A User-Driven Sampling Model for Large-Scale Geographical Point Data Visualization via Convolutional Neural Networks
IEEE Transactions on Human-Machine Systems(2023)
摘要
Numerous sampling strategies have been proposed to reduce the visual clutter of large-scale geographical point data visualization, which focus on the preservation of original data features, such as randomness, spatial distribution, and associated relationship. However, user preferences and demands are not taken into account in the course of sampling, which will lead to the sampled results deviating from user requirements and impede personalized geospatial analysis in specific application scenarios. In this article, we propose a user-driven sampling model for the visual abstraction of the large-scale geographical point data based on convolutional neural networks (CNN). First, a blue noise sampling model is applied to partition the geographical space into local areas, and a set of visual interfaces are designed to present the data features of those points in the local areas, enabling users to visually select representative points according to their requirements. Then, user preferences are quantified with a CNN model based on the eigenvectors of the representative points, which are further utilized to guide the sampling courses of the other local areas. Thus, all the sampled points will retain the spatial distribution of original data points and fulfill the user preference as far as possible. In addition, we implement a visualization framework to integrate manual point selection, CNN training, automatic point sampling, and visual comparison, allowing users to easily obtain and evaluate the sampled points from the perspectives of data analysis and user requirements. Quantitative comparisons and case studies based on real-world datasets are conducted to demonstrate the effectiveness of our sampling model in the preservation of user preferences and visual exploration of large-scale geospatial point data.
更多查看译文
关键词
visualization,sampling model,convolutional neural networks,user-driven,large-scale
AI 理解论文
溯源树
样例
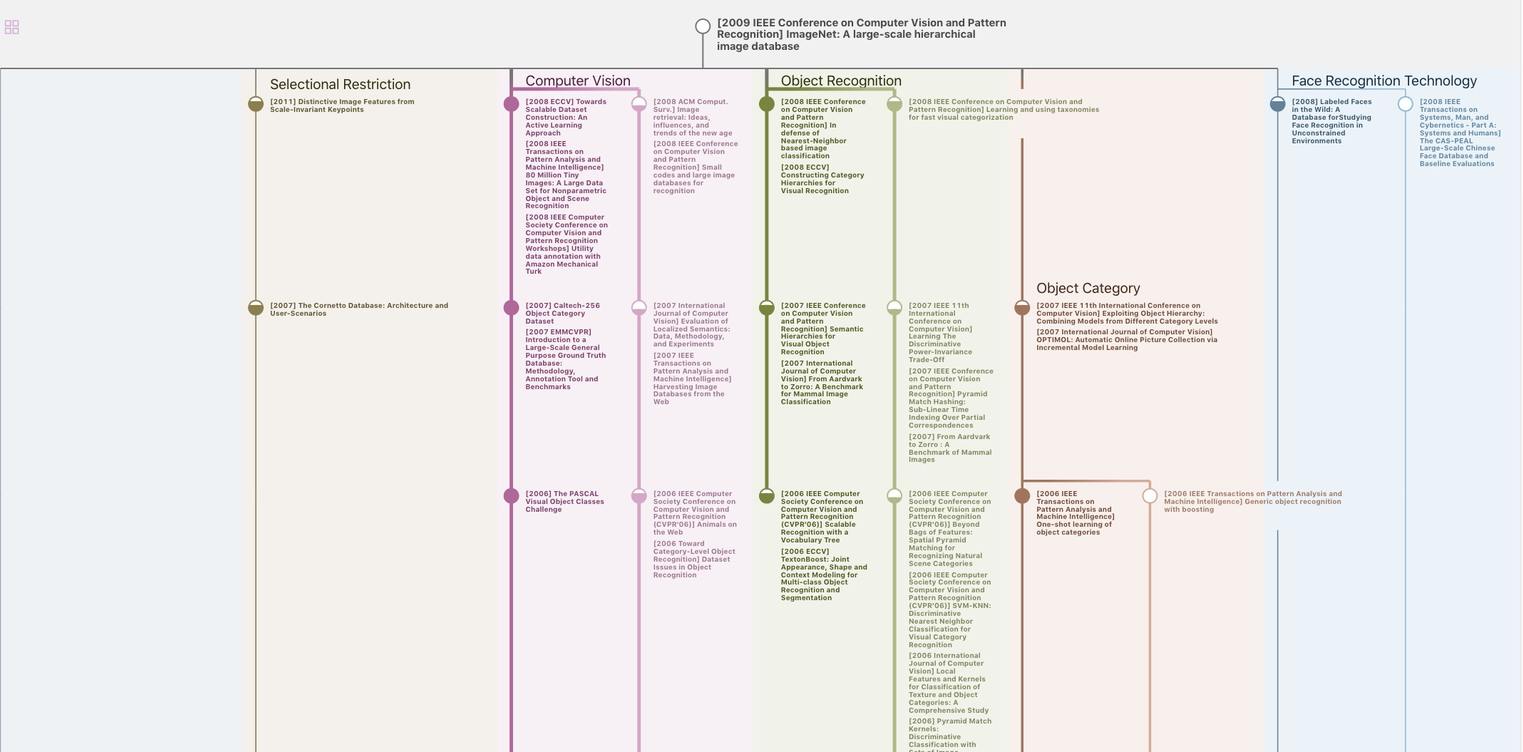
生成溯源树,研究论文发展脉络
Chat Paper
正在生成论文摘要