The CGAS Deep Learning Algorithm for P-Wave Arrival Time Picking of Mining Microseismic Events
IEEE Access(2023)
摘要
In light of the inadequacies of traditional P-wave arrival picking algorithms using long and short windows, which exhibit poor anti-noise ability and do not meet the requirements for efficient and accurate P-wave arrival picking, this study proposes a CGAS deep learning algorithm for P-wave arrival picking in mine micro-seismic events. The algorithm constructs the depth characteristics of microseismic waveform through deep learning training and converts the problem of picking up the microseismic waveform into a classification problem by employing data set segmentation and classification. This allows the algorithm to pick up the characteristic information of microseismic waveform and improve the accuracy of P-wave picking. The algorithm was applied to pick up the three-component micro-seismic waveforms obtained from the microseismic monitoring system in a mining area in Liaoning from 2019 to 2020. Through ablation experiments, it was found that adding each structure to the pickup model improved the relevant indexes to some extent. When all network structures were used, the accuracy of the P-wave pick-up model reached 98.61%, ensuring the accuracy of P-wave pickup upon arrival and demonstrating the effectiveness of each layer structure in the classification of P-wave arrival. Compared to other P-wave arrival picking algorithms such as STA/LTA, U-Net++, Dpick, PhaseNet and EQTransformer, the algorithm proposed in this paper exhibited high precision in P-wave arrival time picking, providing a new research idea and technical means for efficiently determining the P-wave arrival of events.
更多查看译文
关键词
deep learning,p-wave
AI 理解论文
溯源树
样例
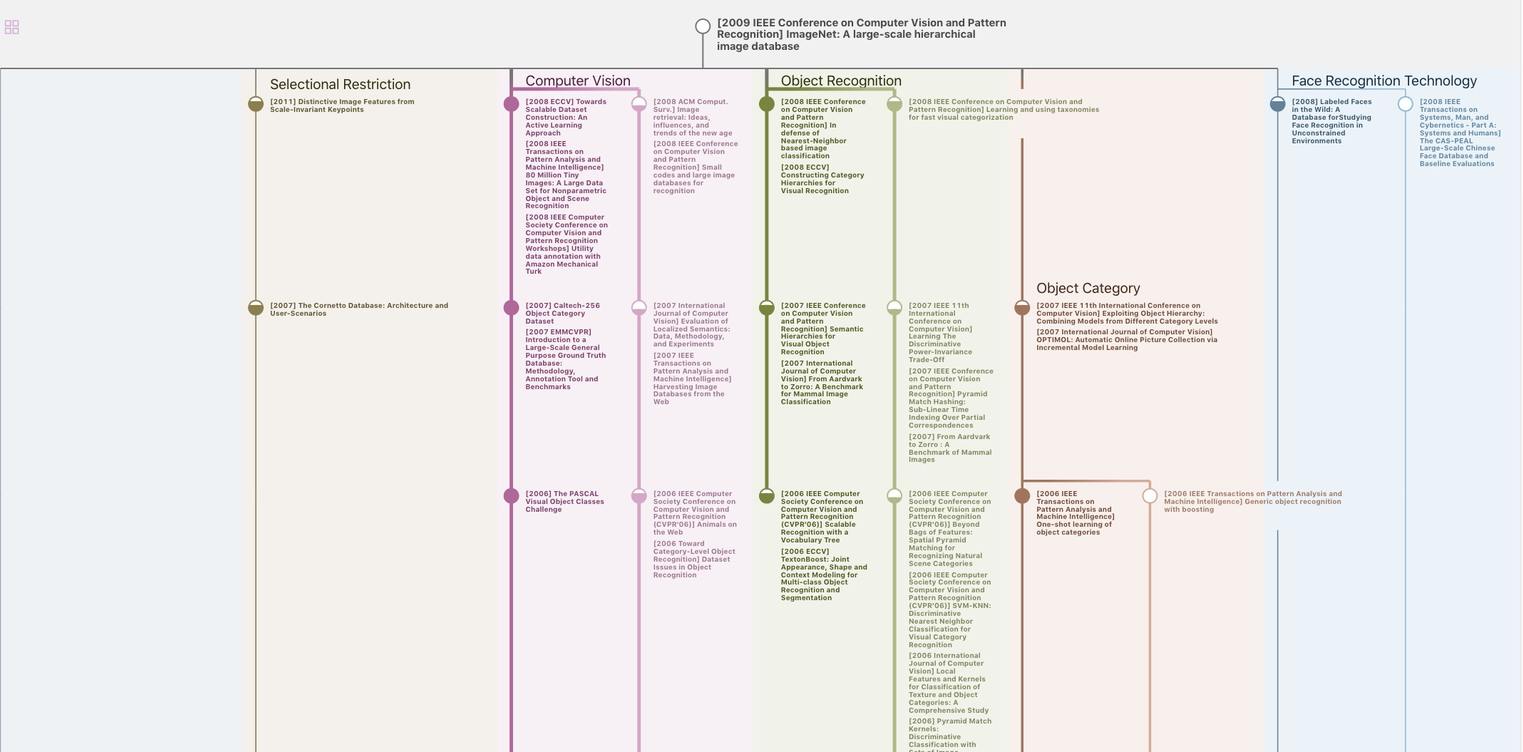
生成溯源树,研究论文发展脉络
Chat Paper
正在生成论文摘要