Reconstructing Microstructures From Statistical Descriptors Using Neural Cellular Automata
Integrating Materials and Manufacturing Innovation(2024)
摘要
The problem of generating microstructures of complex materials in silico has been approached from various directions including simulation, Markov, deep learning and descriptor-based approaches. This work presents a hybrid method that is inspired by all four categories and has interesting scalability properties. A neural cellular automaton is trained to evolve microstructures based on local information. Unlike most machine learning-based approaches, it does not directly require a data set of reference micrographs, but is trained from statistical microstructure descriptors that can stem from a single reference. This means that the training cost scales only with the complexity of the structure and associated descriptors. Since the size of the reconstructed structures can be set during inference, even extremely large structures can be efficiently generated. Similarly, the method is very efficient if many structures are to be reconstructed from the same descriptor for statistical evaluations. The method is formulated and discussed in detail by means of various numerical experiments, demonstrating its utility and scalability.
更多查看译文
关键词
Microstructure,Reconstruction,Descriptor,Neural cellular automata
AI 理解论文
溯源树
样例
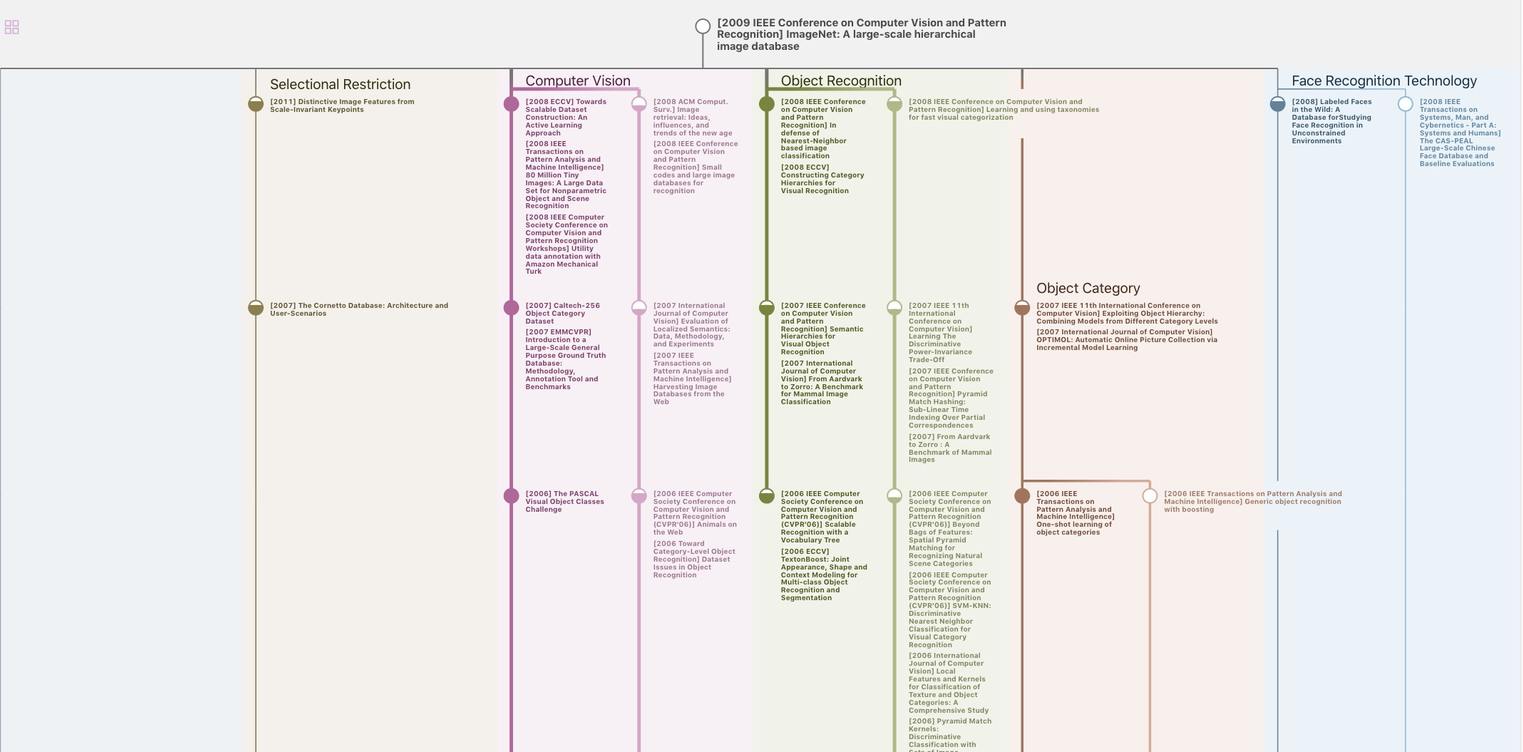
生成溯源树,研究论文发展脉络
Chat Paper
正在生成论文摘要