SNM Radiation Signature Classification Using Different Semi-Supervised Machine Learning Models
Journal of nuclear engineering(2023)
摘要
The timely detection of special nuclear material (SNM) transfers between nuclear facilities is an important monitoring objective in nuclear nonproliferation. Persistent monitoring enabled by successful detection and characterization of radiological material movements could greatly enhance the nuclear nonproliferation mission in a range of applications. Supervised machine learning can be used to signal detections when material is present if a model is trained on sufficient volumes of labeled measurements. However, the nuclear monitoring data needed to train robust machine learning models can be costly to label since radiation spectra may require strict scrutiny for characterization. Therefore, this work investigates the application of semi-supervised learning to utilize both labeled and unlabeled data. As a demonstration experiment, radiation measurements from sodium iodide (NaI) detectors are provided by the Multi-Informatics for Nuclear Operating Scenarios (MINOS) venture at Oak Ridge National Laboratory (ORNL) as sample data. Anomalous measurements are identified using a method of statistical hypothesis testing. After background estimation, an energy-dependent spectroscopic analysis is used to characterize an anomaly based on its radiation signatures. In the absence of ground-truth information, a labeling heuristic provides data necessary for training and testing machine learning models. Supervised logistic regression serves as a baseline to compare three semi-supervised machine learning models: co-training, label propagation, and a convolutional neural network (CNN). In each case, the semi-supervised models outperform logistic regression, suggesting that unlabeled data can be valuable when training and demonstrating value in semi-supervised nonproliferation implementations.
更多查看译文
关键词
nuclear nonproliferation,gamma-ray spectroscopy,radiation monitoring,data analysis,semi-supervised machine learning
AI 理解论文
溯源树
样例
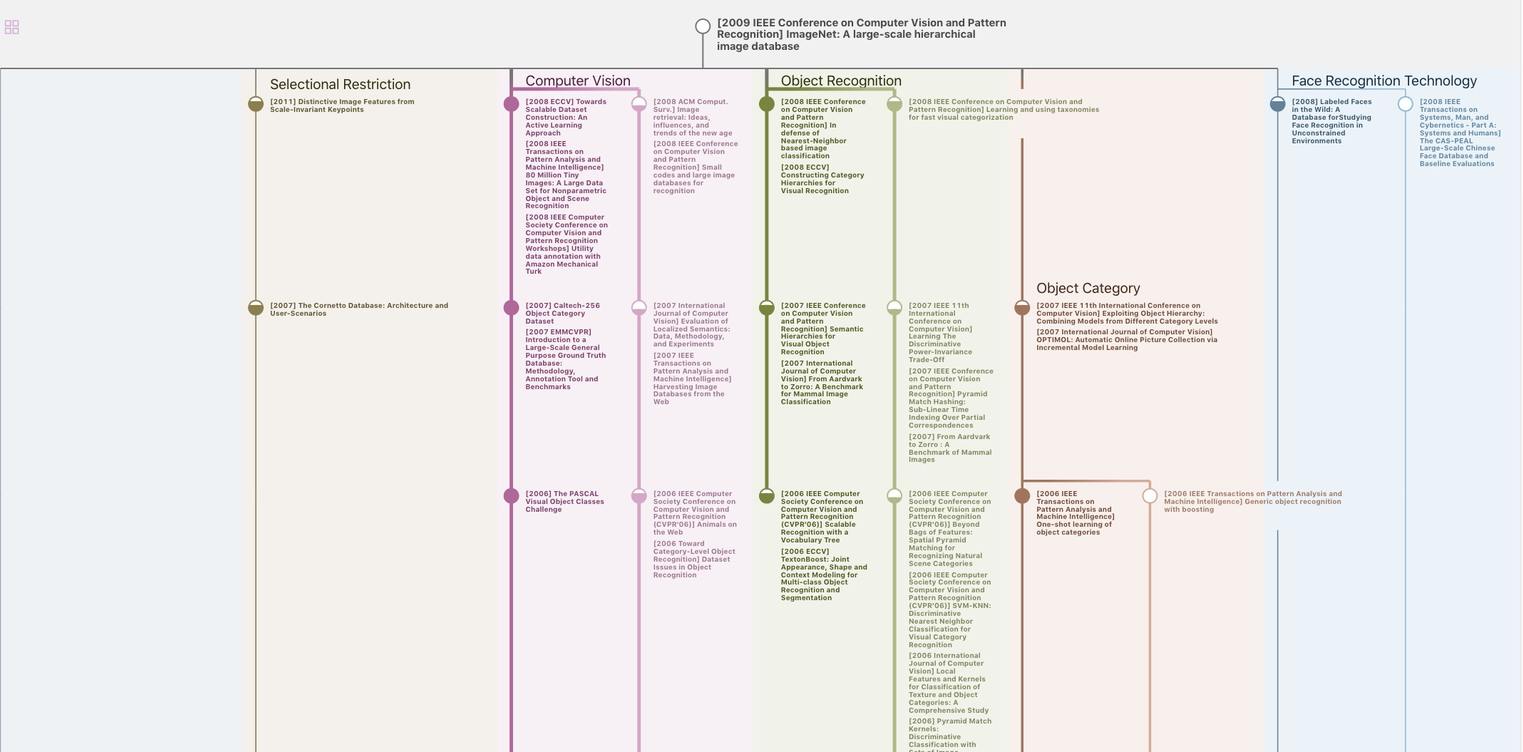
生成溯源树,研究论文发展脉络
Chat Paper
正在生成论文摘要