A Deterministic Almost-Linear Time Algorithm for Minimum-Cost Flow
2023 IEEE 64TH ANNUAL SYMPOSIUM ON FOUNDATIONS OF COMPUTER SCIENCE, FOCS(2023)
摘要
We give a deterministic m(1+o(1)) time algorithm that computes exact maximum flows and minimum-cost flows on directed graphs with m edges and polynomially bounded integral demands, costs, and capacities. As a consequence, we obtain the first running time improvement for deterministic algorithms that compute maximum-flow in graphs with polynomial bounded capacities since the work of Goldberg-Rao [J.ACM '98]. Our algorithm builds on the framework of Chen-Kyng-Liu-Peng-Gutenberg-Sachdeva [FOCS '22] that computes an optimal flow by computing a sequence of m(1+o(1))-approximate undirected minimum-ratio cycles. We develop a deterministic dynamic graph data-structure to compute such a sequence of minimum-ratio cycles in an amortized m(o(1)) time per edge update. Our key technical contributions are deterministic analogues of the vertex sparsification and edge sparsification components of the data-structure from Chen et al. For the vertex sparsification component, we give a method to avoid the randomness in Chen et al. which involved sampling random trees to recurse on. For the edge sparsification component, we design a deterministic algorithm that maintains an embedding of a dynamic graph into a sparse spanner. We also show how our dynamic spanner can be applied to give a deterministic data structure that maintains a fully dynamic low-stretch spanning tree on graphs with polynomially bounded edge lengths, with subpolynomial average stretch and subpolynomial amortized time per edge update.
更多查看译文
关键词
Maximum flow,Minimum cost flow,Data structures,Interior point methods,Convex optimization,Derandomization
AI 理解论文
溯源树
样例
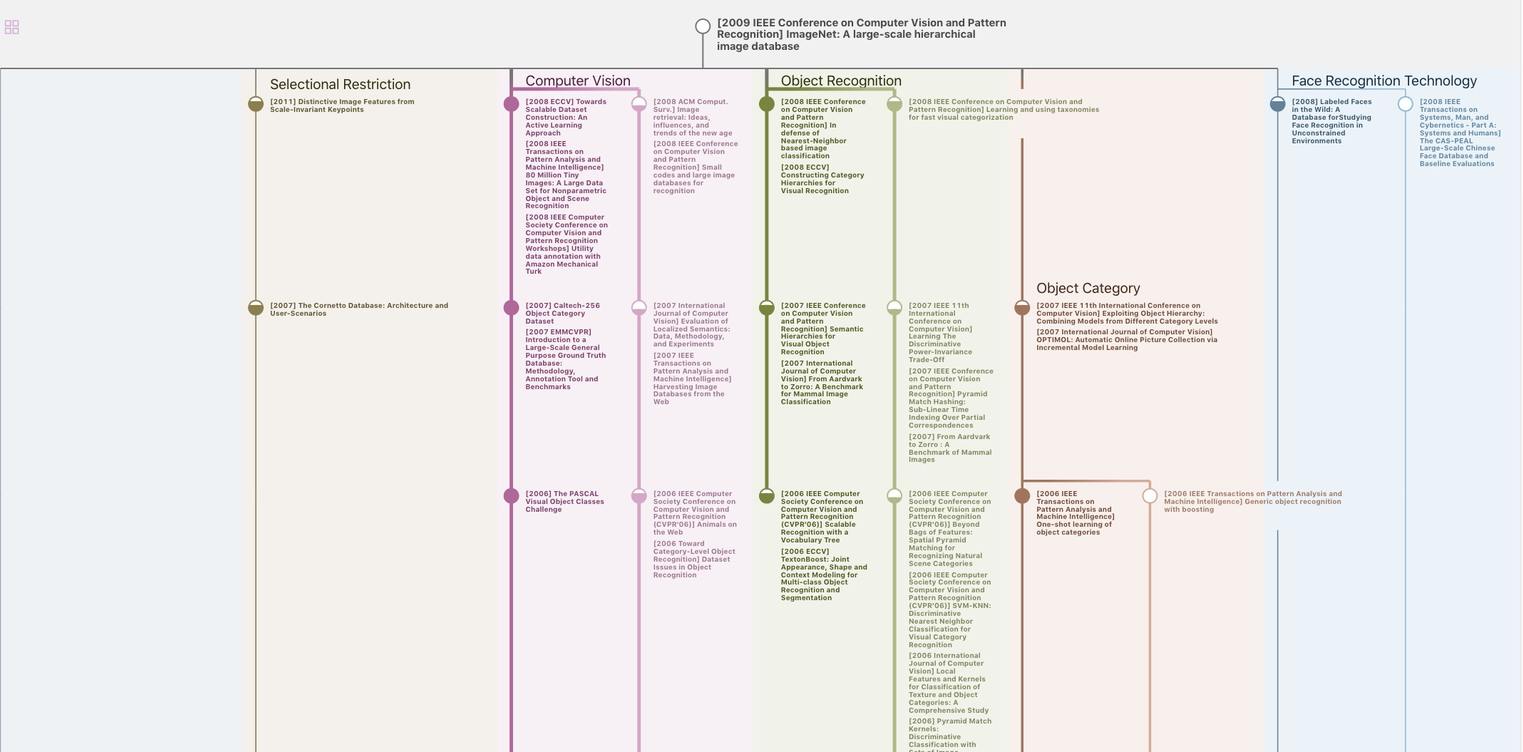
生成溯源树,研究论文发展脉络
Chat Paper
正在生成论文摘要