Unveiling the Power of ARIMA, Support Vector and Random Forest Regressors for the Future of the Dutch Employment Market
Journal of Theoretical and Applied Electronic Commerce Research(2023)
摘要
The increasing popularity of online job vacancies and machine learning methods has raised questions about their combination to enhance our understanding of labour markets and algorithms. However, the lack of comparable studies necessitates further investigation. This research aims to explore the effectiveness of Random Forest Regressor (RFR) and Support Vector Regressor (SVR) machine learning models in predicting online job vacancies compared to the auto-regressive ARIMA method. To answer this question, detailed sub-questions are posed in relation to the sub-samples of the main data provided by Birch Consultants, an external partner originally obtained by Jobdigger. Drawing upon previous research on time-series accuracy, this study combines various approaches to benefit society and the external partner. Using the walk-forward validation method, with a 91-day expanding window, it provides precise answers to the sub-questions. Findings suggest that RFR is suitable for forecasting larger samples, while SVR is preferred due to its capability to predict small series despite relatively small scoring benefits and computational costs. Both machine learning models outperform the baseline ARIMA model in capturing complex time-series. Further research should focus on exploring advanced auto-regressive, deep learning, and hybrid models for future investigations.
更多查看译文
关键词
dutch employment market,random forest regressors,arima,support vector
AI 理解论文
溯源树
样例
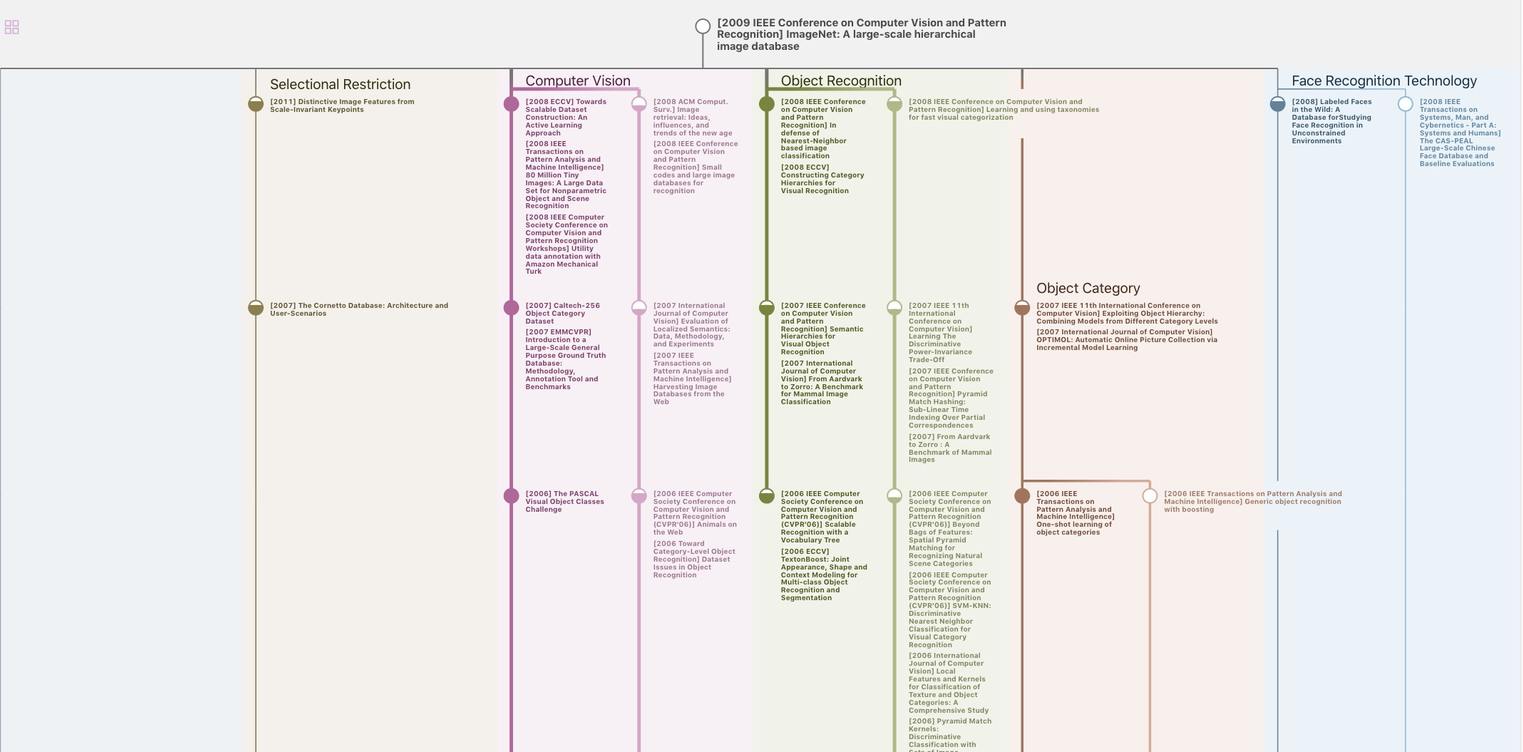
生成溯源树,研究论文发展脉络
Chat Paper
正在生成论文摘要