Data-Driven Framework for Uncovering Hidden Control Strategies in Evolutionary Analysis
MATHEMATICAL AND COMPUTATIONAL APPLICATIONS(2023)
摘要
We devised a data-driven framework for uncovering hidden control strategies used by an evolutionary system described by an evolutionary probability distribution. This innovative framework enables deciphering of the concealed mechanisms that contribute to the progression or mitigation of such situations as the spread of COVID-19. Novel algorithms are used to estimate the optimal control in tandem with the parameters for evolution in general dynamical systems, thereby extending the concept of model predictive control. This marks a significant departure from conventional control methods, which require knowledge of the system to manipulate its evolution and of the controller's strategy or parameters. We use a generalized additive model, supplemented by extensive statistical testing, to identify a set of predictor covariates closely linked to the control. Using real-world COVID-19 data, we delineate the descriptive behaviors of the COVID-19 epidemics in five prefectures in Japan and nine countries. We compare these nine countries and group them on the basis of shared profiles, providing valuable insights into their pandemic responses. Our findings underscore the potential of our framework as a powerful tool for understanding and managing complex evolutionary processes.
更多查看译文
关键词
data-driven optimization algorithm,model predictive control,evolutionary probability distribution,generalized additive model,classification,COVID-19 evolution
AI 理解论文
溯源树
样例
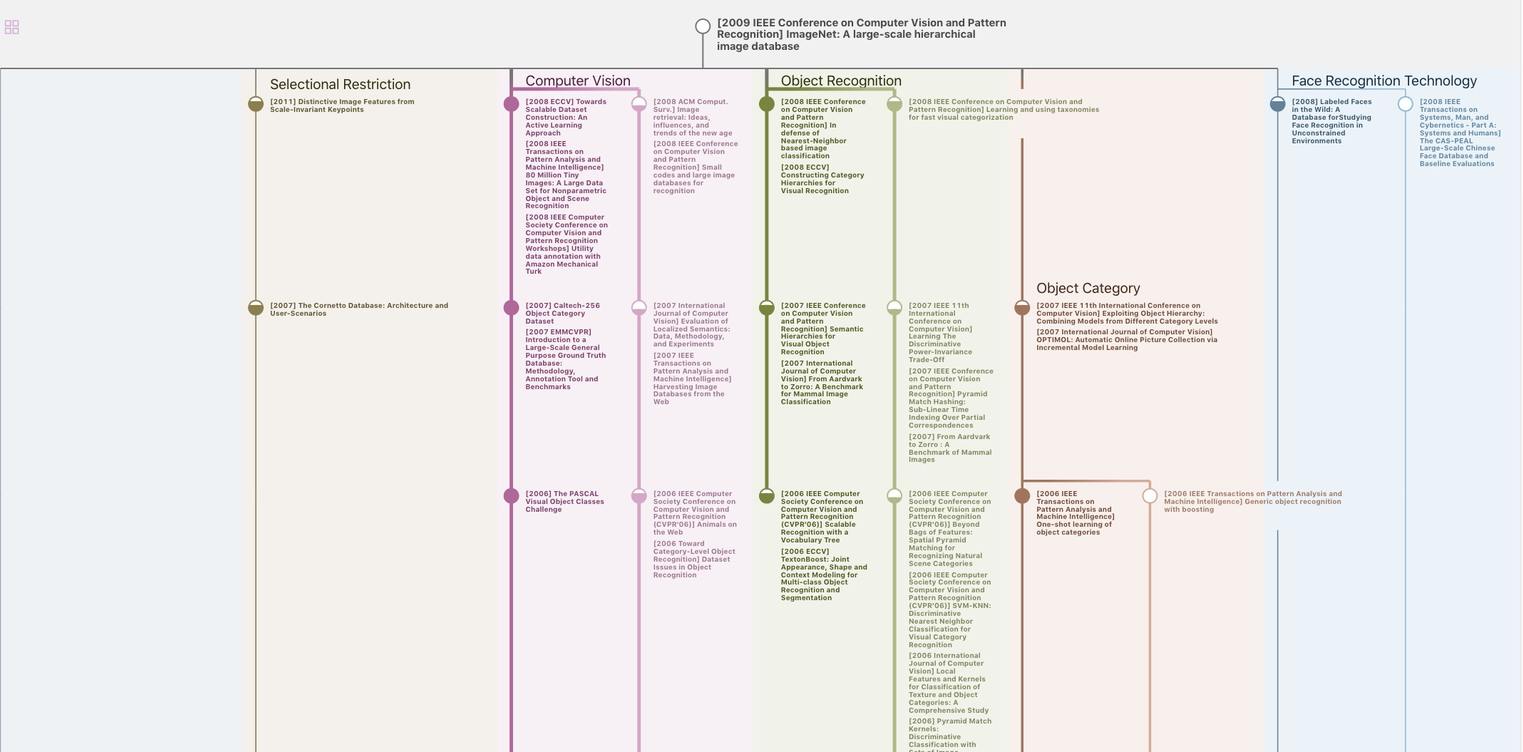
生成溯源树,研究论文发展脉络
Chat Paper
正在生成论文摘要