Exposing image splicing traces in scientific publications via uncertainty-guided refinement
Patterns(2024)
摘要
Recently, a surge in image manipulations in scientific publications has led to numerous retractions, highlighting the importance of image integrity. Although forensic detectors for image duplication and synthesis have been researched, the detection of image splicing in scientific publications remains largely unexplored. Splicing detection is more challenging than duplication detection due to the lack of reference images and more difficult than synthesis detection because of the presence of smaller tampered-with areas. Moreover, disruptive factors in scientific images, such as artifacts, abnormal patterns, and noise, present misleading features like splicing traces, rendering this task difficult. In addition, the scarcity of high-quality datasets of spliced scientific images has limited advancements. Therefore, we propose the uncertainty-guided refinement network (URN) to mitigate these disruptive factors. We also construct a dataset for image splicing detection (SciSp) with 1,290 spliced images by collecting and manually splicing. Comprehensive experiments demonstrate the URN’s superior splicing detection performance.
更多查看译文
关键词
scientific integrity,image splicing detection,convolution neural network,and uncertainty
AI 理解论文
溯源树
样例
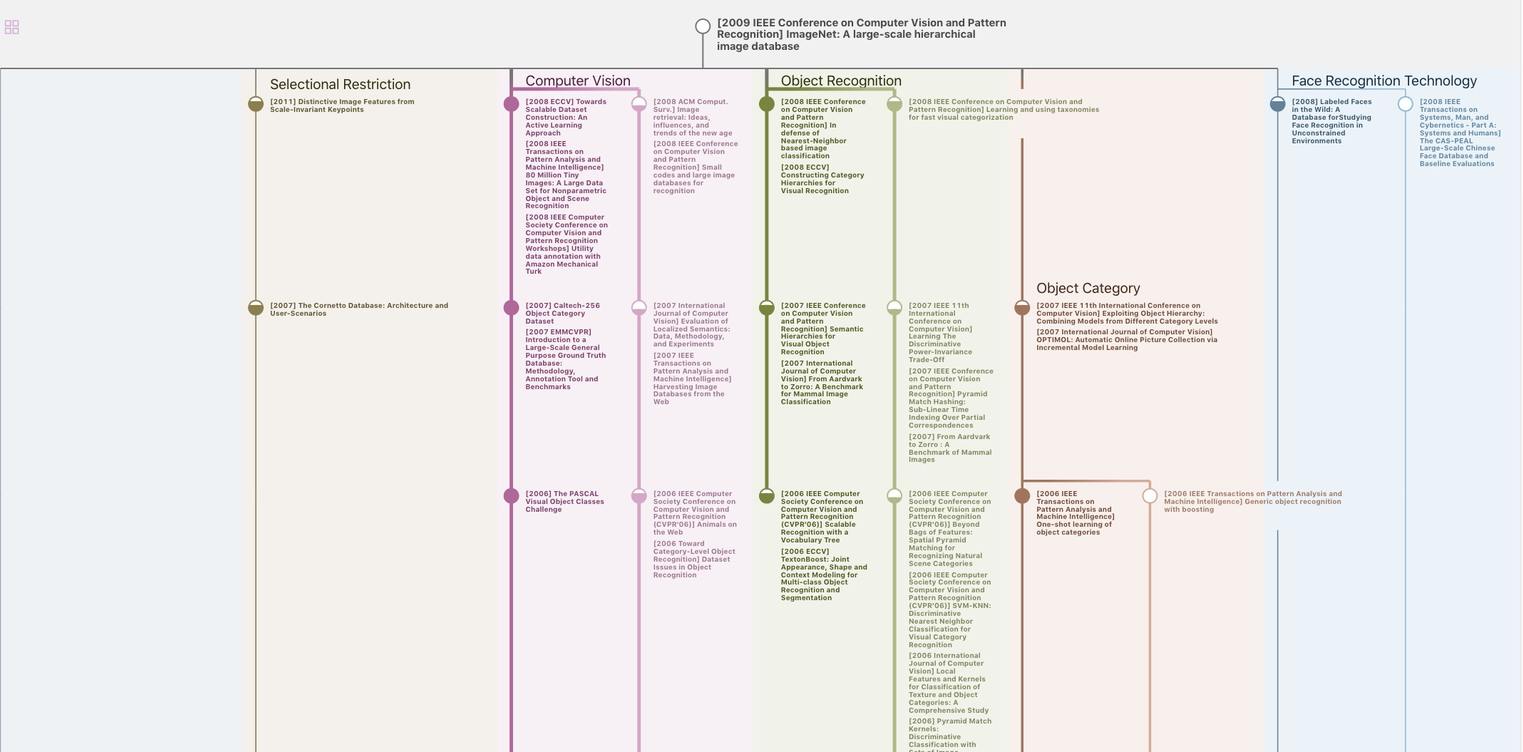
生成溯源树,研究论文发展脉络
Chat Paper
正在生成论文摘要