3D-EddyNet: A Novel Approach for Identifying Three-Dimensional Morphological Features of Mesoscale Eddies in the Ocean
Journal of Marine Science and Engineering(2023)
摘要
Mesoscale eddies are characterized by swirling currents spanning from tens to hundreds of kilometers in diameter three-dimensional attributes holds paramount significance in driving advancements in both oceanographic research and engineering applications. Nonetheless, a notable absence of models capable of adeptly harnessing the scarcity of high-quality annotated marine data, to efficiently discern the three-dimensional morphological attributes of mesoscale eddies, is evident. To address this limitation, this paper constructs an innovative deep-learning-based model termed 3D-EddyNet, tailored for the precise identification and visualization of mesoscale eddies. In contrast to the prevailing 2D models that remain confined to surface-level data, 3D-EddyNet takes full advantage of three-dimensional convolutions to capture the essential characteristics of eddies. It is specifically tailored for recognizing spatial features within mesoscale eddies, including parameters like position, radius, and depth. The combination of dynamic convolutions and residual networks effectively enhances the model’s performance in a synergistic manner. The model employs the PReLU activation function to tackle gradient vanishing issues and improve convergence rates. It also addresses the challenge of foreground–background imbalance through cross-entropy functions. Additionally, to fine-tune the model’s effectiveness during the training phase, techniques such as random dropblock and batch normalization are skillfully incorporated. Furthermore, we created a training dataset using HYCOM data specifically from the South China Sea region. This dataset allowed for a comprehensive analysis of the spatial-temporal distribution and three-dimensional morphology of the eddies, serving as an assessment of the model’s practical effectiveness. The culmination of this analysis reveals an impressive 20% enhancement over 3D-UNet in detection accuracy, coupled with expedited convergence speed. Notably, the results obtained through our detection using empirical data align closely with those obtained by other scholars. The mesoscale eddies within this specific region unveil a discernible northeast-to-southwest distribution pattern, categorized into three principal morphological classifications: bowl-shaped, olive-shaped, and nearly cylindrical, with the bowl-shaped eddies prominently dominating.
更多查看译文
关键词
mesoscale eddies,ocean,d-eddynet,three-dimensional
AI 理解论文
溯源树
样例
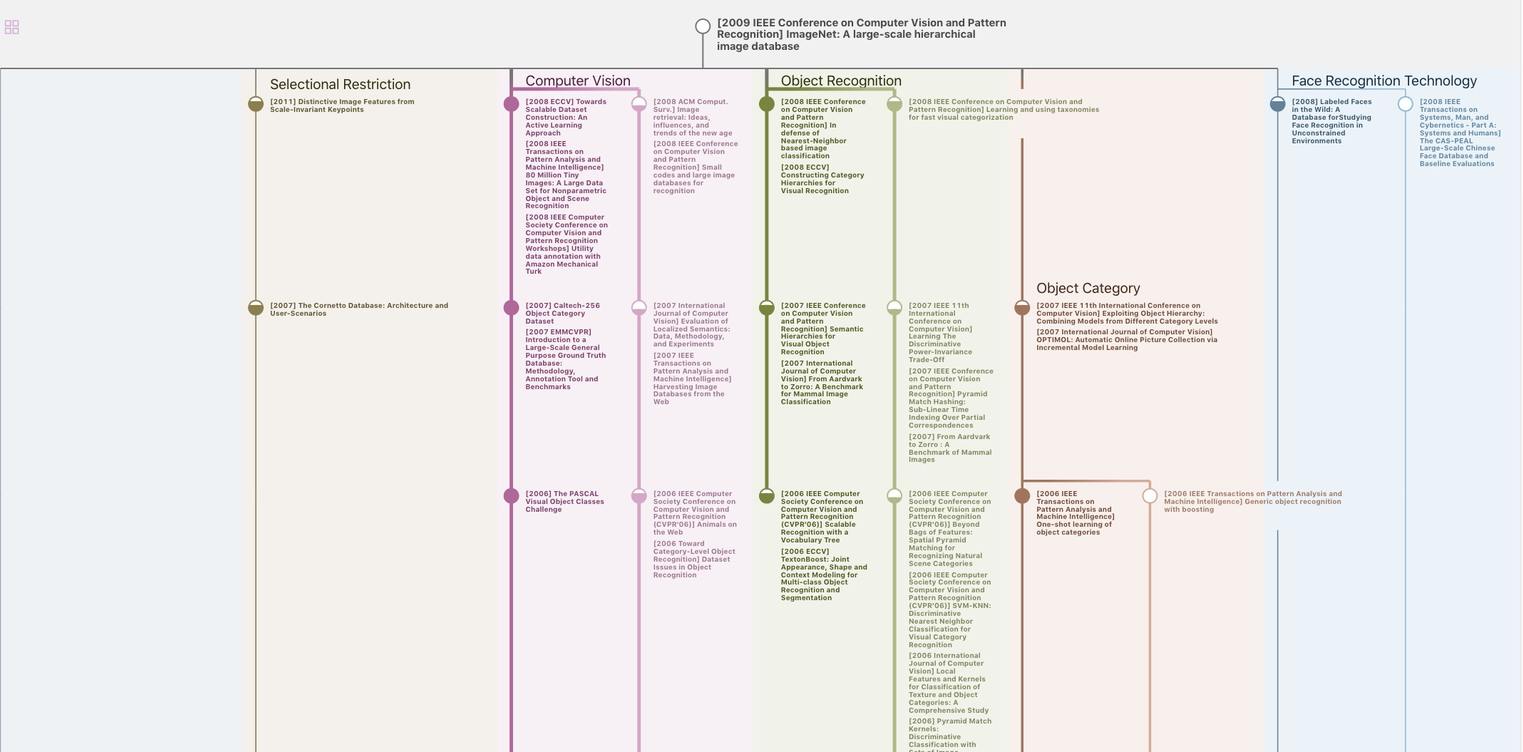
生成溯源树,研究论文发展脉络
Chat Paper
正在生成论文摘要