Dense Neural Network for Calculating Solvation Free Energies from Electronegativity-Equalization Atomic Charges.
Journal of chemical information and modeling(2023)
摘要
I propose a dense Neural Network for evaluation of solvation free energies Δ for molecules and ions in water and nonaqueous solvents, Easy Solvation Energy with Electronegativity Equalization charges and Dense Neural Network (ESE-EE-DNN). As input features, it uses the Conductor-like Screening Model (COSMO) electrostatic energy, atomic cavity surface areas, total cavity volume, and induced surface charges. For the COSMO calculation, electronegativity-equalization atomic charges are employed. ESE-EE-DNN exhibits fairly high accuracy, similar or even superior to that of mainstream density functional theory-based methods. For neutral solutes in water, polar protic, polar aprotic, and nonpolar solvents, ESE-EE-DNN yields a root-mean-square error (RMSE) of 1.25, 1.36, 0.70, and 0.71 kcal/mol, respectively. ESE-EE-DNN is particularly advantageous for ionic solutes, with an RMSE of 2.82 and 1.42 kcal/mol for aqueous and nonaqueous ion solutions, correspondingly. ESE-EE-DNN is very efficient due to a fast evaluation of the electronegativity-equalization charges.
更多查看译文
关键词
solvation free energies,dense neural network,neural network,electronegativity-equalization
AI 理解论文
溯源树
样例
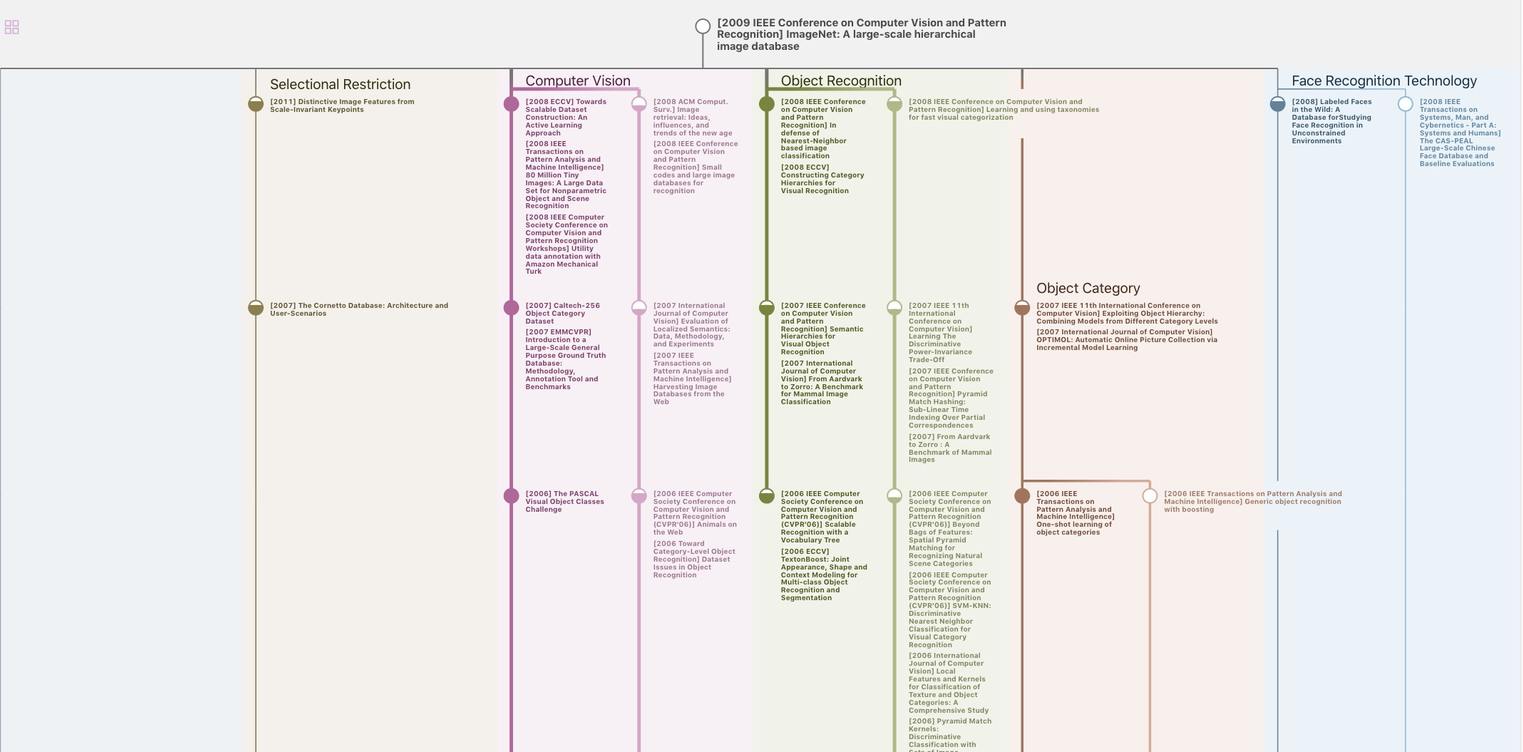
生成溯源树,研究论文发展脉络
Chat Paper
正在生成论文摘要