Deep Learning Reconstruction Improves the Image Quality of CT Angiography Derived From 80-kVp Cerebral CT Perfusion Data
Academic radiology(2023)
摘要
Rationale and Objective: To investigate the impact of the deep learning reconstruction (DLR) technique on the image quality of CT angiography (CTA) derived from 80-kVp cerebral CT perfusion (CTP) data and compare it with hybrid-iterative reconstruction (HIR).Materials and methods: Thirty-three patients underwent CTP at 80 kVp were prospectively enrolled. CTP data were reconstructed with HIR and DLR. Four image datasets were reconstructed: HIRpeak and DLRpeak were single arterial phase images derived from the time point showing the peak value, HIRtMIP and HIRtAve were time-resolved maximum intensity projection image and time-resolved average image derived from three time points with the greatest enhancement of HIR. The mean CT values, standard deviation, signal-to-noise ratio, and contrast-to-noise ratio of the internal carotid artery and basilar artery were compared among the four image dataset. Image quality was performed using a five-point rating scale. Arterial stenosis was evaluated.Results: DLRpeak had the highest CT value and contrast-to-noise ratio in the internal carotid artery and basilar artery (all p < 0.001). DLRpeak showed the best subjective image quality and had the highest score (4.93 +/- 0.4) compared to the other three HIR CTA images (all p < 0.001). The degree of vascular stenosis was consistent among the four evaluated sequences (HIRtAve, HIRpeak, and HIRtMIP DLRpeak).Conclusion: For CTA derived from 80-kVp cerebral CTP data, images reconstructed with deep learning showed better image quality and improved intracranial artery visualization than those processed with HIR and other currently used techniques.(c) 2023 Published by Elsevier Inc. on behalf of The Association of University Radiologists.
更多查看译文
关键词
reconstruction angiography,image quality,deep learning
AI 理解论文
溯源树
样例
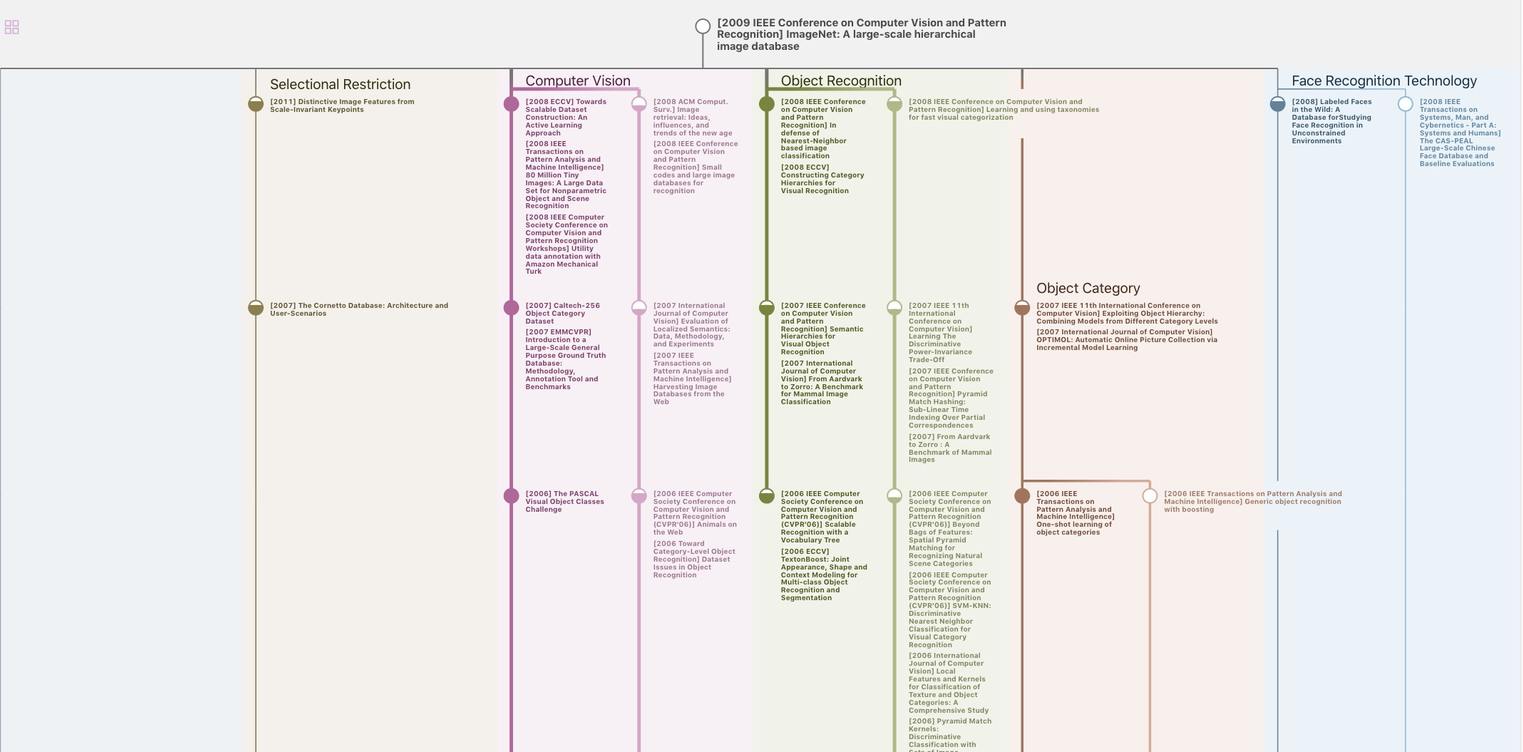
生成溯源树,研究论文发展脉络
Chat Paper
正在生成论文摘要