Advancing Fault Prediction: A Comparative Study between LSTM and Spiking Neural Networks
Processes(2023)
摘要
Predicting system faults is critical to improving productivity, reducing costs, and enforcing safety in industrial processes. Yet, traditional methodologies frequently falter due to the intricate nature of the task. This research presents a novel use of spiking neural networks (SNNs) in anticipating faults in syntactical time series, utilizing the generalized stochastic Petri net (GSPN) model. The inherent ability of SNNs to process both time and space aspects of data positions them as a prime instrument for this endeavor. A comparative evaluation with long short-term memory (LSTM) networks suggests that SNNs offer comparable robustness and performance.
更多查看译文
关键词
spiking neural networks (SNNs),system fault prediction,generalized stochastic Petri net (GSPN),industrial processes,LSTM networks
AI 理解论文
溯源树
样例
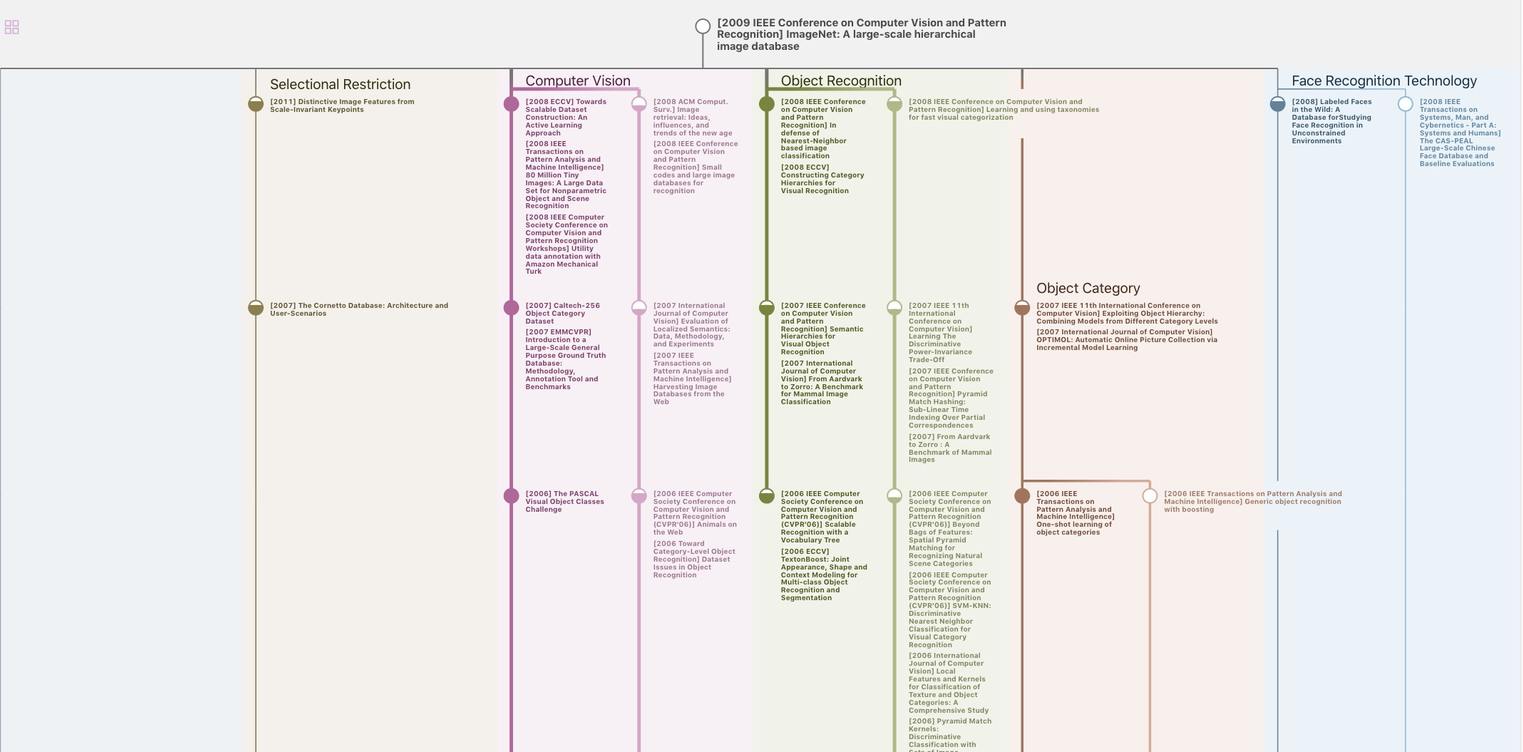
生成溯源树,研究论文发展脉络
Chat Paper
正在生成论文摘要