General Lipschitz: Certified Robustness Against Resolvable Semantic Transformations via Transformation-Dependent Randomized Smoothing
CoRR(2023)
摘要
Randomized smoothing is the state-of-the-art approach to construct image classifiers that are provably robust against additive adversarial perturbations of bounded magnitude. However, it is more complicated to construct reasonable certificates against semantic transformation (e.g., image blurring, translation, gamma correction) and their compositions. In this work, we propose \emph{General Lipschitz (GL),} a new framework to certify neural networks against composable resolvable semantic perturbations. Within the framework, we analyze transformation-dependent Lipschitz-continuity of smoothed classifiers w.r.t. transformation parameters and derive corresponding robustness certificates. Our method performs comparably to state-of-the-art approaches on the ImageNet dataset.
更多查看译文
关键词
resolvable semantic
AI 理解论文
溯源树
样例
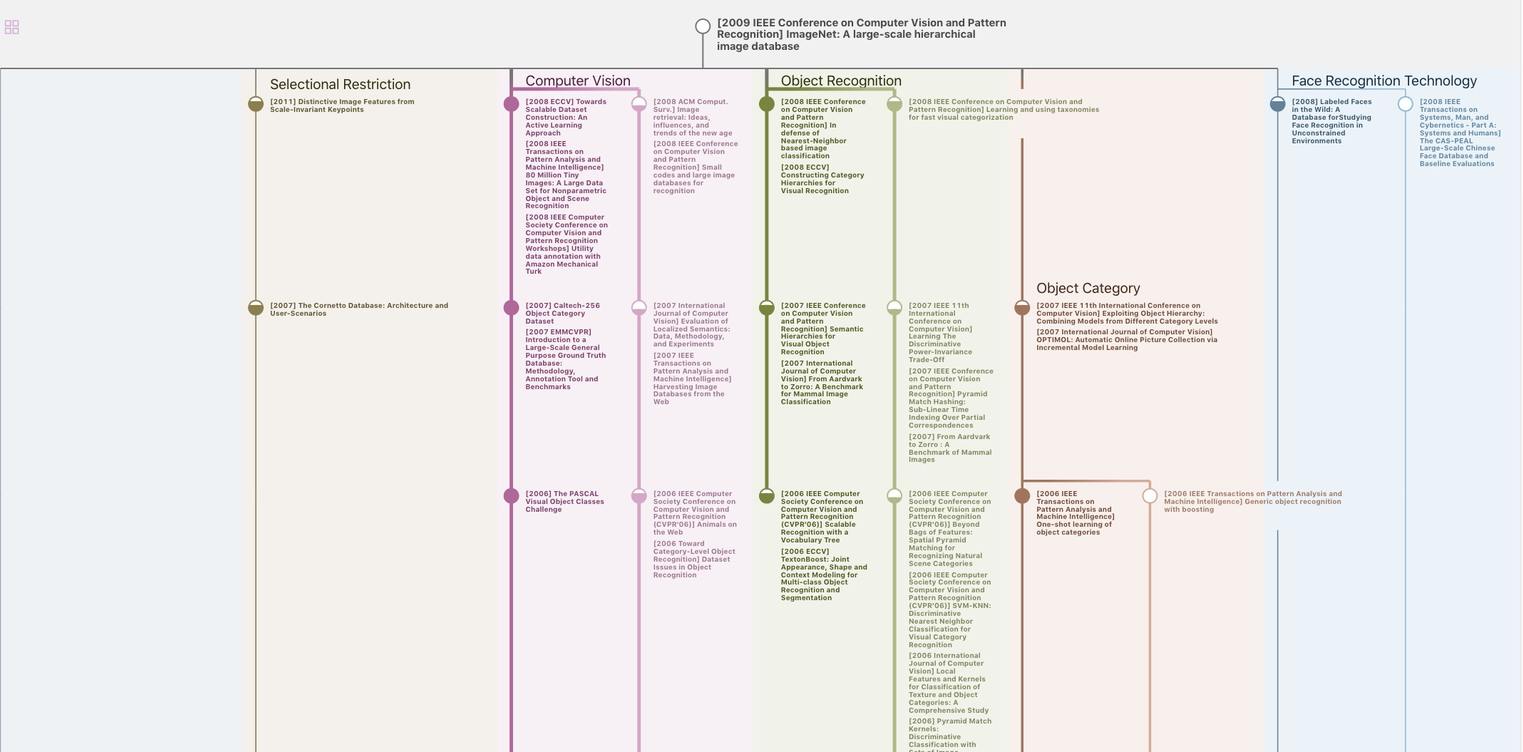
生成溯源树,研究论文发展脉络
Chat Paper
正在生成论文摘要