Requirements Classification for Smart Allocation: A Case Study in the Railway Industry
2023 IEEE 31st International Requirements Engineering Conference (RE)(2023)
摘要
Allocation of requirements to different teams is a typical preliminary task in large-scale system development projects. This critical activity is often performed manually and can benefit from automated requirements classification techniques. To date, limited evidence is available about the effectiveness of existing machine learning (ML) approaches for requirements classification in industrial cases. This paper aims to fill this gap by evaluating state-of-the-art language models and ML algorithms for classification in the railway industry. Since the interpretation of the results of ML systems is particularly relevant in the studied context, we also provide an information augmentation approach to complement the output of the ML-based classification. Our results show that the BERT uncased language model with the softmax classifier can allocate the requirements to different teams with a 76% F1 score when considering requirements allocation to the most frequent teams. Information augmentation provides potentially useful indications in 76% of the cases. The results confirm that currently available techniques can be applied to real-world cases, thus enabling the first step for technology transfer of automated requirements classification. The study can be useful to practitioners operating in requirements-centered contexts such as railways, where accurate requirements classification becomes crucial for better allocation of requirements to various teams.
更多查看译文
关键词
requirements classification,requirements allocation,natural language processing,language models
AI 理解论文
溯源树
样例
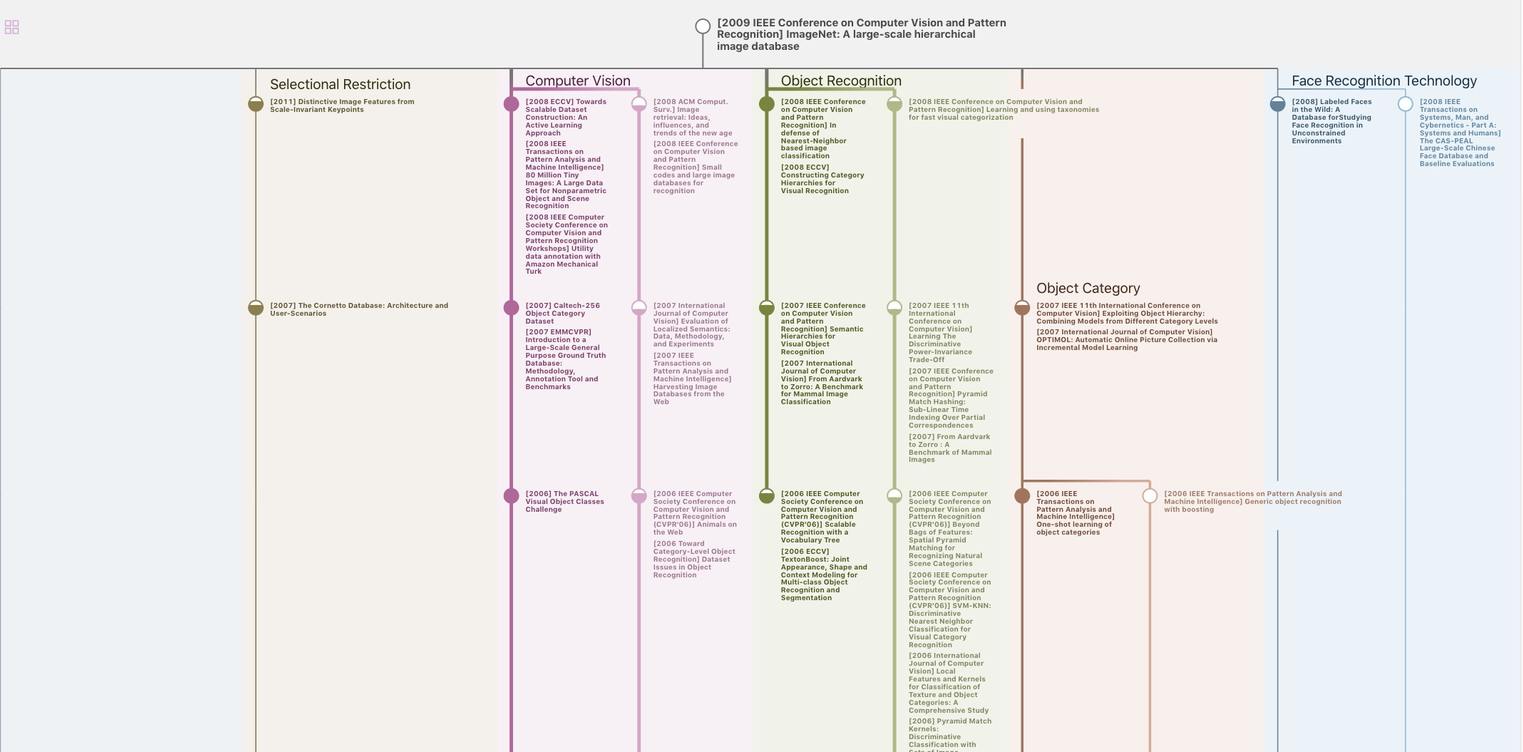
生成溯源树,研究论文发展脉络
Chat Paper
正在生成论文摘要