Towards Foundation Models Learned from Anatomy in Medical Imaging via Self-supervision
DOMAIN ADAPTATION AND REPRESENTATION TRANSFER, DART 2023(2024)
摘要
Human anatomy is the foundation of medical imaging and boasts one striking characteristic: its hierarchy in nature, exhibiting two intrinsic properties: (1) locality: each anatomical structure is morphologically distinct from the others; and (2) compositionality: each anatomical structure is an integrated part of a larger whole. We envision a foundation model for medical imaging that is consciously and purposefully developed upon this foundation to gain the capability of "understanding" human anatomy and to possess the fundamental properties of medical imaging. As our first step in realizing this vision towards foundation models in medical imaging, we devise a novel self-supervised learning (SSL) strategy that exploits the hierarchical nature of human anatomy. Our extensive experiments demonstrate that the SSL pretrained model, derived from our training strategy, not only outperforms state-of-the-art (SOTA) fully/self-supervised baselines but also enhances annotation efficiency, offering potential few-shot segmentation capabilities with performance improvements ranging from 9% to 30% for segmentation tasks compared to SSL baselines. This performance is attributed to the significance of anatomy comprehension via our learning strategy, which encapsulates the intrinsic attributes of anatomical structures-locality and compositionality-within the embedding space, yet overlooked in existing SSL methods. All code and pretrained models are available at GitHub.com/JLiangLab/Eden.
更多查看译文
关键词
Self-supervised Learning,Learning from Anatomy
AI 理解论文
溯源树
样例
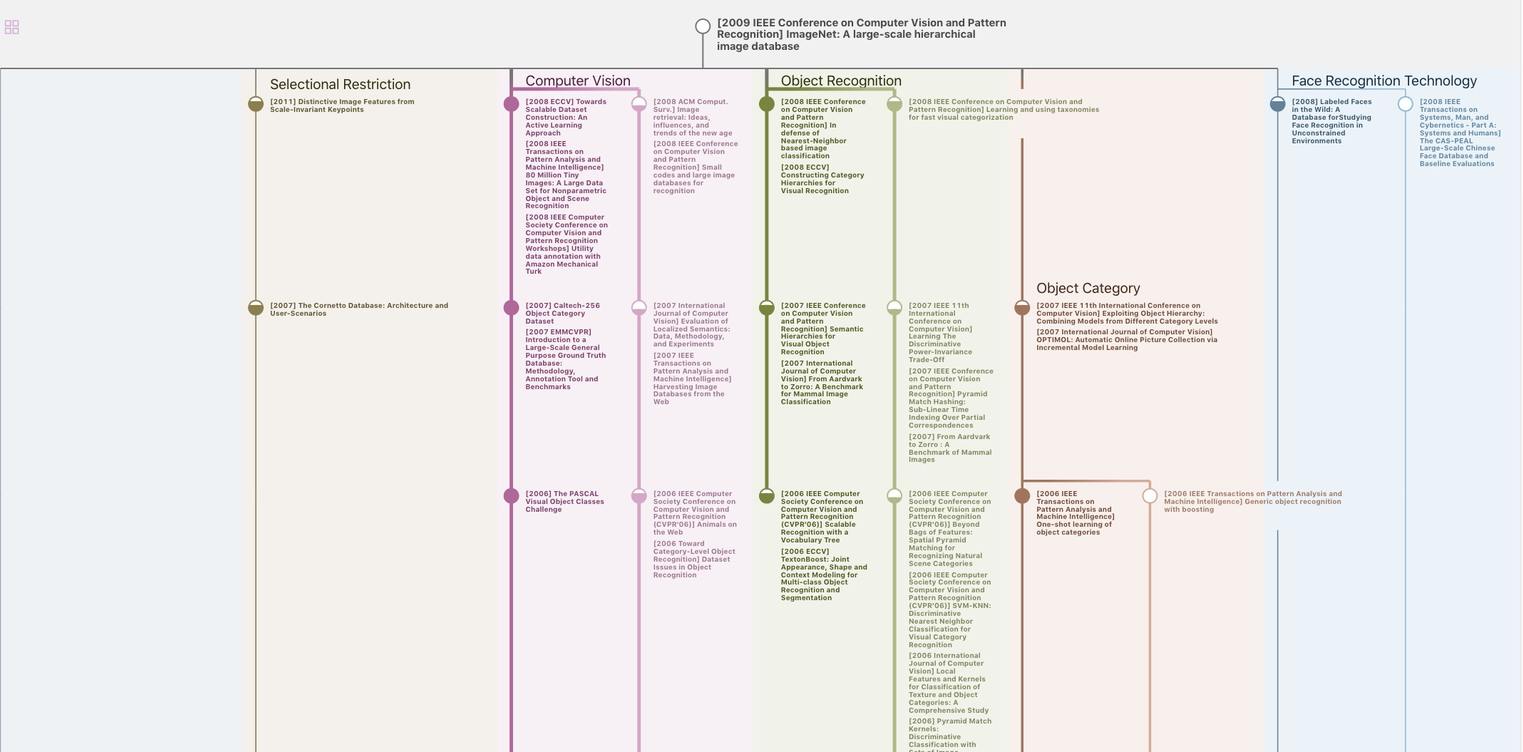
生成溯源树,研究论文发展脉络
Chat Paper
正在生成论文摘要