Evaluation of Constrained Reinforcement Learning Algorithms for Legged Locomotion
CoRR(2023)
摘要
Shifting from traditional control strategies to Deep Reinforcement Learning (RL) for legged robots poses inherent challenges, especially when addressing real-world physical constraints during training. While high-fidelity simulations provide significant benefits, they often bypass these essential physical limitations. In this paper, we experiment with the Constrained Markov Decision Process (CMDP) framework instead of the conventional unconstrained RL for robotic applications. We perform a comparative study of different constrained policy optimization algorithms to identify suitable methods for practical implementation. Our robot experiments demonstrate the critical role of incorporating physical constraints, yielding successful sim-to-real transfers, and reducing operational errors on physical systems. The CMDP formulation streamlines the training process by separately handling constraints from rewards. Our findings underscore the potential of constrained RL for the effective development and deployment of learned controllers in robotics.
更多查看译文
关键词
constrained reinforcement learning algorithms,locomotion,reinforcement learning,legged
AI 理解论文
溯源树
样例
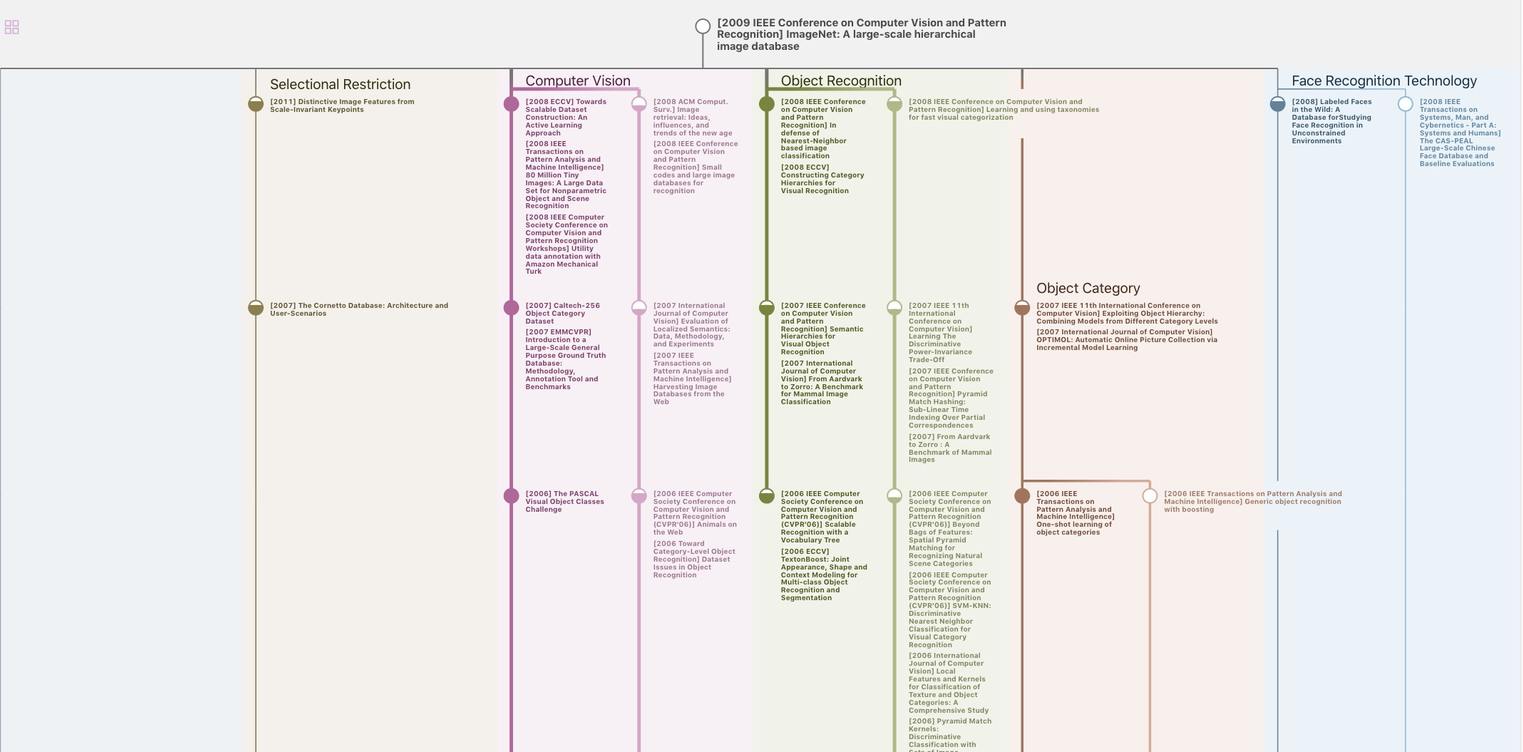
生成溯源树,研究论文发展脉络
Chat Paper
正在生成论文摘要