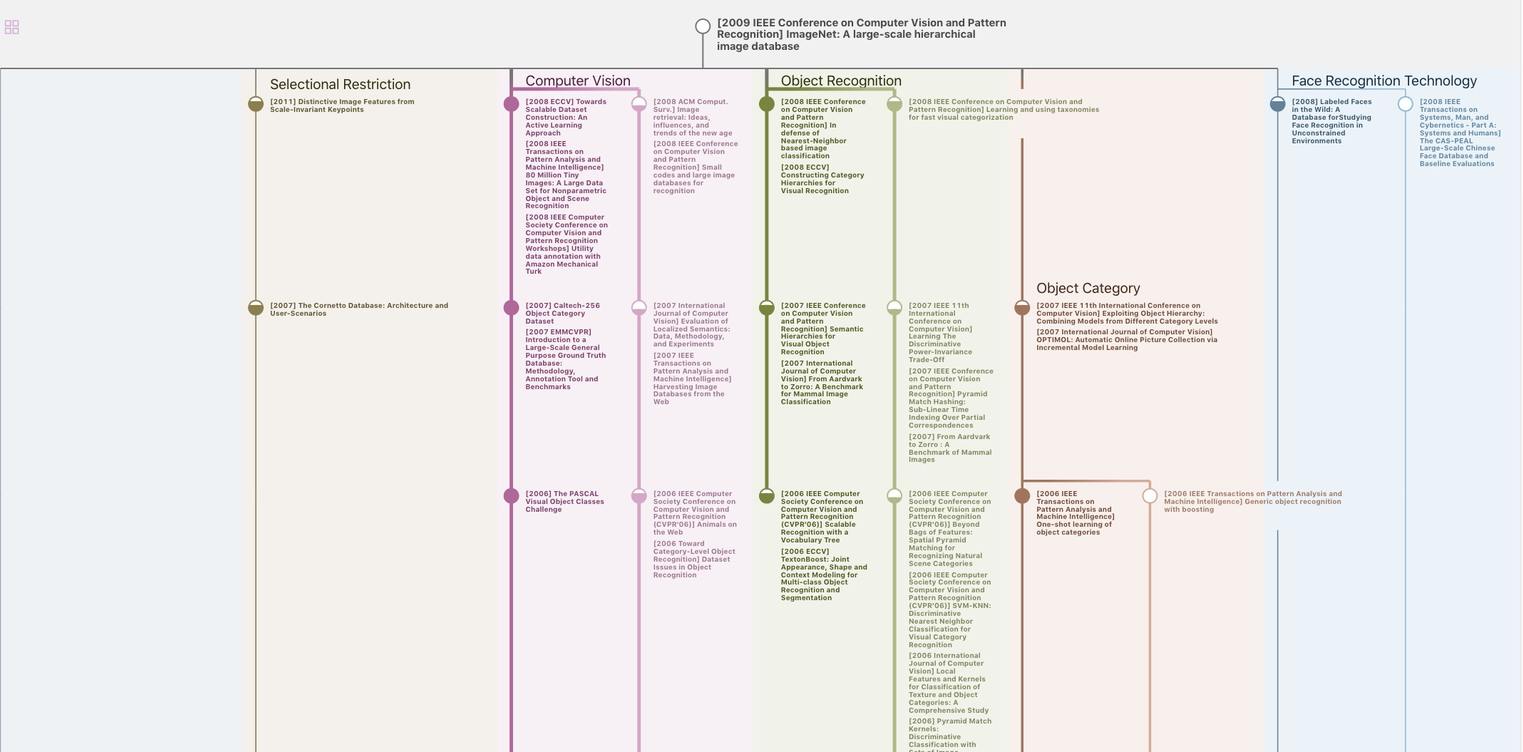
生成溯源树,研究论文发展脉络
Chat Paper
正在生成论文摘要