Mapping Buildings Across Heterogeneous Landscapes: Machine Learning and Deep Learning Applied to Multi-Modal Remote Sensing Data
Remote sensing(2023)
摘要
We describe the production of maps of buildings on Hawai’i Island, based on complementary information contained in two different types of remote sensing data. The maps cover 3200 km2 over a highly varied set of landscape types and building densities. A convolutional neural network was first trained to identify building candidates in LiDAR data. To better differentiate between true buildings and false positives, the CNN-based building probability map was then used, together with 400–2400 nm imaging spectroscopy, as input to a gradient boosting model. Simple vector operations were then employed to further refine the final maps. This stepwise approach resulted in detection of 84%, 100%, and 97% of manually labeled buildings, at the 0.25, 0.5, and 0.75 percentiles of true building size, respectively, with very few false positives. The median absolute error in modeled building areas was 15%. This novel integration of deep learning, machine learning, and multi-modal remote sensing data was thus effective in detecting buildings over large scales and diverse landscapes, with potential applications in urban planning, resource management, and disaster response. The adaptable method presented here expands the range of techniques available for object detection in multi-modal remote sensing data and can be tailored to various kinds of input data, landscape types, and mapping goals.
更多查看译文
关键词
LiDAR,imaging spectroscopy,hyperspectral imaging,remote sensing,deep learning,convolutional neural networks,gradient boosting,image segmentation,building detection,rural residential development
AI 理解论文
溯源树
样例
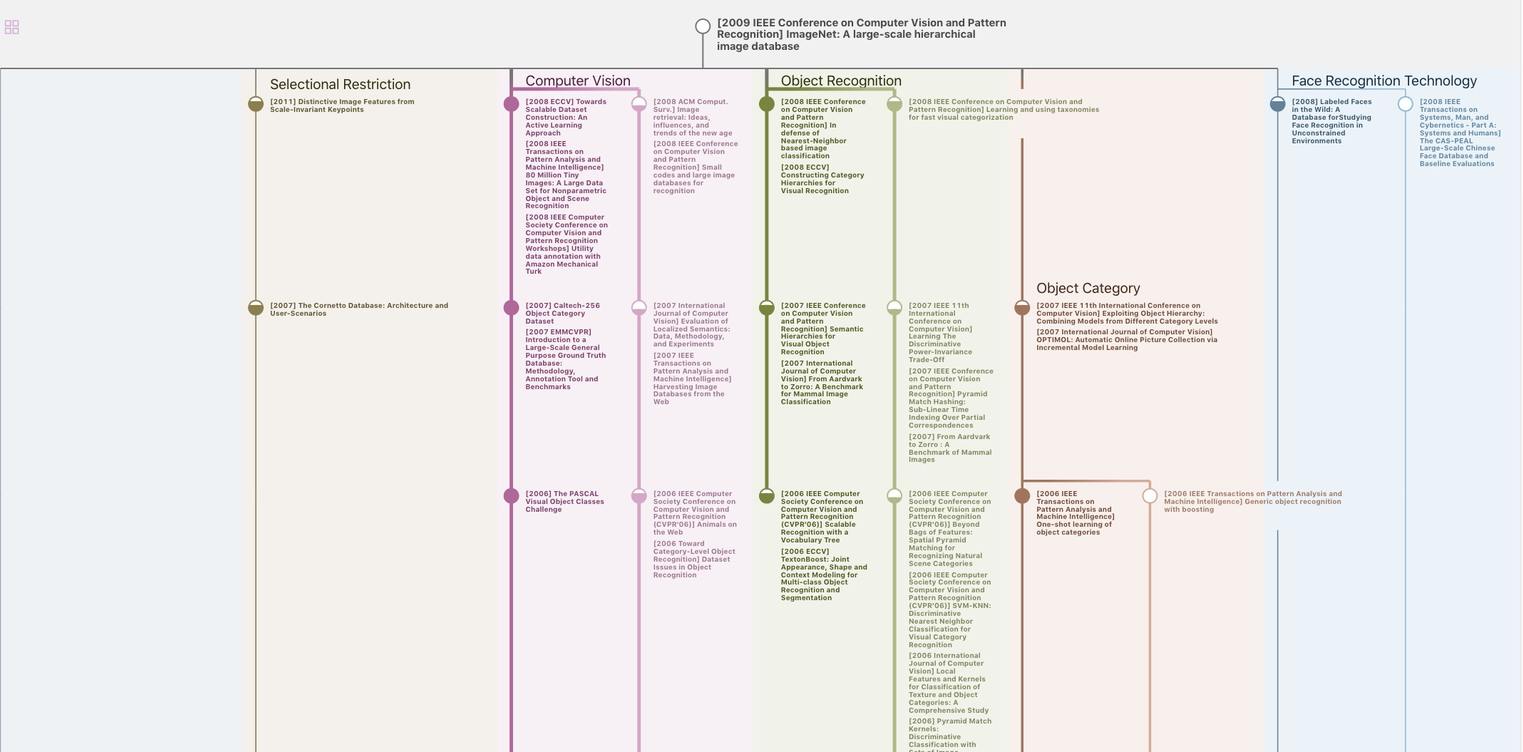
生成溯源树,研究论文发展脉络
Chat Paper
正在生成论文摘要