Structural Risk Minimization for Learning Nonlinear Dynamics
CoRR(2023)
摘要
Recent advances in learning or identification of nonlinear dynamics focus on learning a suitable model within a pre-specified model class. However, a key difficulty that remains is the choice of the model class from which the dynamics will be learned. The fundamental challenge is trading the richness of the model class with the learnability within the model class. Toward addressing the so-called model selection problem, we introduce a novel notion of Structural Risk Minimization (SRM) for learning nonlinear dynamics. Inspired by classical SRM for classification, we minimize a bound on the true prediction error over hierarchies of model classes. The class selected by our SRM scheme is shown to achieve a nearly optimal learning guarantee among all model classes contained in the hierarchy. Employing the proposed scheme along with computable model class complexity bounds, we derive explicit SRM schemes for learning nonlinear dynamics under hierarchies of: i) norm-constrained Reproducing Kernel Hilbert Spaces, and ii) norm-constrained Neural Network classes. We empirically show that even though too loose to be used as absolute estimates, our SRM bounds on the true prediction error are able to track its relative behavior across different model classes of the hierarchy.
更多查看译文
关键词
structural risk minimization,dynamics,learning
AI 理解论文
溯源树
样例
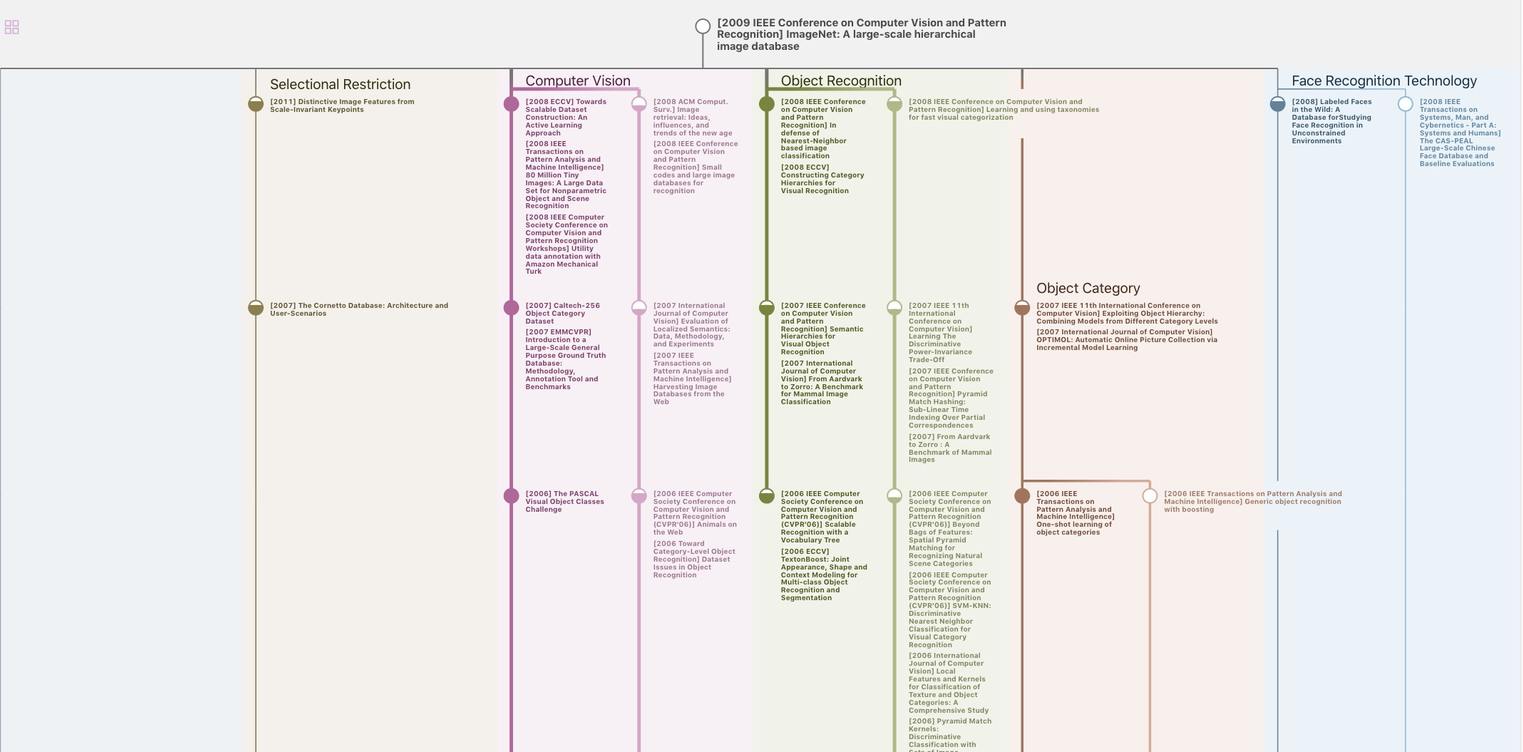
生成溯源树,研究论文发展脉络
Chat Paper
正在生成论文摘要